Scrutable Feature Sets for Stance Classification.
ArgMining@ACL(2016)
摘要
This paper describes and evaluates a novel feature set for stance classification of argumentative texts; i.e. deciding whether a post by a user is for or against the issue being debated. We model the debate both as attitude bearing features, including a set of automatically acquired ‘topic terms’ associated with a Distributional Lexical Model (DLM) that captures the writer’s attitude towards the topic term, and as dependency features that represent the points being made in the debate. The stance of the text towards the issue being debated is then learnt in a supervised framework as a function of these features. The main advantage of our feature set is that it is scrutable: The reasons for a classification can be explained to a human user in natural language. We also report that our method outperforms previous approaches to stance classification as well as a range of baselines based on sentiment analysis and topic-sentiment analysis.
更多查看译文
AI 理解论文
溯源树
样例
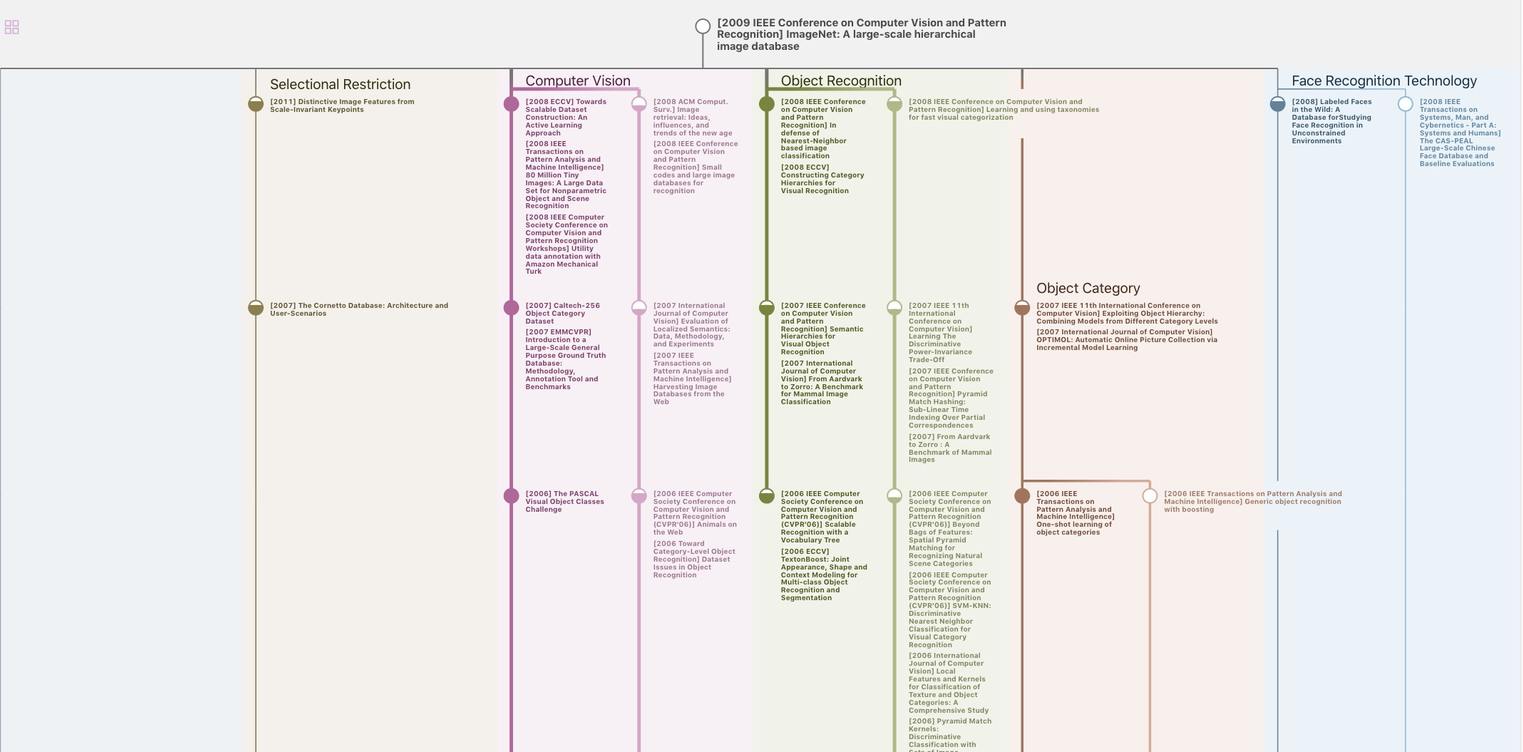
生成溯源树,研究论文发展脉络
Chat Paper
正在生成论文摘要