Overcoming Occlusion With Inverse Graphics
COMPUTER VISION - ECCV 2016 WORKSHOPS, PT III(2016)
摘要
Scene understanding tasks such as the prediction of object pose, shape, appearance and illumination are hampered by the occlusions often found in images. We propose a vision-as-inverse-graphics approach to handle these occlusions by making use of a graphics renderer in combination with a robust generative model (GM). Since searching over scene factors to obtain the best match for an image is very inefficient, we make use of a recognition model (RM) trained on synthetic data to initialize the search. This paper addresses two issues: (i) We study how the inferences are affected by the degree of occlusion of the foreground object, and show that a robust GM which includes an outlier model to account for occlusions works significantly better than a non-robust model. (ii) We characterize the performance of the RM and the gains that can be made by refining the search using the GM, using a new dataset that includes background clutter and occlusions. We find that pose and shape are predicted very well by the RM, but appearance and especially illumination less so. However, accuracy on these latter two factors can be clearly improved with the generative model.
更多查看译文
关键词
Vision-as-inverse-graphics,Scene understanding,Occlusion
AI 理解论文
溯源树
样例
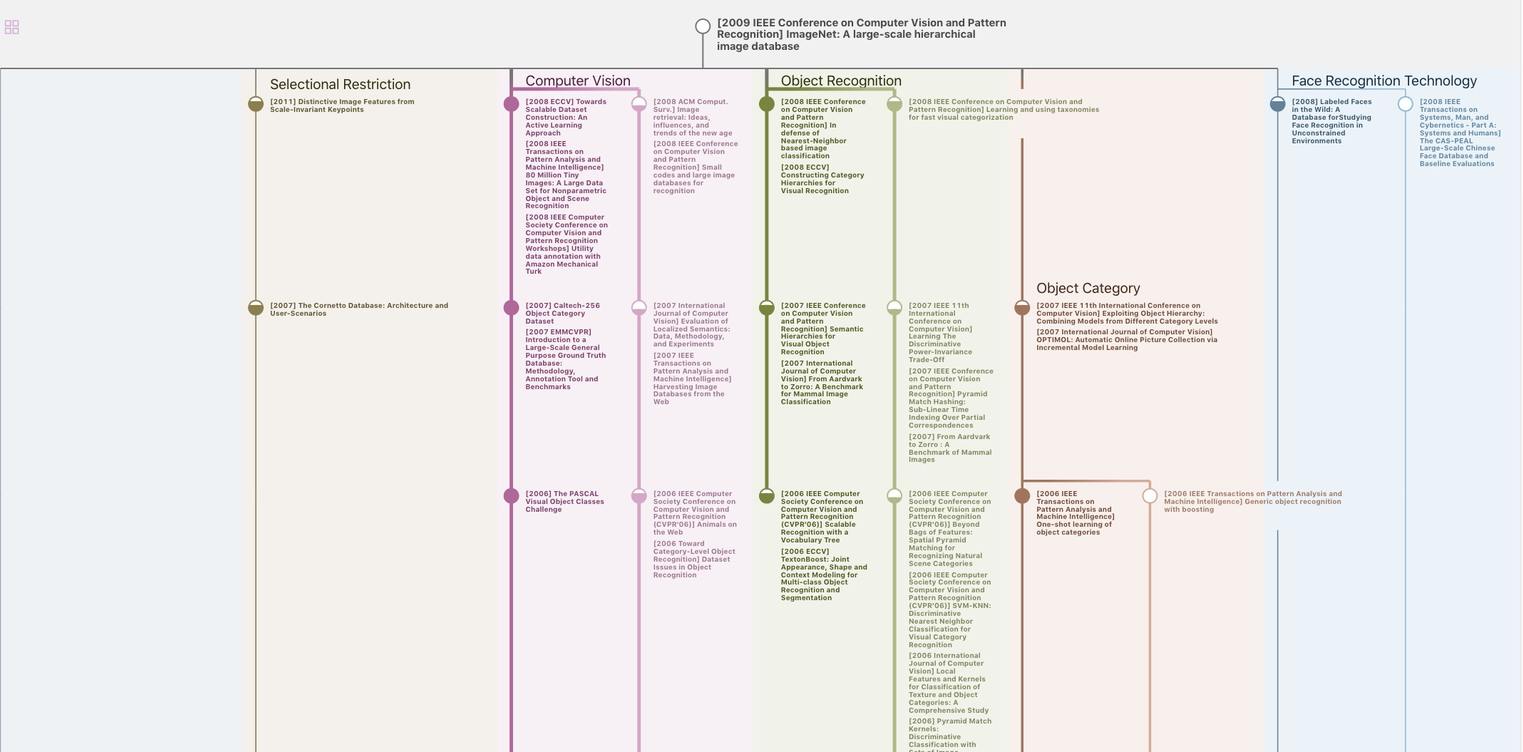
生成溯源树,研究论文发展脉络
Chat Paper
正在生成论文摘要