Anomaly Detection In Hyperspectral Images Through Spectral Unmixing And Low Rank Decomposition
2016 IEEE International Geoscience and Remote Sensing Symposium (IGARSS)(2016)
摘要
Anomaly detection has been known to be a challenging, illposed problem due to the uncertainty of anomaly and the interference of noise. In this paper, we propose a novel low rank anomaly detection algorithm in hyperspectral images (HSI), where three components are involved. First, due to the highly mixed nature of pixels in HSI, instead of using the raw pixel directly for anomaly detection, the proposed algorithm applies spectral unmixing algorithms to obtain the abundance vectors and uses these vectors for anomaly detection. Second, for better classification, a dictionary is built based on the mean-shift clustering of the abundance vectors to better represent the highly-correlated background and the sparse anomaly. Finally, a low-rank matrix decomposition is proposed to encourage the sparse coefficients of the dictionary to be low-rank, and the residual matrix to be sparse. Anomalies can then be extracted by summing up the columns of the residual matrix. The proposed algorithm is evaluated on both synthetic and real datasets. Experimental results show that the proposed approach constantly achieves high detection rate while maintaining low false alarm rate regardless of the type of images tested.
更多查看译文
关键词
Hyperspectral image,anomaly detection,mesn-shift clustering,low-rank,sparsity
AI 理解论文
溯源树
样例
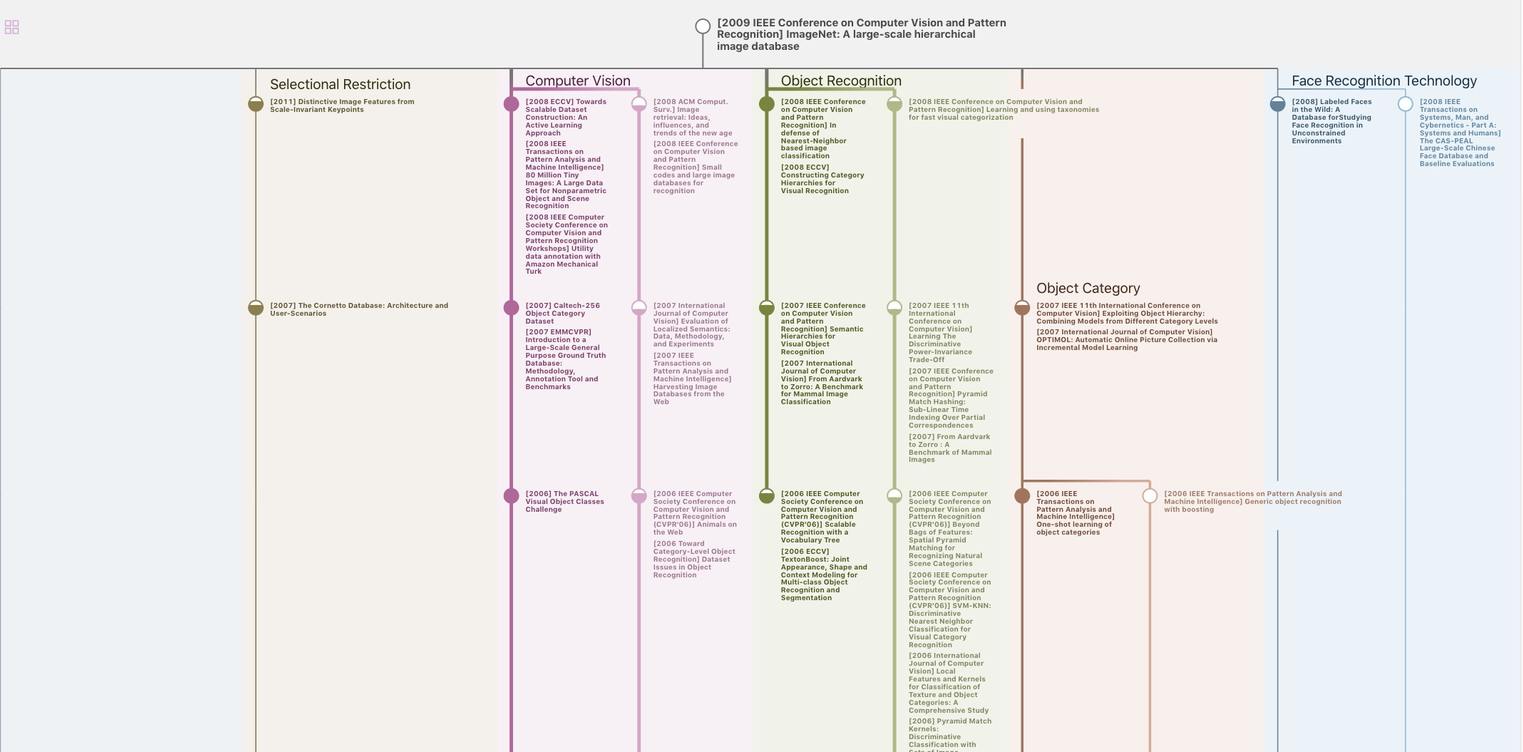
生成溯源树,研究论文发展脉络
Chat Paper
正在生成论文摘要