Self Organized Scale-Free Distributed Classification Using A Distributed, Unreliable Set Of Limited Capability Components
2016 ANNUAL IEEE SYSTEMS CONFERENCE (SYSCON)(2016)
摘要
Bandwidth requirements for networks that monitor the environment often result in scale limitations. Such limitations can be avoided by applying in-network data classification to reduce required bandwidth, however, traditional strategies that require a priori network configuration become expensive as networks begin to scale. We describe a self-organizing strategy that allows a scale-free set of independent sensing components to operate as a distributed classification system. A shared storage protocol is run over a set of simple, distributed physical components, which creates an abstraction of a reliable in-network storage device. The emergent storage device is used to record classification templates. Each component that encounters environmental information uses data in the network to perform data classification, and collaborates with neighbours to increase the information content. Consensus techniques are used to disambiguate between conflicting classifications. We apply the strategy to implement a distributed face recognition implementation. The strategy allows macro-level configuration of a self organizing process that supports an application. New deployment paradigms that depend on an extremely large number of components, such as nano-technology, are well suited to benefit from the strategy.
更多查看译文
关键词
self-organized scale-free distributed classification,limited capability components,distributed unreliable set,in-network data classification,a priori network configuration,independent sensing components,shared storage protocol,distributed physical components,in-network storage device,classification templates,environmental information,information content,consensus techniques,distributed face recognition implementation,macrolevel configuration
AI 理解论文
溯源树
样例
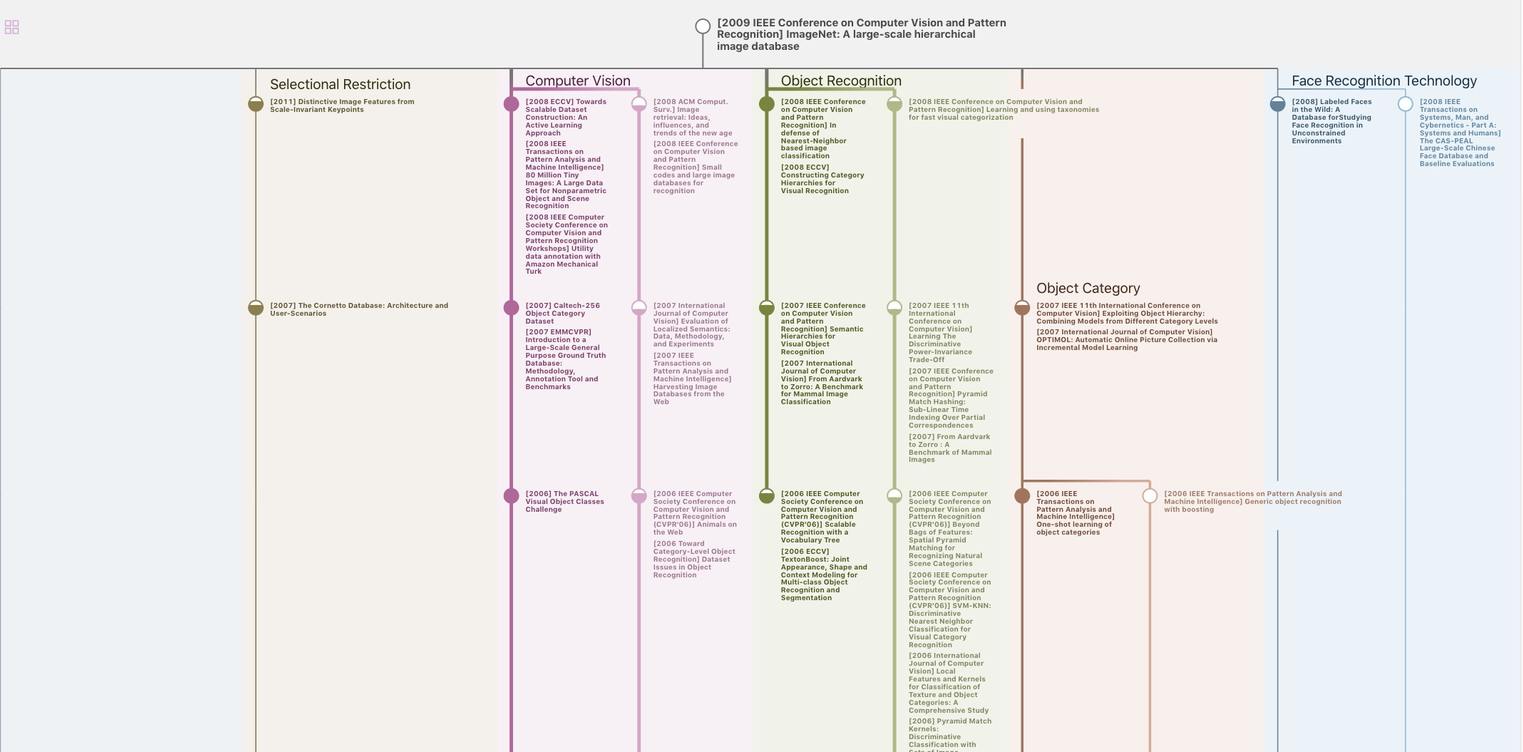
生成溯源树,研究论文发展脉络
Chat Paper
正在生成论文摘要