Deep Convolutional Networks With Superpixel Segmentation For Hyperspectral Image Classification
2016 IEEE International Geoscience and Remote Sensing Symposium (IGARSS)(2016)
摘要
To combat the well-known Hughes phenomenon occurred in hyperspectral classification, most of the previous works adopt dimensionality reduction or manifold learning technique before supervised learning. While in this paper, we propose a different scheme: First, we design a pixel-wise classifier based on Convolutional Neural Network that could directly mapping observed spectrum to class distribution. Then, we conduct superpixel segmentation on the prediction map that learned by previous model and output the final classification results by spatial and spectral factors jointly. Varied from other deep learning method, our classification framework learns and infers spectrum efficiently via deep hierarchy with convolutional and pooling layers, thus forming a direct relationship between high-order data and class distribution. Moreover, superpixel segmentation helps further boost the accuracy of the classification by combining the spatial information. In experimental studies, multiple hyperspectral datasets with various context and spatial resolution are used to validate the proposed method. The experimental results show that the proposed method is efficient and competitive in practical uses.
更多查看译文
关键词
Hyperspectral Image Classification,Deep Learning,Convolutional Neural Network,Superpixel Segmentation,Support Vector Machine
AI 理解论文
溯源树
样例
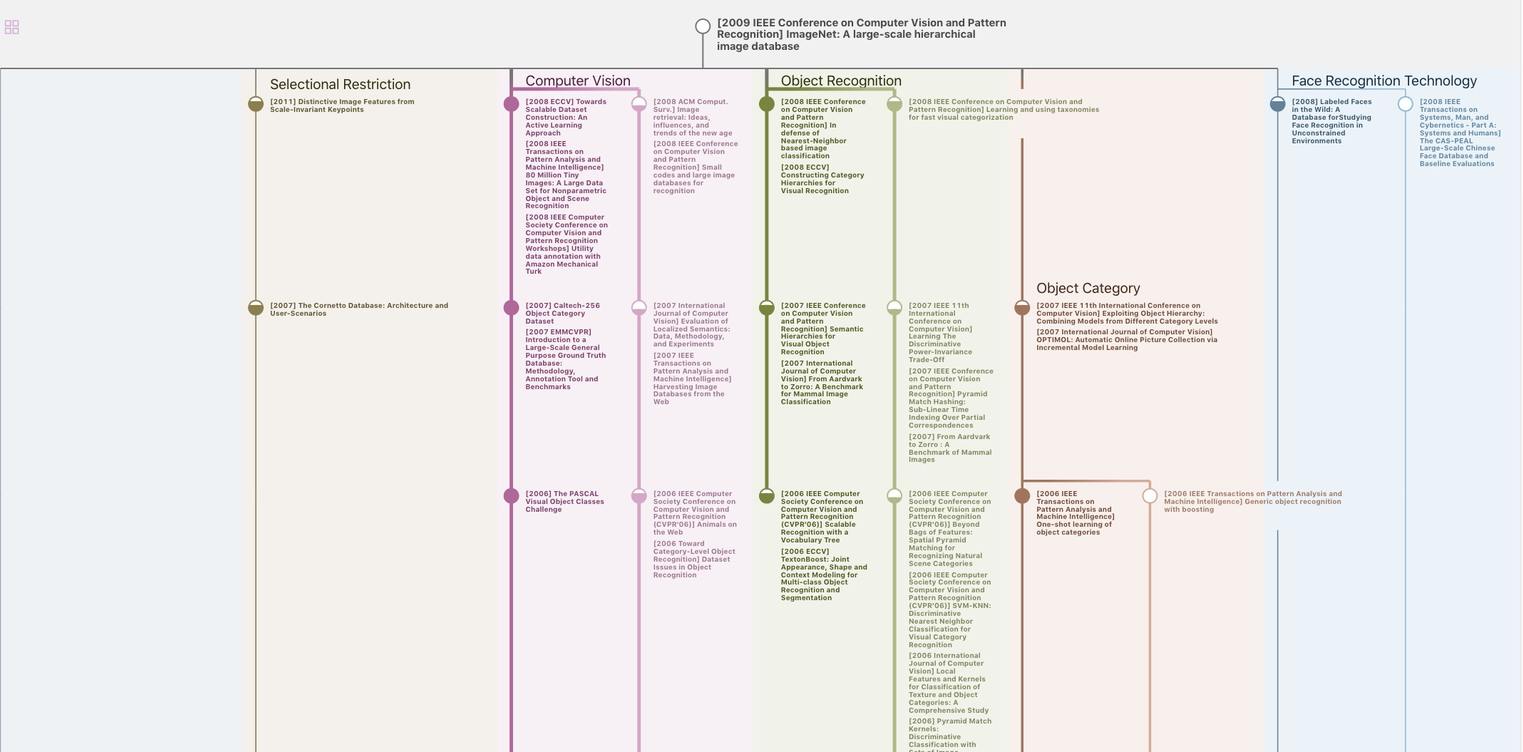
生成溯源树,研究论文发展脉络
Chat Paper
正在生成论文摘要