Improving NoC performance under spatio-temporal variability by runtime reconfiguration: a general mathematical framework
2016 Tenth IEEE/ACM International Symposium on Networks-on-Chip (NOCS)(2016)
摘要
Real-world applications exhibit time varying traffic volumes and patterns (structures). However, regular or application specific networks-on-chip (NoC) optimized at design time are predominantly developed either under the assumption of time independent application structures and dynamics, or without considering them at all. The limited adaptability of the monolithic NoCs to spatio-temporal application variability results in poor performance and power inefficient designs. Most of prior reconfiguration approaches are evaluated and validated through a subset of experimental instances out of the entire problem space, resulting in a constrained applicability to general cases. Towards this end, we propose a general mathematical framework for reconfigurable NoC design and runtime optimization under spatio-temporal varying workloads. Our proposed mathematical modeling framework can be applied to NoCs of arbitrary topologies, sizes and routing protocols. More precisely, we first propose the general mathematical modeling for reconfigurable NoC systems. Then, as a case study, we formulate the runtime NoC reconfiguration as an optimization problem assuming applications modeled via time dependent graphical models. We show this problem is NP-hard and prove its submodularity. This submodularity property provides guarantees that our proposed greedy algorithms attaints optimality region. To further justify the mathematical framework, we investigate a reconfigurable NoC architecture and evaluate it under real-world applications. Experimental results demonstrate a 52.3% latency improvement and a 30.2% energy reduction compared to baseline design.
更多查看译文
关键词
spatiotemporal variability,runtime reconfiguration,mathematical framework,networks-on-chip,runtime optimization,reconfigurable NoC system,time dependent graphical model,NP-hard problem,submodularity property,greedy algorithm,energy reduction
AI 理解论文
溯源树
样例
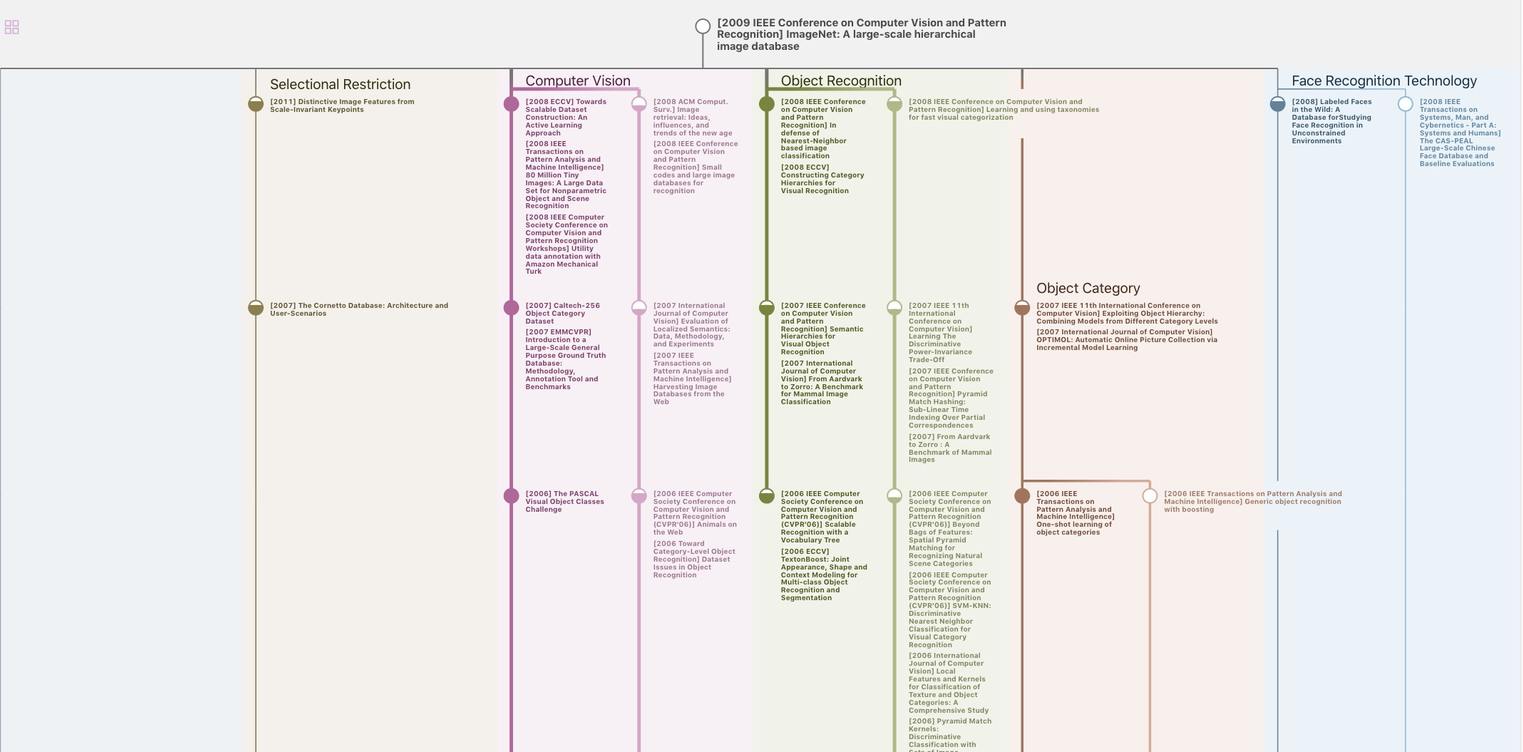
生成溯源树,研究论文发展脉络
Chat Paper
正在生成论文摘要