A Gaussian Bayesian Model To Identify Spatio-Temporal Causalities For Air Pollution Based On Urban Big Data
2016 IEEE CONFERENCE ON COMPUTER COMMUNICATIONS WORKSHOPS (INFOCOM WKSHPS)(2016)
摘要
Identifying the causalities for air pollutants and answering questions, such as, where do Beijing's air pollutants come from, are crucial to inform government decision-making. In this paper, we identify the spatio-temporal (ST) causalities among air pollutants at different locations by mining the urban big data. This is challenging for two reasons: 1) since air pollutants can be generated locally or dispersed from the neighborhood, we need to discover the causes in the ST space from many candidate locations with time efficiency; 2) the cause-and-effect relations between air pollutants are further affected by confounding variables like meteorology. To tackle these problems, we propose a coupled Gaussian Bayesian model with two components: 1) a Gaussian Bayesian Network (GBN) to represent the cause-and-effect relations among air pollutants, with an entropy-based algorithm to efficiently locate the causes in the ST space; 2) a coupled model that combines cause-and-effect relations with meteorology to better learn the parameters while eliminating the impact of confounding. The proposed model is verified using air quality and meteorological data from 52 cities over the period Jun 1st 2013 to May 1st 2015. Results show superiority of our model beyond baseline causality learning methods, in both time efficiency and prediction accuracy.
更多查看译文
关键词
Causality analysis,Bayesian network (BN),urban big data,spatio-temporal (ST),air pollution
AI 理解论文
溯源树
样例
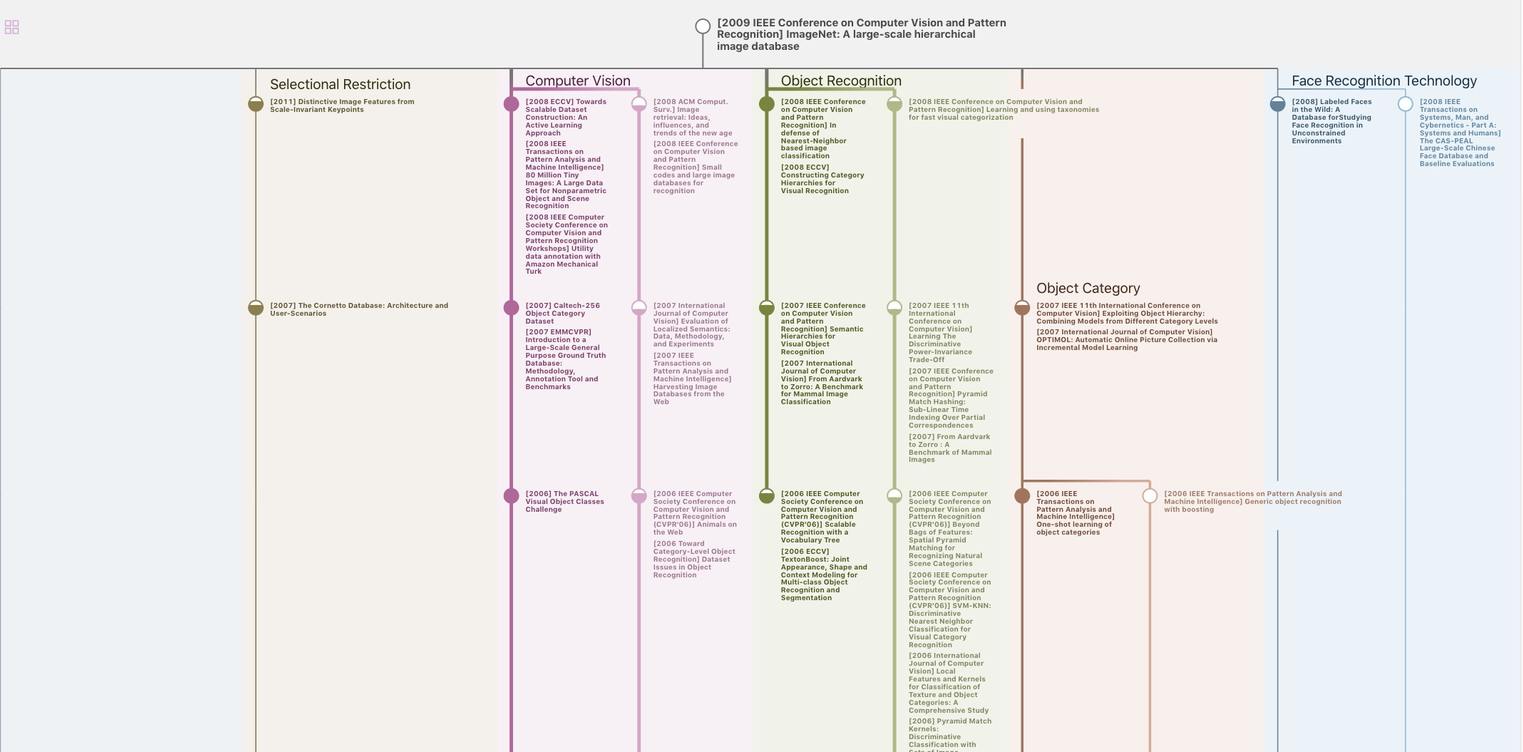
生成溯源树,研究论文发展脉络
Chat Paper
正在生成论文摘要