Stochastic CoSaMP: Randomizing Greedy Pursuit for Sparse Signal Recovery.
ECML/PKDD(2016)
摘要
In this paper, we formulate the K-sparse compressed signal recovery problem with the $$L_0$$ norm within a Stochastic Local Search SLS framework. Using this randomized framework, we generalize the popular sparse recovery algorithm CoSaMP, creating Stochastic CoSaMP StoCoSaMP. Interestingly, our deterministic worst case analysis shows that under the Restricted Isometric Property RIP, even a purely random version of StoCoSaMP is guaranteed to recover a notion of strong components of a sparse signal, thereby leading to support convergence. Empirically, we find that StoCoSaMP outperforms CoSaMP, both in terms of signal recoverability and computational cost, on different problems with upï¾źto 1 million dimensions. Further, StoCoSaMP outperforms several other popular recovery algorithms, including StoGradMP and StoIHT, on large real-world gene-expression datasets.
更多查看译文
AI 理解论文
溯源树
样例
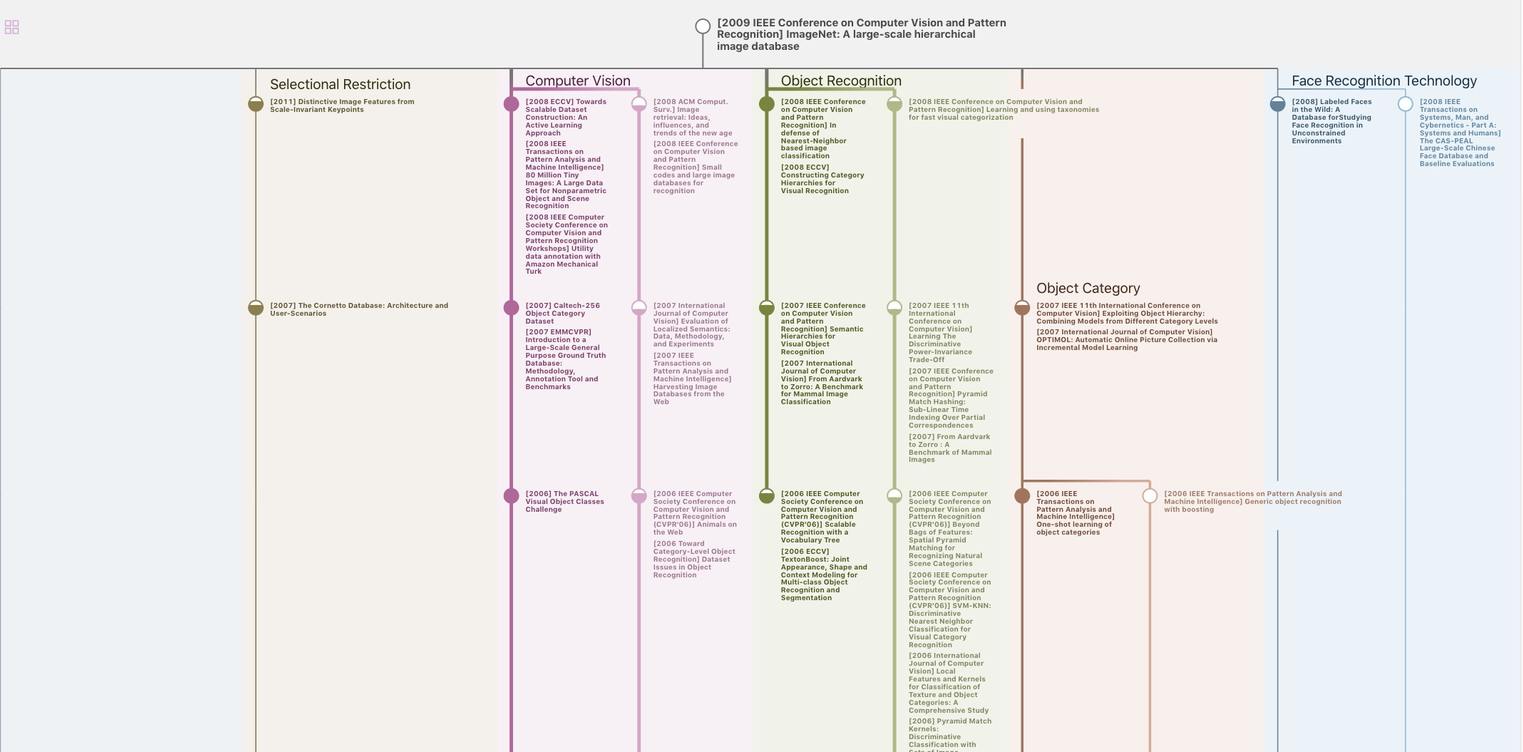
生成溯源树,研究论文发展脉络
Chat Paper
正在生成论文摘要