Learning for Graph-Based Sensorless Freehand 3D Ultrasound.
Lecture Notes in Computer Science(2016)
摘要
Sensorless freehand 3D ultrasound (US) uses speckle decorrelation to estimate small rigid motions between pairs of 2D images. Trajectory estimation combines these motion estimates to obtain the position each image relative to the first. This is prone to the accumulation of measurement bias. Whereas previous work concentrated on correcting biases at the source, this paper proposes to reduce error accumulation by carefully choosing the set of measurements used for trajectory estimation. An undirected graph is created with frames as vertices and motion measurements as edges. Using constrained shortest paths in the graph, random trajectories are generated and averaged to obtain trajectory estimate and uncertainty. To improve accuracy, a Gaussian process regressor is trained on tracked US sequences to predict systematic motion measurement error, which is then used to weigh the edges of the graph. Results on speckle phantom imagery show significantly improved trajectory estimates in comparison with the state-of-the-art, promising accurate volumetric reconstruction.
更多查看译文
关键词
Freehand 3D ultrasound,Speckle decorrelation,Sensorless reconstruction,Measurement selection,Gaussian process regression
AI 理解论文
溯源树
样例
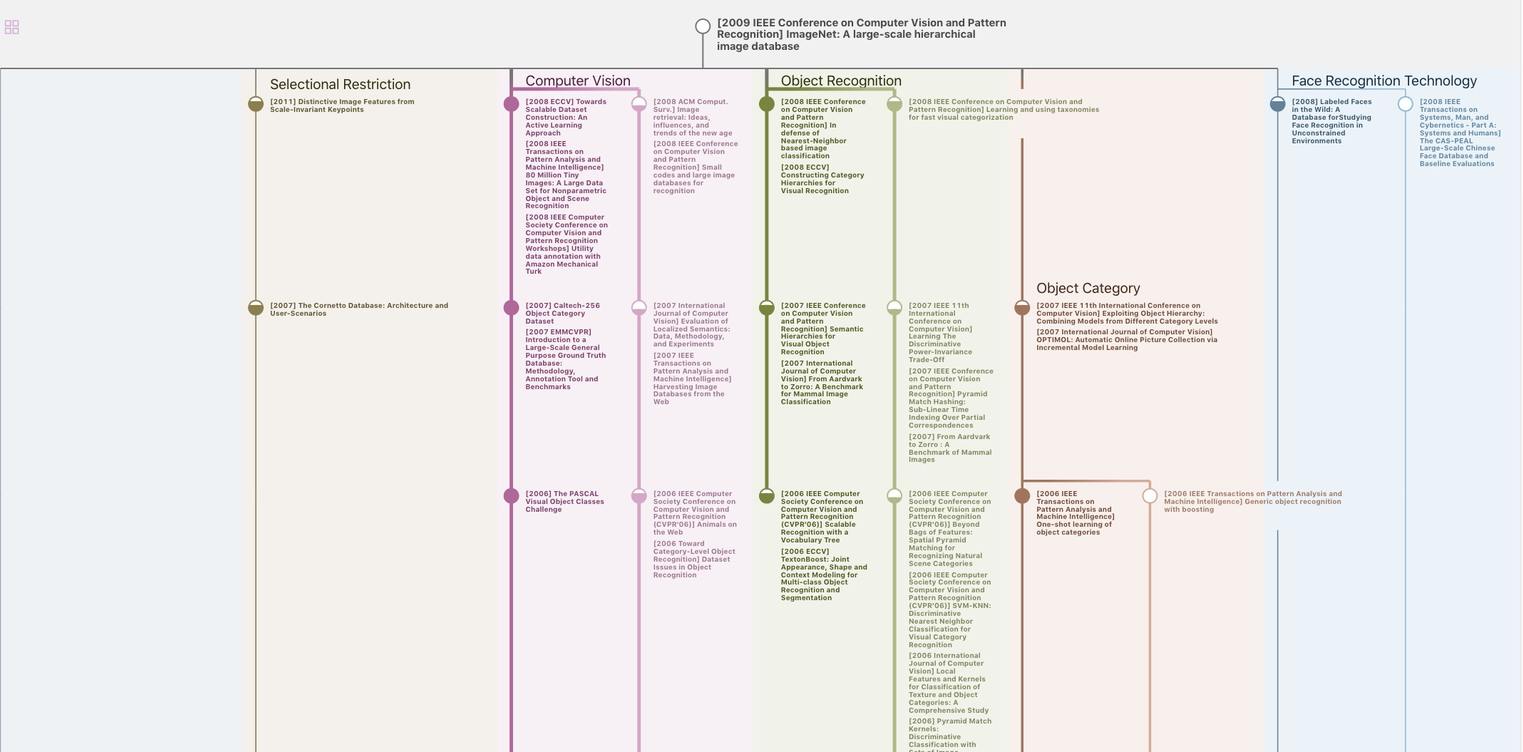
生成溯源树,研究论文发展脉络
Chat Paper
正在生成论文摘要