Evolving Deep Neural Networks : A New Prospect
2016 12TH INTERNATIONAL CONFERENCE ON NATURAL COMPUTATION, FUZZY SYSTEMS AND KNOWLEDGE DISCOVERY (ICNC-FSKD)(2016)
摘要
The success of Deep Neural Networks (DNNs) for various applications like language processing (NLP), image processing, character recognition inspired to use machine learning (ML) and Evolutionary Computation (EC) techniques for improving learning process. Using evolutionary algorithms to improve the efficiency of deep learning attained some success. However, these techniques are unable to reduce the learning time which is the key concern for deep learning. The main problem with DNN is that, it uses a random topology to start with (similar to artificial neural networks). If the topology is not suitable, training procedure will restart with a new topology and this process continues till expected results are obtained.In this paper, we propose, for the first time, a new prospect for evolving optimized deep neural networks which can provide a warm start to the training process compared to heuristic random initial architecture. We discuss the theoretical approach towards possibility of optimizing the learning process inspired from the existing un-conventional approaches. The training process of DNN with EC approach is faster than regular approach by a considerable difference of over 6 hours for MNIST data set. Further, we observed a considerable improvement in the classification accuracies. Our approaches resulted in an improved classification accuracy of 2% and 4.4% for MNIST data set and 1.2% and 1.4% for IRIS data set compared to heuristic random weights approach. Our initial experimental results prove that evolutionary approaches provides a warm start to the deep learning, thus, reducing the training time.
更多查看译文
关键词
Evolving Neural Networks,Deep Neural Networks,Multi-population evolution,Co-evolution
AI 理解论文
溯源树
样例
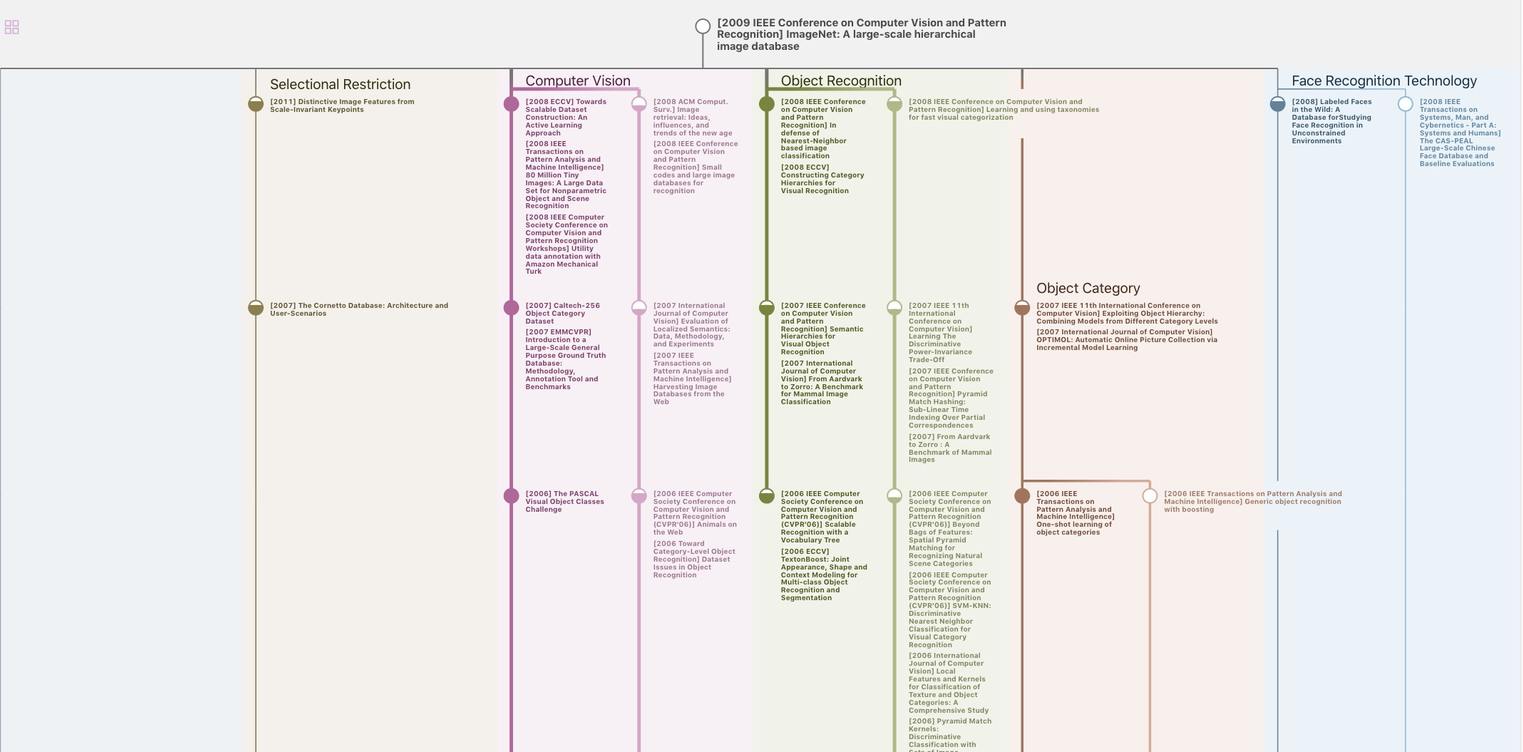
生成溯源树,研究论文发展脉络
Chat Paper
正在生成论文摘要