Enhancing Location Prediction With Big Data: Evidence From Dhaka
UbiComp '16: The 2016 ACM International Joint Conference on Pervasive and Ubiquitous Computing Heidelberg Germany September, 2016(2016)
摘要
In recent years, the study of location prediction has received heightened attention due to its applications in LBS and other areas. However, most of the techniques and subsequent conclusions drawn from previous research works are specific to the data used in the study. For instance, resolution of location data and inclusion of external data (e.g., from social networks) may limit application of previous techniques to new situations. Therefore, we explore ways of enhancing location prediction techniques which leverage big data without the need for external data sources. To this end, we study a large CDR dataset with more than 3.5 billion calls from a leading cellular network provider in Dhaka, Bangladesh. The research question we tackle is how we can leverage big data to enhance performance of location predictors? Based on spatio-temporal analysis of call activity, we devise a scheme to compute prior probabilities from cell call activity. With this reasoning, we develop an enhanced Bayes predictor which uses a distance threshold and the users' regular location to improve generation of prior probabilities. Experimental results show that overall the enhanced Bayes predictor improves accuracy by 17 percentage points.
更多查看译文
关键词
Data mining,Human mobility,Location prediction,Supervised classification
AI 理解论文
溯源树
样例
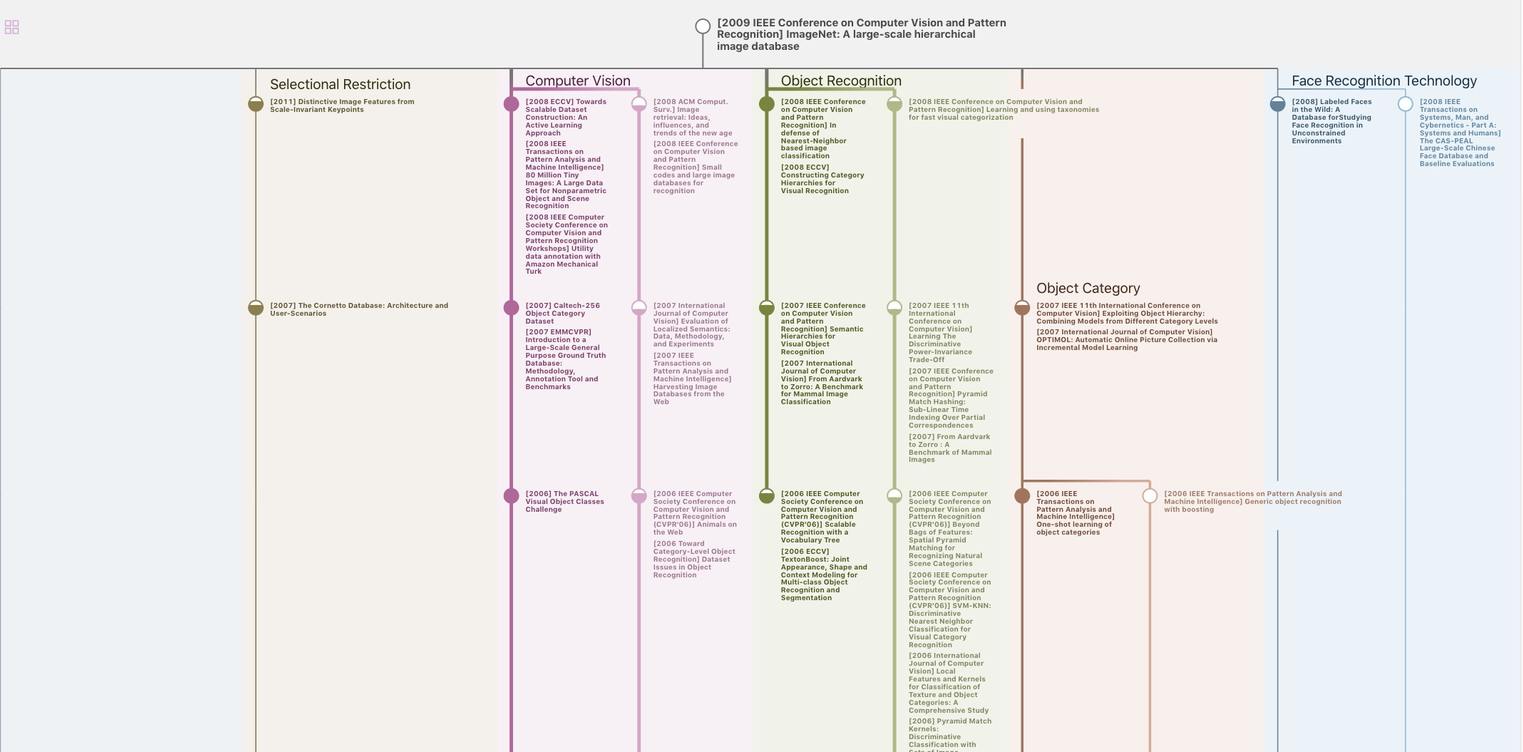
生成溯源树,研究论文发展脉络
Chat Paper
正在生成论文摘要