Towards parameter-independent data clustering and image segmentation.
Pattern Recognition(2016)
摘要
While there are a large amount of clustering algorithms proposed in the literature, the clustering results of existing algorithms usually depend on user-specified parameters heavily, and it is usually difficult to determine the optimal parameters. With the pairwise data similarity matrix as the input, dominant sets clustering has been shown to be an effective data clustering and image segmentation approach, partly due to its ability to find out the underlying data structure and determine the number of clusters automatically. However, we find that the original dominant sets algorithm is sensitive to the similarity measures used in building the similarity matrix. This means that parameter tuning is required to generate satisfactory clustering results, and dominant sets clustering results are also parameter dependent. In order to remove the dependence on the user-specified parameter, we study how the similarity measures influence the dominant sets clustering results. As a result, we propose to transform similarity matrices by histogram equalization before clustering. While this transformation is shown to remove the sensitiveness to similarity measures effectively, it also results in over-segmentation. Therefore in the next step we present a cluster extension method to overcome the over-segmentation effect and generate more reasonable clustering results. We test the enhanced clustering algorithm in both data clustering and image segmentation experiments, and comparisons with the state-of-the-art algorithms validate the effectiveness of our algorithm. HighlightsWe study how similarity measures influence the dominant sets clustering results.We use histogram equalization to remove the dependence on similarity parameters.We present a density based cluster extension method to overcome over-segmentation.Experiments validate the effectiveness of our algorithm.
更多查看译文
关键词
Dominant sets,Clustering,Image segmentation,Similarity matrix,Similarity measure
AI 理解论文
溯源树
样例
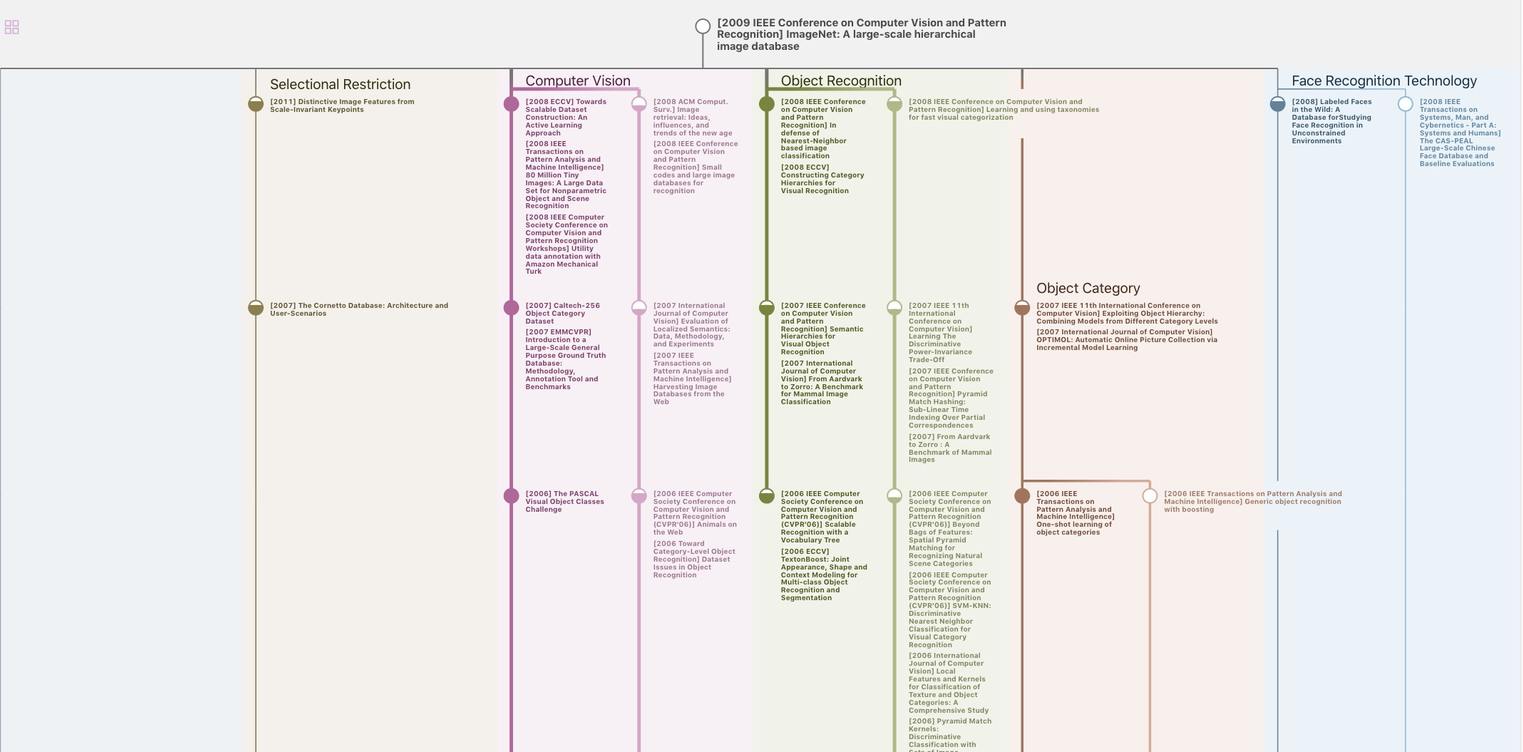
生成溯源树,研究论文发展脉络
Chat Paper
正在生成论文摘要