A Meta-Framework for Modeling the Human Reading Process in Sentiment Analysis.
ACM Trans. Inf. Syst.(2016)
摘要
This article introduces a sentiment analysis approach that adopts the way humans read, interpret, and extract sentiment from text. Our motivation builds on the assumption that human interpretation should lead to the most accurate assessment of sentiment in text. We call this automated process Human Reading for Sentiment (HRS). Previous research in sentiment analysis has produced many frameworks that can fit one or more of the HRS aspects; however, none of these methods has addressed them all in one approach. HRS provides a meta-framework for developing new sentiment analysis methods or improving existing ones. The proposed framework provides a theoretical lens for zooming in and evaluating aspects of any sentiment analysis method to identify gaps for improvements towards matching the human reading process. Key steps in HRS include the automation of humans low-level and high-level cognitive text processing. This methodology paves the way towards the integration of psychology with computational linguistics and machine learning to employ models of pragmatics and discourse analysis for sentiment analysis. HRS is tested with two state-of-the-art methods; one is based on feature engineering, and the other is based on deep learning. HRS highlighted the gaps in both methods and showed improvements for both.
更多查看译文
关键词
Sentiment analysis,human reading,psychology,supervised learning and notions
AI 理解论文
溯源树
样例
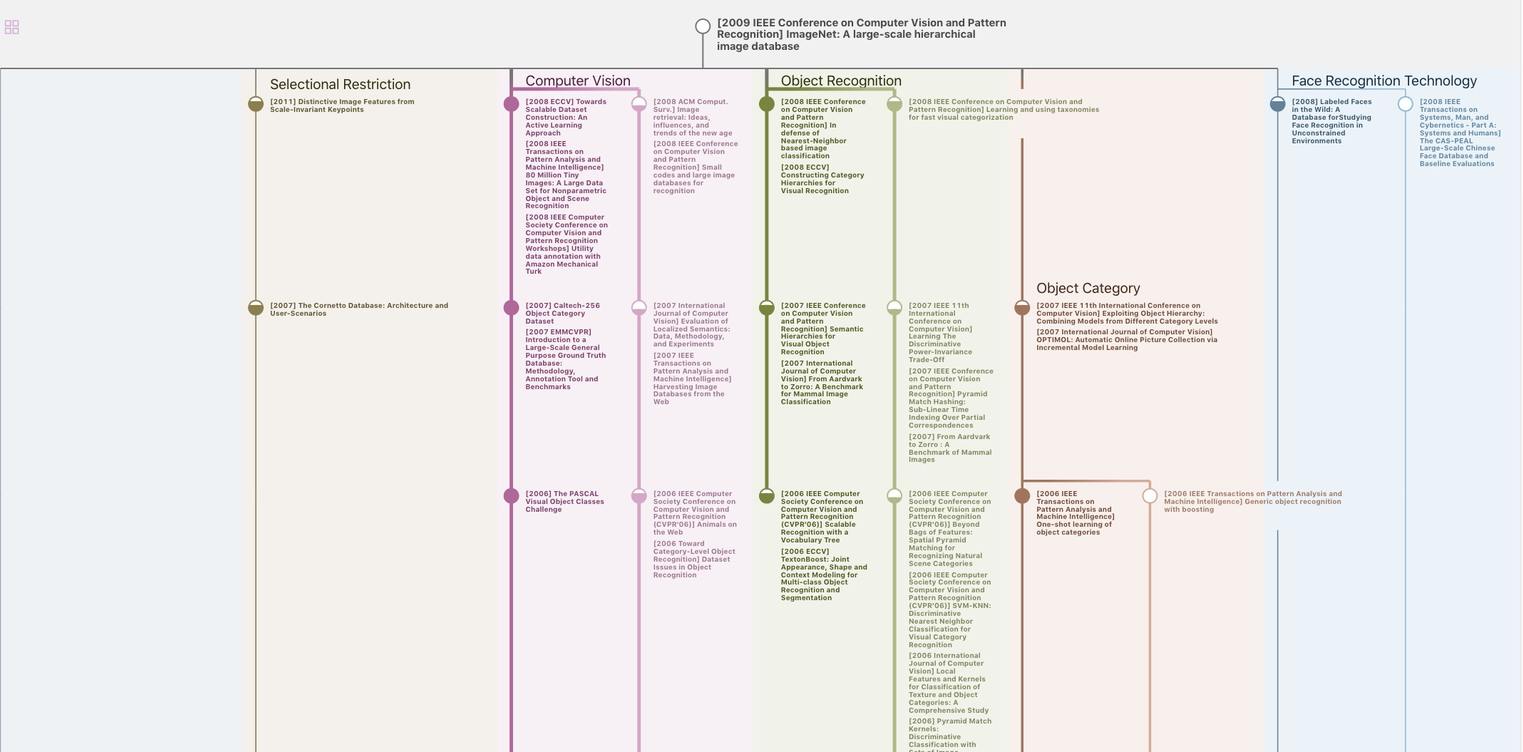
生成溯源树,研究论文发展脉络
Chat Paper
正在生成论文摘要