Unsupervised Identification of Clinically Relevant Clusters in Routine Imaging Data.
Medical Image Computing and Computer-Assisted Intervention – MICCAI 2016: 19th International Conference, Athens, Greece, October 17-21, 2016, Proceedings, Part I(2016)
摘要
A key question in learning from clinical routine imaging data is whether we can identify coherent patterns that re-occur across a population, and at the same time are linked to clinically relevant patient parameters. Here, we present a feature learning and clustering approach that groups 3D imaging data based on visual features at corresponding anatomical regions extracted from clinical routine imaging data without any supervision. On a set of 7812 routine lung computed tomography volumes, we show that the clustering results in a grouping linked to terms in radiology reports which were not used for clustering. We evaluate different visual features in light of their ability to identify groups of images with consistent reported findings.
更多查看译文
AI 理解论文
溯源树
样例
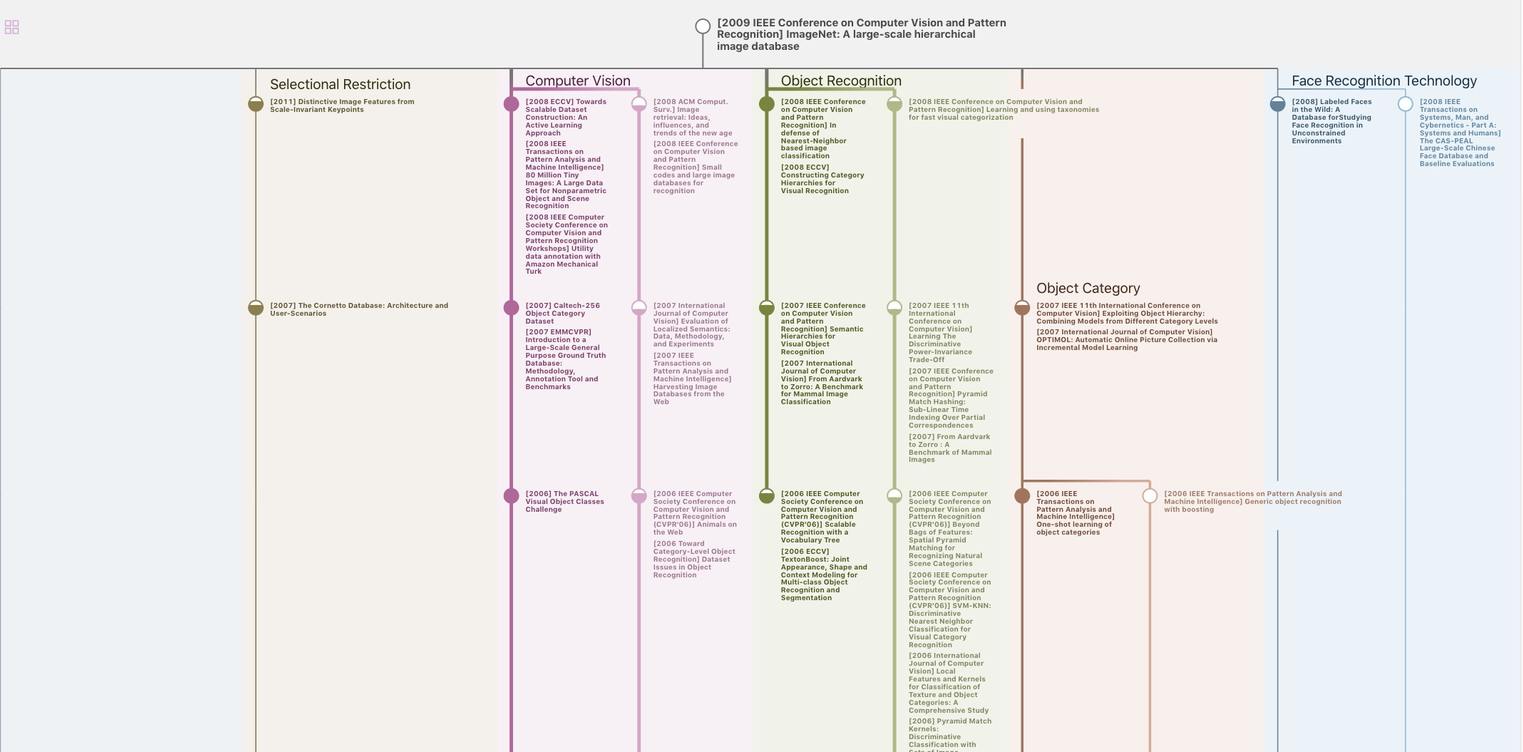
生成溯源树,研究论文发展脉络
Chat Paper
正在生成论文摘要