Empirical Evaluation of Cross-Release Effort-Aware Defect Prediction Models
2016 IEEE International Conference on Software Quality, Reliability and Security (QRS)(2016)
摘要
To prioritize quality assurance efforts, various fault prediction models have been proposed. However, the best performing fault prediction model is unknown due to three major drawbacks: (1) comparison of few fault prediction models considering small number of data sets, (2) use of evaluation measures that ignore testing efforts and (3) use of n-fold cross-validation instead of the more practical cross-release validation. To address these concerns, we conducted cross-release evaluation of 11 fault density prediction models using data sets collected from 2 releases of 25 open source software projects with an effort-aware performance measure known as Norm(P
opt
). Our result shows that, whilst M5 and K* had the best performances, they were greatly influenced by the percentage of faulty modules present and size of data set. Using Norm(P
opt
) produced an overall average performance of more than 50% across all the selected models clearly indicating the importance of considering testing efforts in building fault-prone prediction models.
更多查看译文
关键词
fault-density estimation,empirical study,open sourceproject,crossversionprediction,Demsar's significance diagram
AI 理解论文
溯源树
样例
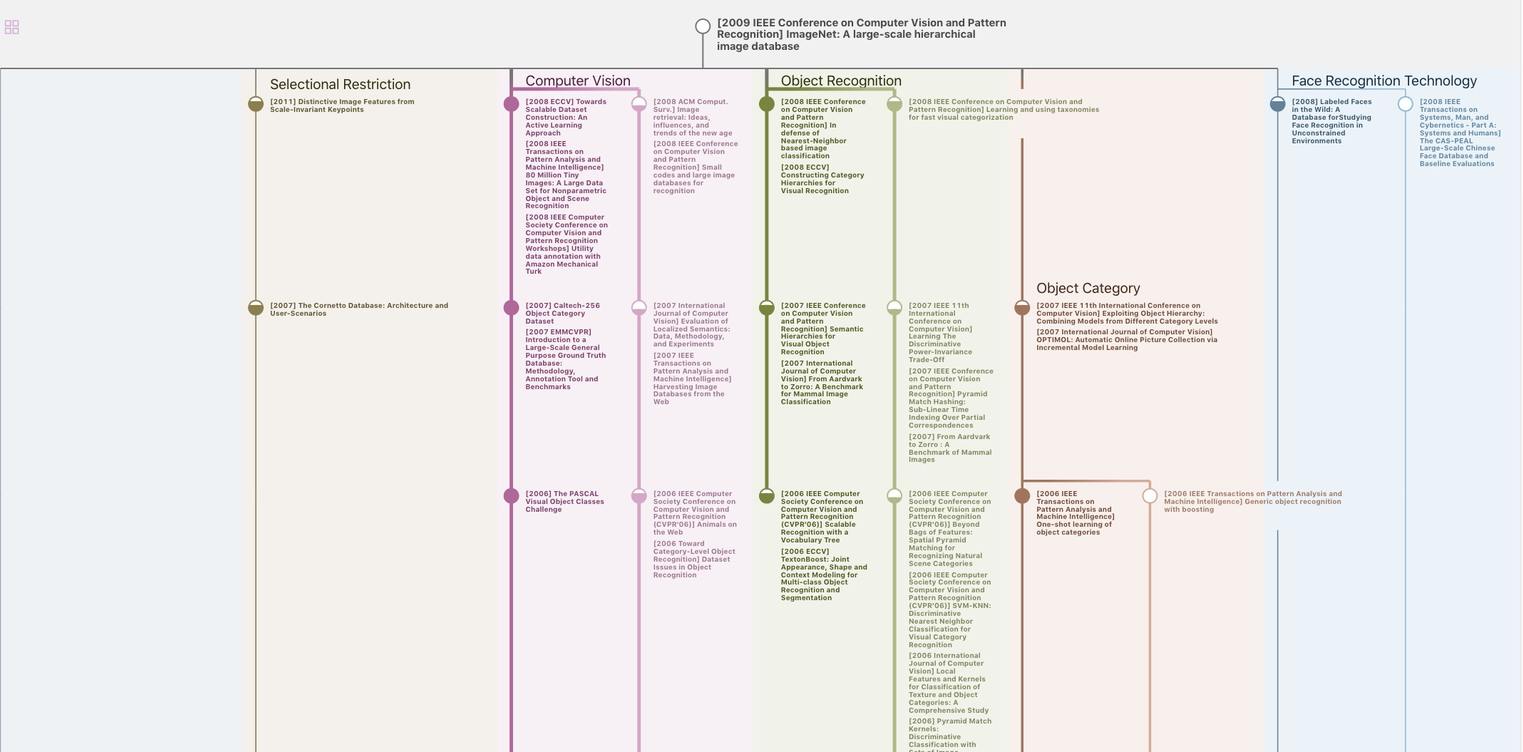
生成溯源树,研究论文发展脉络
Chat Paper
正在生成论文摘要