Joint Time Invariant And Time Dependent Brain Connectivity Network Estimation
2016 IEEE Canadian Conference on Electrical and Computer Engineering (CCECE)(2016)
摘要
Inferring interactions between discrete brain regions has being increasingly recognized as important for studying brain in both normal and disease states. In addition to static brain network inference, the temporal dynamics of brain connectivity access the brain in the temporal dimension and provide a new perspective to the understanding of brain function. Most current brain network modeling approaches are based on assumptions that brain connections are purely static or purely dynamic. This may be unrealistic as the brain must strike a balance between stability and flexibility. In this paper, we propose making joint inference of time invariant connections and time varying coupling patterns by employing a multitask learning model followed by a least square approach to precisely estimate the connectivity coefficients. When applied to a real resting state fMRI study, the eigenconnectivity networks were extracted to obtain the representative patterns of both static and dynamic brain connectivity networks. The results demonstrated that the static and dynamic connectivity networks may represent complementary information on the brain connectivity patterns.
更多查看译文
关键词
joint time invariant-time dependent brain connectivity network estimation,discrete brain regions,disease states,static brain network inference,brain connectivity temporal dynamics,brain network modeling approaches,time invariant connections,time varying coupling patterns,multitask learning model,least square approach,connectivity coefficient estimation,real resting state fMRI study,eigenconnectivity networks,dynamic brain connectivity networks,static brain connectivity networks
AI 理解论文
溯源树
样例
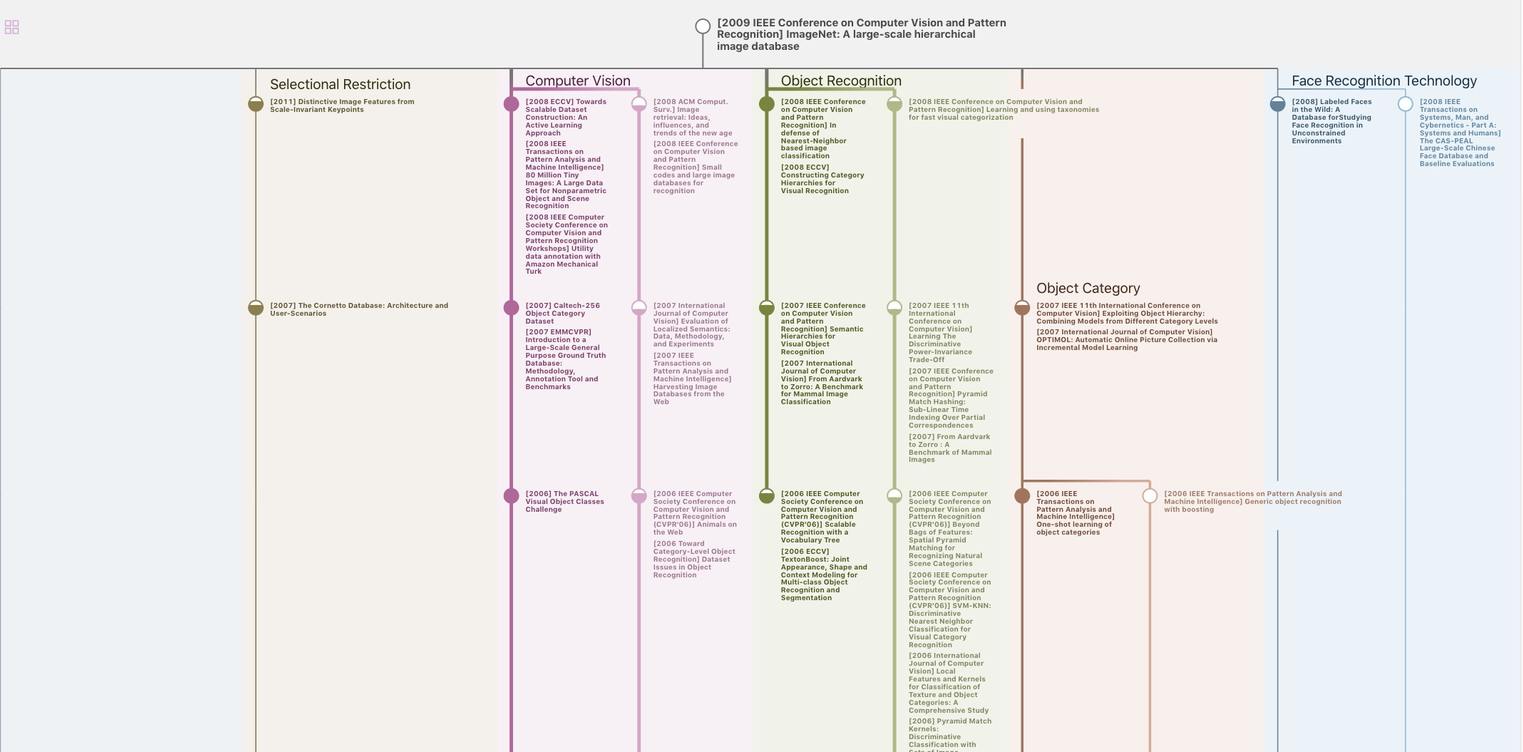
生成溯源树,研究论文发展脉络
Chat Paper
正在生成论文摘要