Learning High-Level Navigation Strategies via Inverse Reinforcement Learning: A Comparative Analysis.
Australasian Conference on Artificial Intelligence(2016)
摘要
With an increasing number of robots acting in populated environments, there is an emerging necessity for programming techniques that allow for efficient adjustment of the robot’s behavior to new environments or tasks. A promising approach for teaching robots a certain behavior is Inverse Reinforcement Learning (IRL), which estimates the underlying reward function of a Markov Decision Process (MDP) from observed behavior of an expert. Recently, an approach called Simultaneous Estimation of Rewards and Dynamics (SERD) has been proposed, which extends IRL by simultaneously estimating the dynamics. The objective of this work is to compare classical IRL algorithms with SERD for learning high level navigation strategies in a realistic hallway navigation scenario solely from human expert demonstrations. We show that the theoretical advantages of SERD also pay off in practice by estimating better models of the dynamics and explaining the expert’s demonstrations more accurately.
更多查看译文
关键词
Inverse Reinforcement Learning, Simultaneous Estimation of Rewards and Dynamics, Reinforcement Learning, Learning from demonstration
AI 理解论文
溯源树
样例
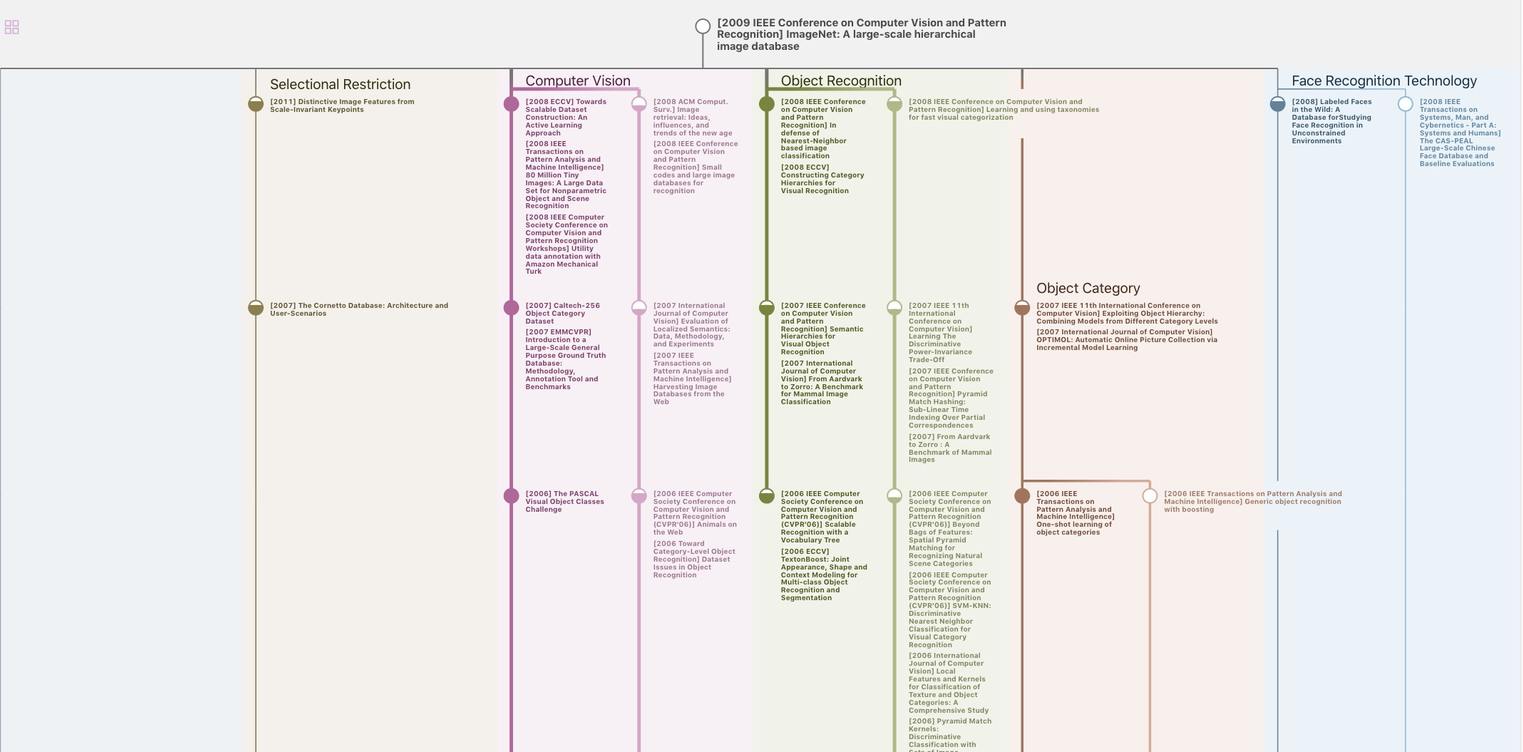
生成溯源树,研究论文发展脉络
Chat Paper
正在生成论文摘要