Single Image Super-resolution Based on Self-Similarity and Dictionary Neighborhood
2016 IEEE 14th Intl Conf on Dependable, Autonomic and Secure Computing, 14th Intl Conf on Pervasive Intelligence and Computing, 2nd Intl Conf on Big Data Intelligence and Computing and Cyber Science and Technology Congress(DASC/PiCom/DataCom/CyberSciTech)(2016)
摘要
This paper proposes a method of super-resolution reconstruction based on self-similarity and dictionary neighborhood. Unlike most learning-based super-resolution algorithm, this method does not require an external image in the training phase. Moreover, the image itself and cross-scale image similarity are utilized to generate an image pyramid. Then this method uses the image pyramid to generate an image set containing of high/low resolution image pairs to train a corresponding high/low dictionary. In the reconstruction phase, according to the feature of each image patch of the input image, we select the neighborhood of corresponding dictionary atom, rather than using the entire dictionary. When solving the coefficients, most methods usually select L1 norm, the proposed method uses L2 norm in order to improve computing speed. Experimental results show that, compared with the conventional methods, the proposed method has improved the PSNR of the reconstructed image and the subjective visual effect, while the space requirements are reduced.
更多查看译文
关键词
super-resolution,self-similarity,dictionary neighborhood,ridge regression
AI 理解论文
溯源树
样例
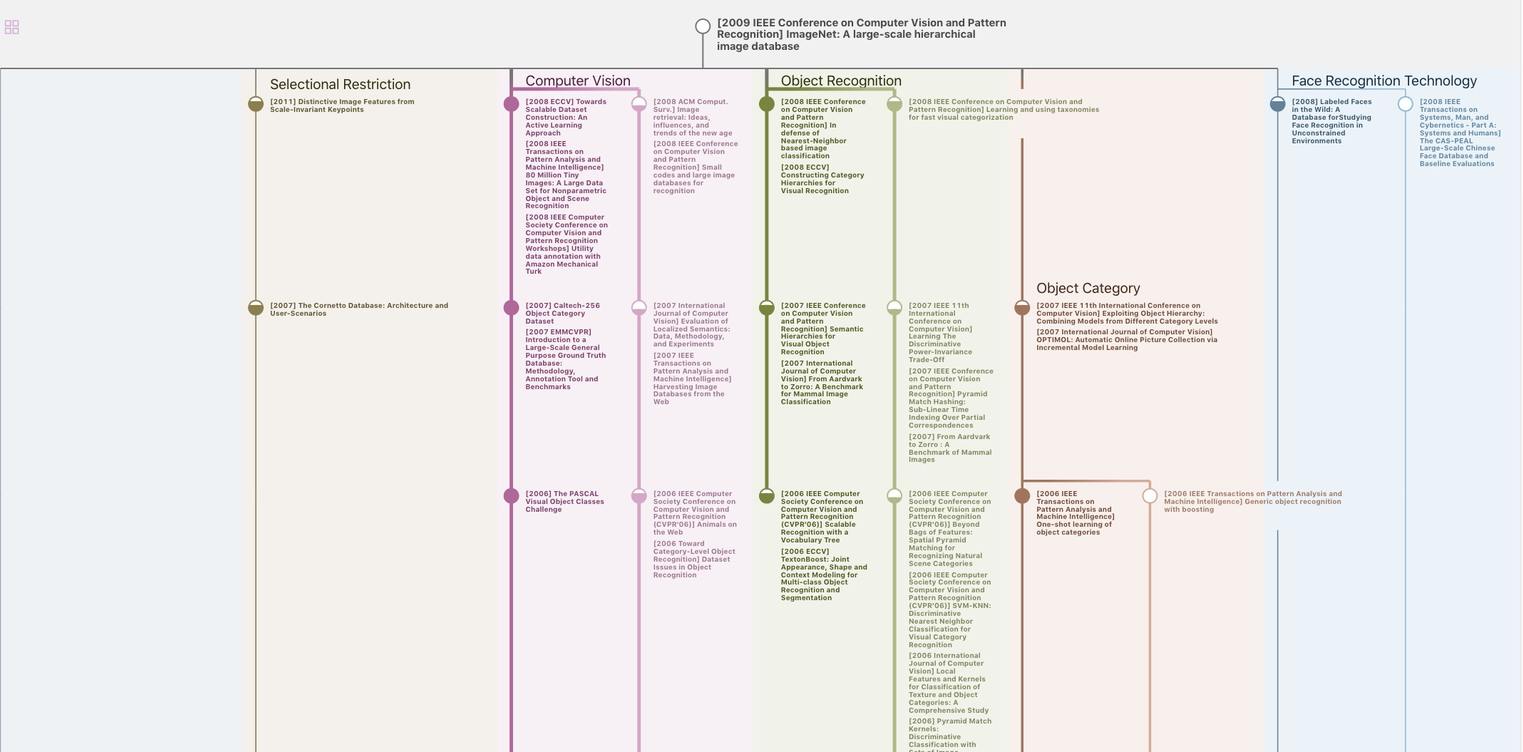
生成溯源树,研究论文发展脉络
Chat Paper
正在生成论文摘要