Node Label Matching Improves Classification Performance In Deep Belief Networks
2016 International Joint Conference on Neural Networks (IJCNN)(2016)
摘要
If output signals of artificial neural network classifiers are interpreted per node as class label predictors then partial knowledge encoded by the network during the learning procedure can be exploited in order to reassign which output node should represent each class label so that learning speed and final classification accuracy are improved. Our method for computing these reassignments is based on the maximum average correlation between actual node outputs and target labels over a small labeled validation dataset. Node Label Matching is an ancillary method for both supervised and unsupervised learning in artificial neural networks and we demonstrate its integration with Contrastive Divergence pre-training in Restricted Boltzmann Machines and Back Propagation fine-tuning in Deep Belief Networks. We introduce the Segmented Density Random Binary dataset and present empirical results of Node Label Matching on both our synthetic data and a subset of the MNIST benchmark.
更多查看译文
关键词
classification performance,deep belief networks,artificial neural network classifiers,class label predictors,partial knowledge,learning speed,classification accuracy,maximum average correlation,small labeled validation dataset,supervised learning,unsupervised learning,contrastive divergence pretraining,restricted Boltzmann machines,back propagation fine-tuning,segmented density random binary dataset,MNIST benchmark,node label matching
AI 理解论文
溯源树
样例
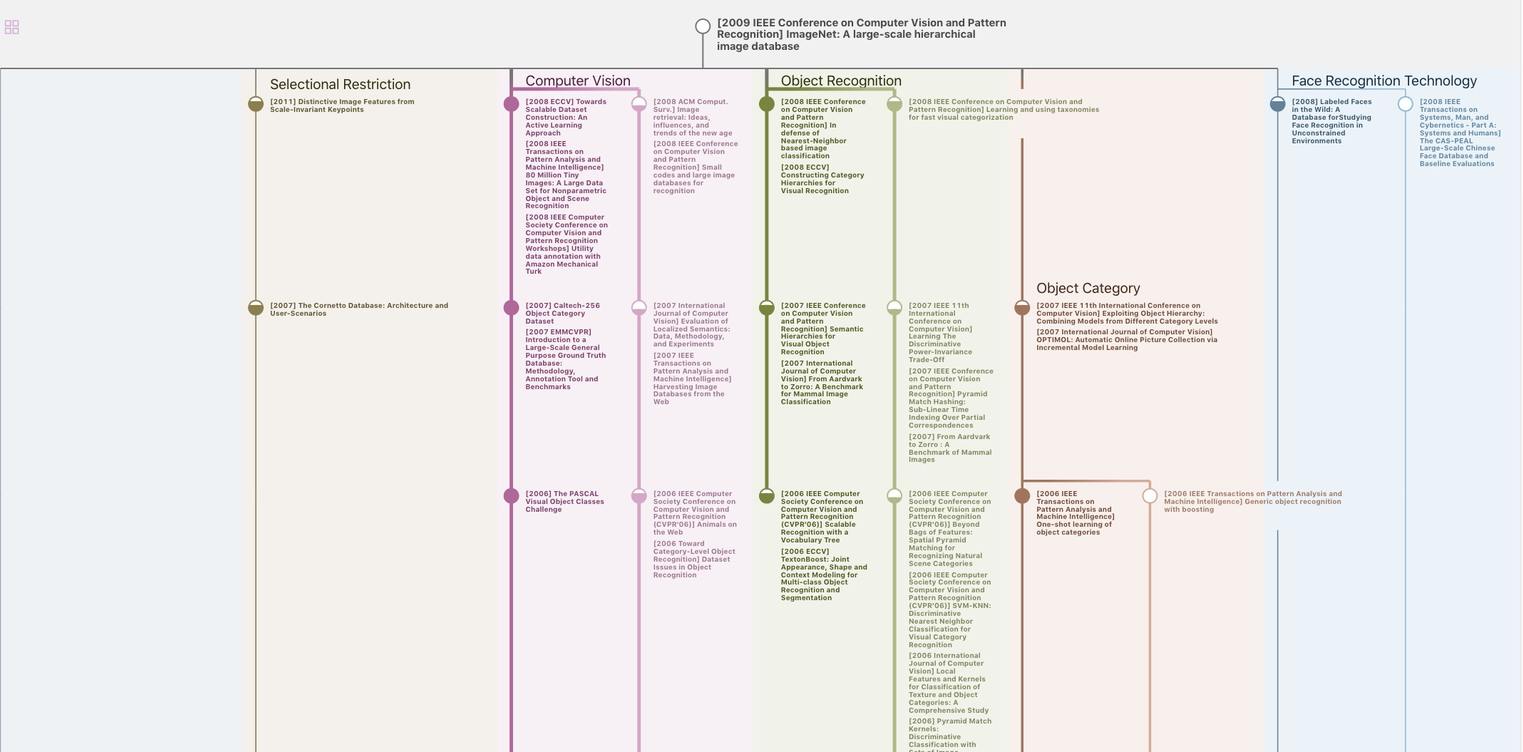
生成溯源树,研究论文发展脉络
Chat Paper
正在生成论文摘要