Hidden-Markov Factor analysis as a spatiotemporal model for electrocorticography.
2016 38th Annual International Conference of the IEEE Engineering in Medicine and Biology Society (EMBC)(2016)
摘要
We present a new approach to extracting low-dimensional neural trajectories that summarize the electrocorticographic (ECoG) signals recorded with high-channel-count electrode arrays implanted subdurally. In our approach, Hidden-Markov Factor Analysis (HMFA), a finite set of factor analyzers are used to model the relationship between the high-dimensional ECoG neural space and a low-dimensional latent neural space; the factor analyzers at different time points are in turn linked together with a hidden Markov model. The recorded ECoG signals were band-pass filtered such that our analysis was focused on a sub-band (76-100Hz) of high gamma. HMFA affords the quantization of the ECoG neural space and dimensionality reduction in a common probabilistic space. We applied this method to the ECoG recordings of 2 subjects who responded with button presses to audiovisual stimuli in an experimental task. Using a goodness-of-fit metric that measures how well the ECoG activity of each electrode can be predicted by all the other electrodes, we found that HMFA performed best when compared with Gaussian-Process Factor Analysis (GPFA) and other related spatiotemporal modeling techniques. In contradistinction to HMFA, GPFA and the other techniques integrate temporal smoothing with dimensionality reduction. We believe that this method will provide a powerful tool for relating high-channel-count ECoG signals to the perception and behavior of subjects.
更多查看译文
关键词
Electrocorticography,Electrodes, Implanted,Electroencephalography,Factor Analysis, Statistical,Normal Distribution
AI 理解论文
溯源树
样例
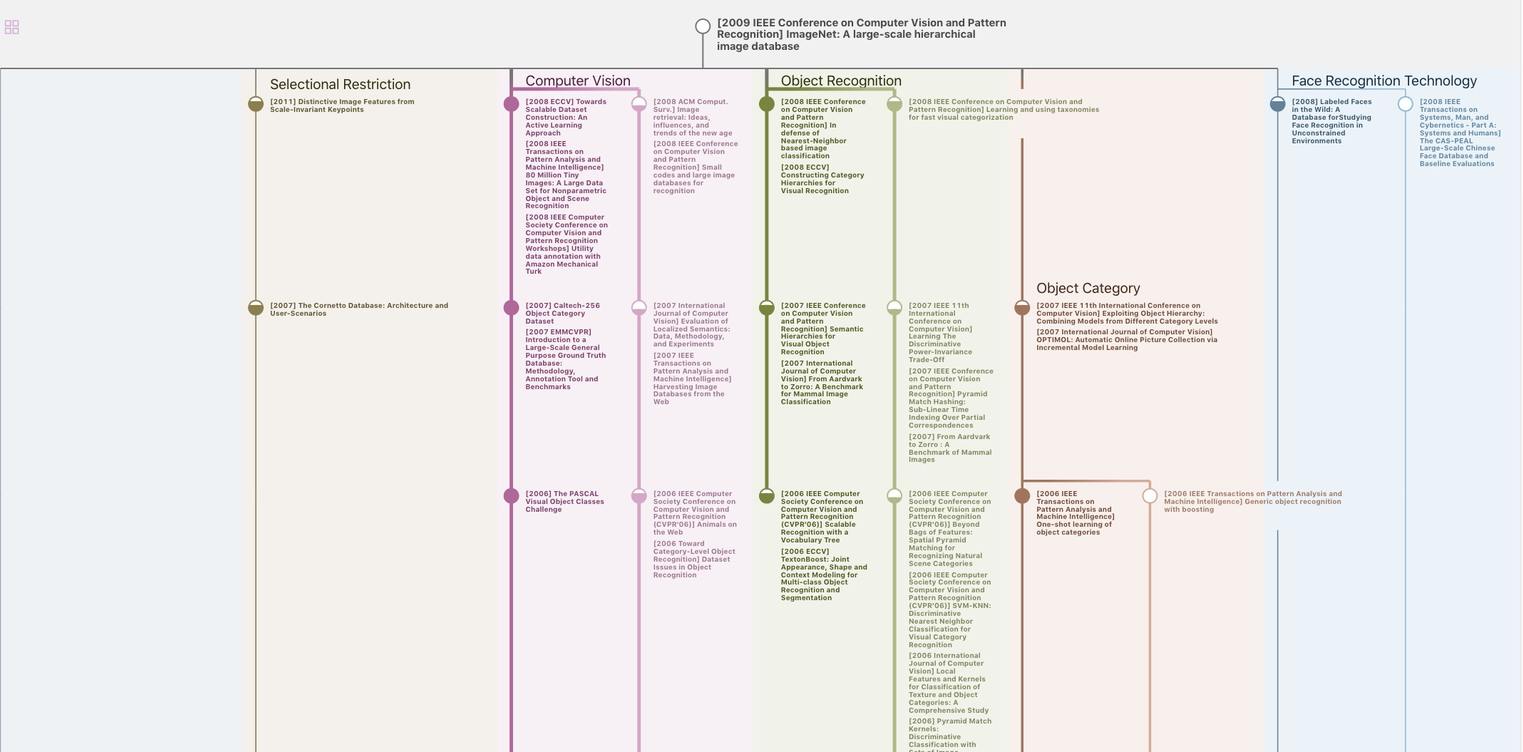
生成溯源树,研究论文发展脉络
Chat Paper
正在生成论文摘要