PDF: A Probabilistic Data Fusion Framework for Retrieval and Ranking
ICTIR(2016)
摘要
Data fusion has been shown to be a simple and effective way to improve retrieval results. Most existing data fusion methods combine ranked lists from different retrieval functions for a single given query. But in many real search settings, the diversity of retrieval functions required to achieve good fusion performance is not available. Researchers are typically limited to a few variants on a scoring function used by the engine of their choice, with these variants often producing similar results due to being based on the same underlying term statistics. This paper presents a framework for data fusion based on combining ranked lists from different queries that users could have entered for their information need. If we can identify a set of \"possible queries\" for an information need, and estimate probability distributions concerning the probability of generating those queries, the probability of retrieving certain documents for those queries, and the probability of documents being relevant to that information need, we have the potential to dramatically improve results over a baseline system given a single user query. Our framework is based on several component models that can be mixed and matched. We present several simple estimation methods for components. In order to demonstrate effectiveness, we present experimental results on 5 different datasets covering tasks such as ad-hoc search, novelty and diversity search, and search in the presence of implicit user feedback. Our results show strong performances for our method; it is competitive with state-of-the-art methods on the same datasets, and in some cases outperforms them.
更多查看译文
AI 理解论文
溯源树
样例
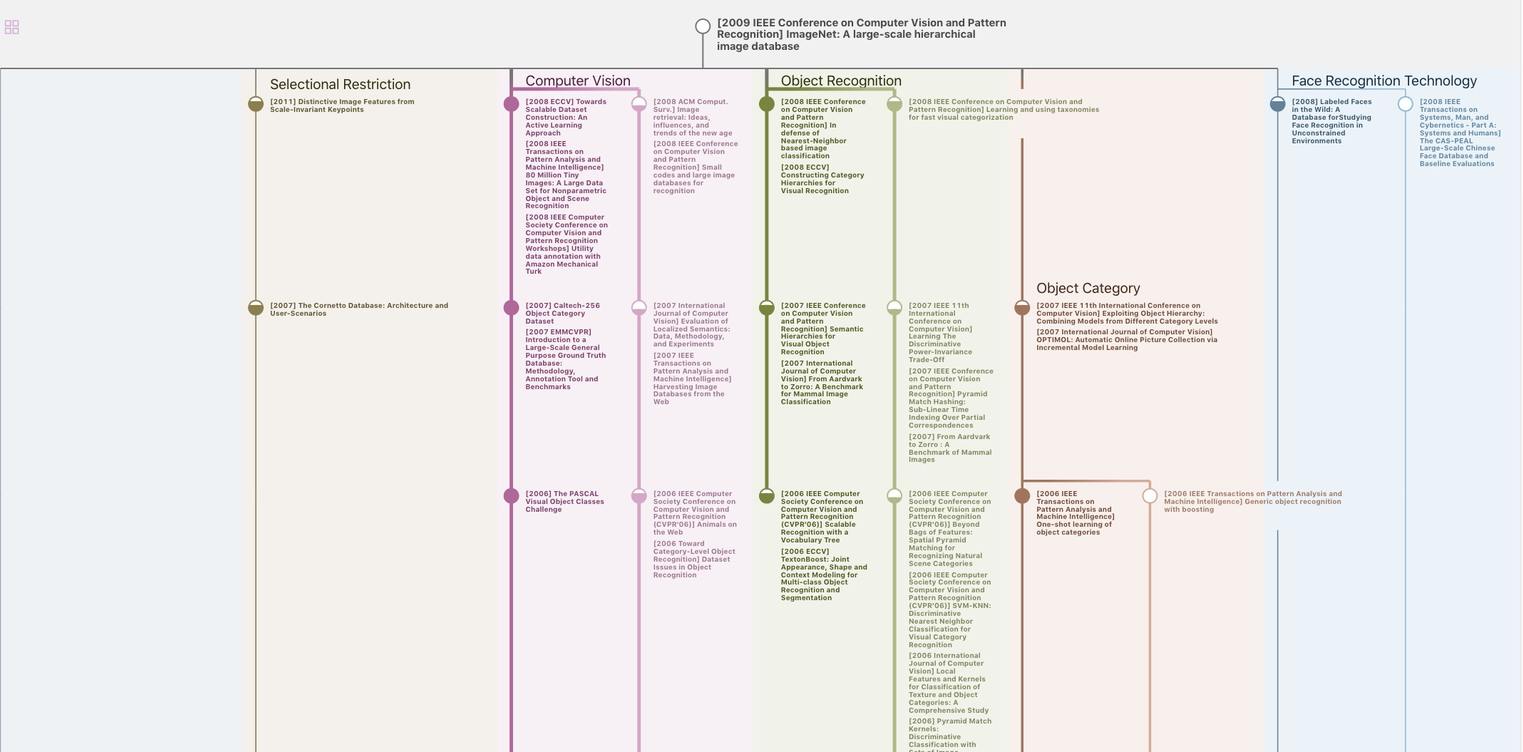
生成溯源树,研究论文发展脉络
Chat Paper
正在生成论文摘要