Supersense tagging with inter-annotator disagreement.
LAW@ACL(2016)
摘要
Linguistic annotation underlies many successful approaches in Natural Language Processing (NLP), where the annotated corpora are used for training and evaluating supervised learners. The consistency of annotation limits the performance of supervised models, and thus a lot of effort is put into obtaining high-agreement annotated datasets. Recent research has shown that annotation disagreement is not random noise, but carries a systematic signal that can be used for improving the supervised learner. However, prior work was limited in scope, focusing only on part-of-speech tagging in a single language. In this paper we broaden the experiments to a semantic task (supersense tagging) using multiple languages. In particular, we analyse how systematic disagreement is for sense annotation, and we present a preliminary study of whether patterns of disagreements transfer across languages.
更多查看译文
AI 理解论文
溯源树
样例
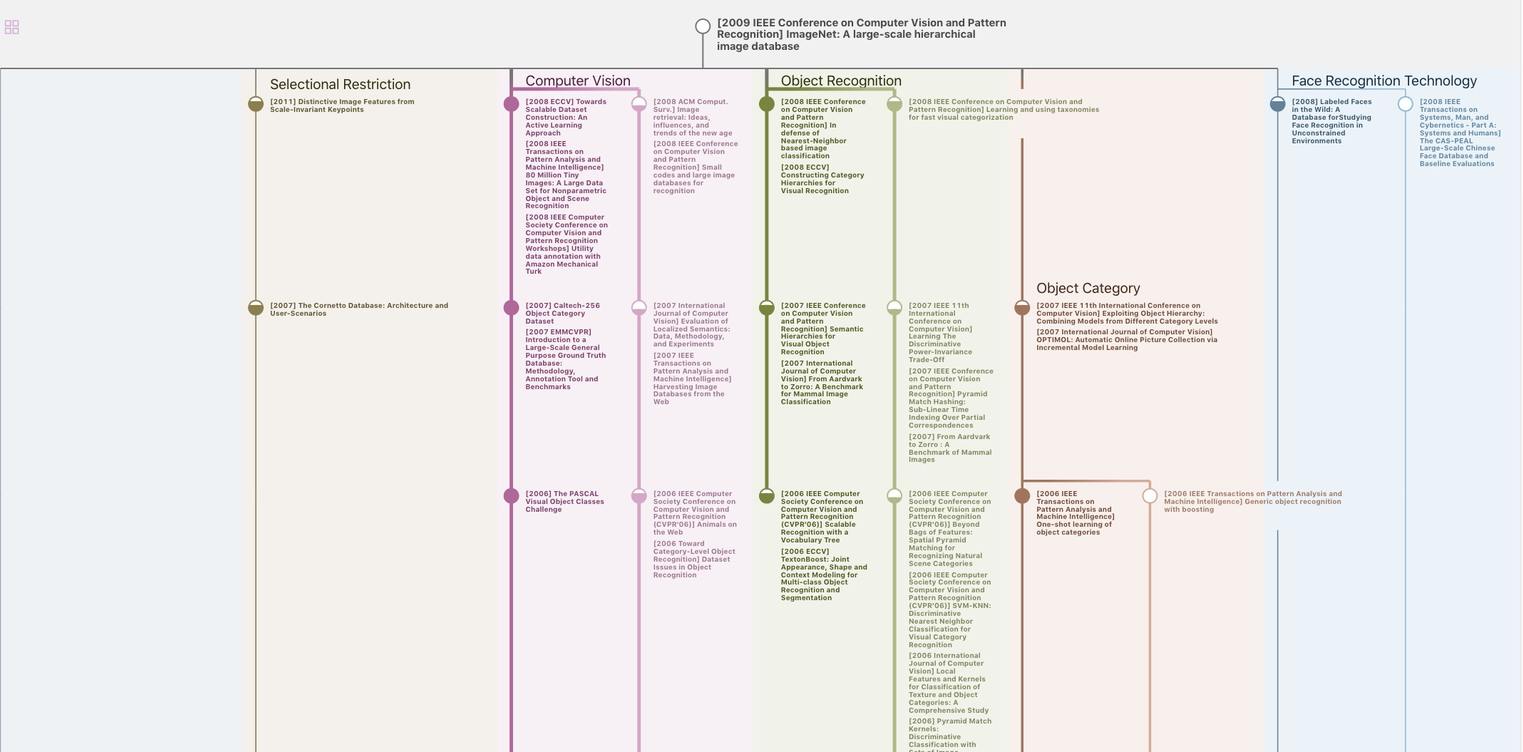
生成溯源树,研究论文发展脉络
Chat Paper
正在生成论文摘要