Distributed Learning of Random Weights Fuzzy Neural Networks
IEEE International Conference on Fuzzy Systems(2016)
摘要
In this paper, we propose a scalable, decentralized learning algorithm for Random Weights Fuzzy Neural Networks, when training data is distributed through a network of interconnected computing agents. In this scenario, the aim is for all the agents to converge to a single model, with the requirement that only local communications between the agents are permitted. In this work we assume that all the agents know the parameters of the antecedents, while the parameters of the consequents are estimated by using the Alternating Direction Method of Multipliers strategy. Experimental results show that the performance of the proposed algorithm is comparable to that of a centralized model, where all the data is collected by a single agent before the training process. To this date, this is the first publication that addressed the problem of training a fuzzy neural network over a fully decentralized infrastructure.
更多查看译文
关键词
distributed online learning,random-weight fuzzy neural networks,inference system,fuzzy rule parameters,membership functions,regularized least squares algorithm,interconnected agents,distributed average consensus protocol,centralized training set
AI 理解论文
溯源树
样例
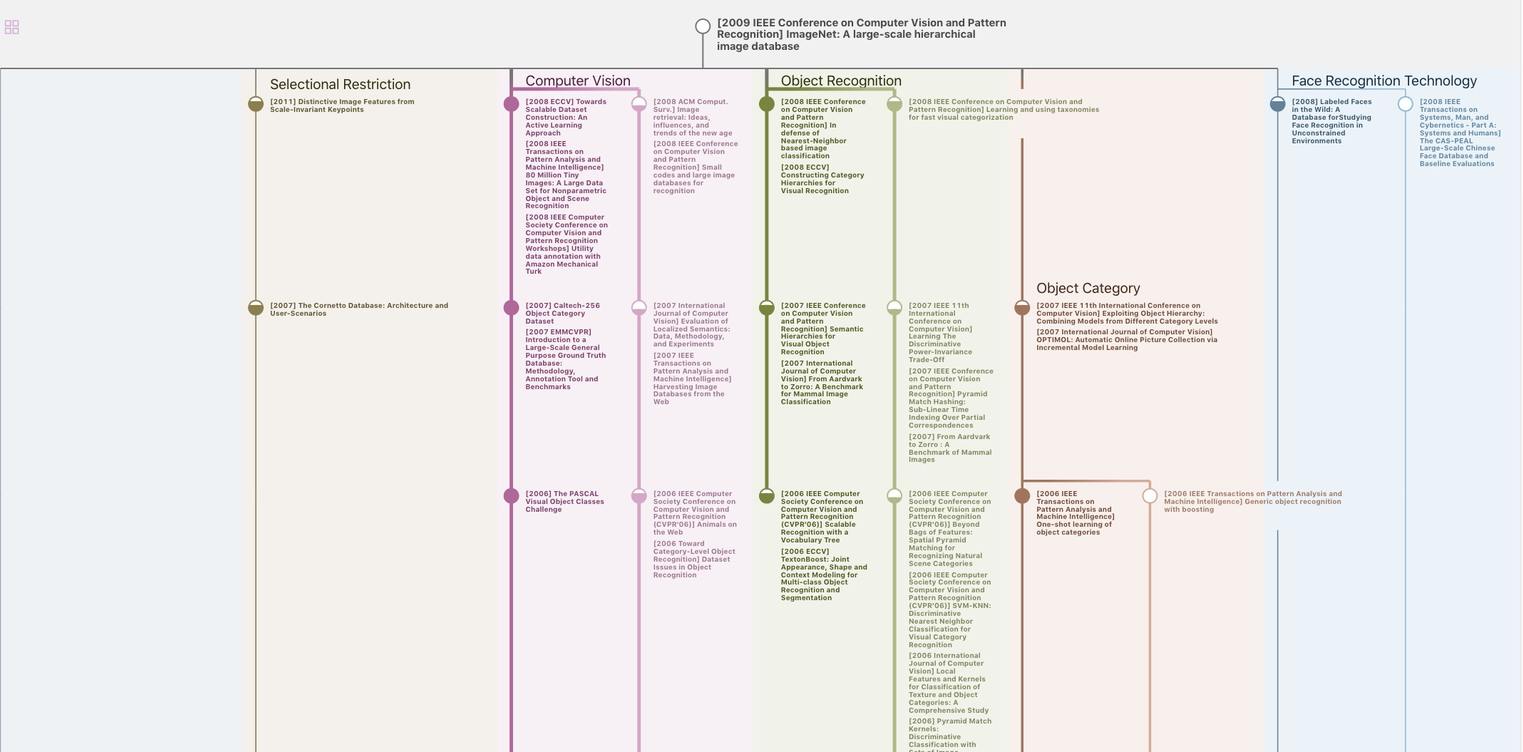
生成溯源树,研究论文发展脉络
Chat Paper
正在生成论文摘要