Learning Cascaded Influence Under Partial Monitoring
ASONAM '16: Advances in Social Networks Analysis and Mining 2016 Davis California August, 2016(2016)
摘要
Social influence has attracted tremendous attention from both academic and industrial communities due to the rapid development of online social networks. While most research has been focused on the direct influence between peers, learning cascaded indirect influence has not been previously studied. In this paper, we formulate the concept of cascade indirect influence based on the Independent Cascade model and then propose a novel online learning algorithm for learning the cascaded influence in the partial monitoring setting. We propose two bandit algorithms E-EXP3 and RE-EXP3 to address this problem. We theoretically prove that E-EXP3 has a cumulative regret bound of O (root T) over T, the number of time stamps. We will also show that RE-EXP3, a relaxed version of E-EXP3, achieves a better performance in practice. We compare the proposed algorithms with three baseline methods on both synthetic and real networks (Weibo and AMiner). Our experimental results show that RE-EXP3 converges 100x faster than E-EXP3. Both of them significantly outperform the alternative methods in terms of normalized regret. Finally, we apply the learned cascaded influence to help behavior prediction and experiments show that our proposed algorithms can help achieve a significant improvement (10-15% by accuracy) for behavior prediction.
更多查看译文
关键词
cascaded influence learning,academic-and-industrial communities,online social network development,independent cascade model,online learning algorithm,bandit algorithms,E-EXP3,RE-EXP3,behavior prediction
AI 理解论文
溯源树
样例
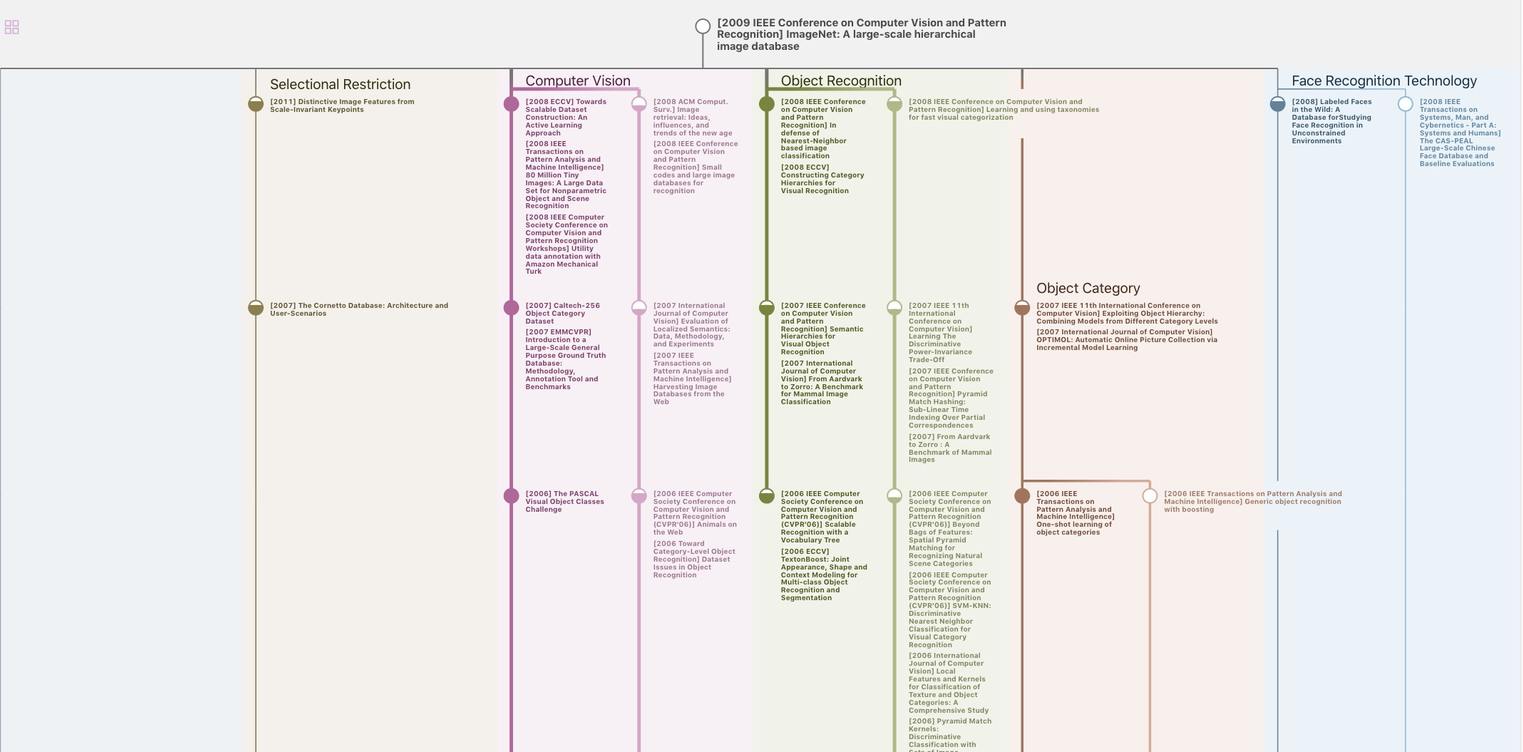
生成溯源树,研究论文发展脉络
Chat Paper
正在生成论文摘要