Advantage Based Value Iteration For Markov Decision Processes With Unknown Rewards
2016 International Joint Conference on Neural Networks (IJCNN)(2016)
摘要
This paper addresses approximating the optimal policy in Markov Decision Process with unknown rewards. The MDP is transformed into a Vector-Valued MDP (VVMDP). We introduce a new interactive algorithm ABVI, whose principle is using value iteration on VVMDPs and querying the user when necessary. This algorithm uses classification method to reduce the number of proposed queries. We integrate value iteration with querying the user to select appropriate backups. In this paper, our goal is to accelerate the value iteration algorithm and to reduce the number of queries.
更多查看译文
关键词
advantage based value iteration,Markov decision processes,unknown rewards,optimal policy approximation,vector-valued MDP,VVMDP,interactive algorithm,ABVI,classification method,reinforcement learning
AI 理解论文
溯源树
样例
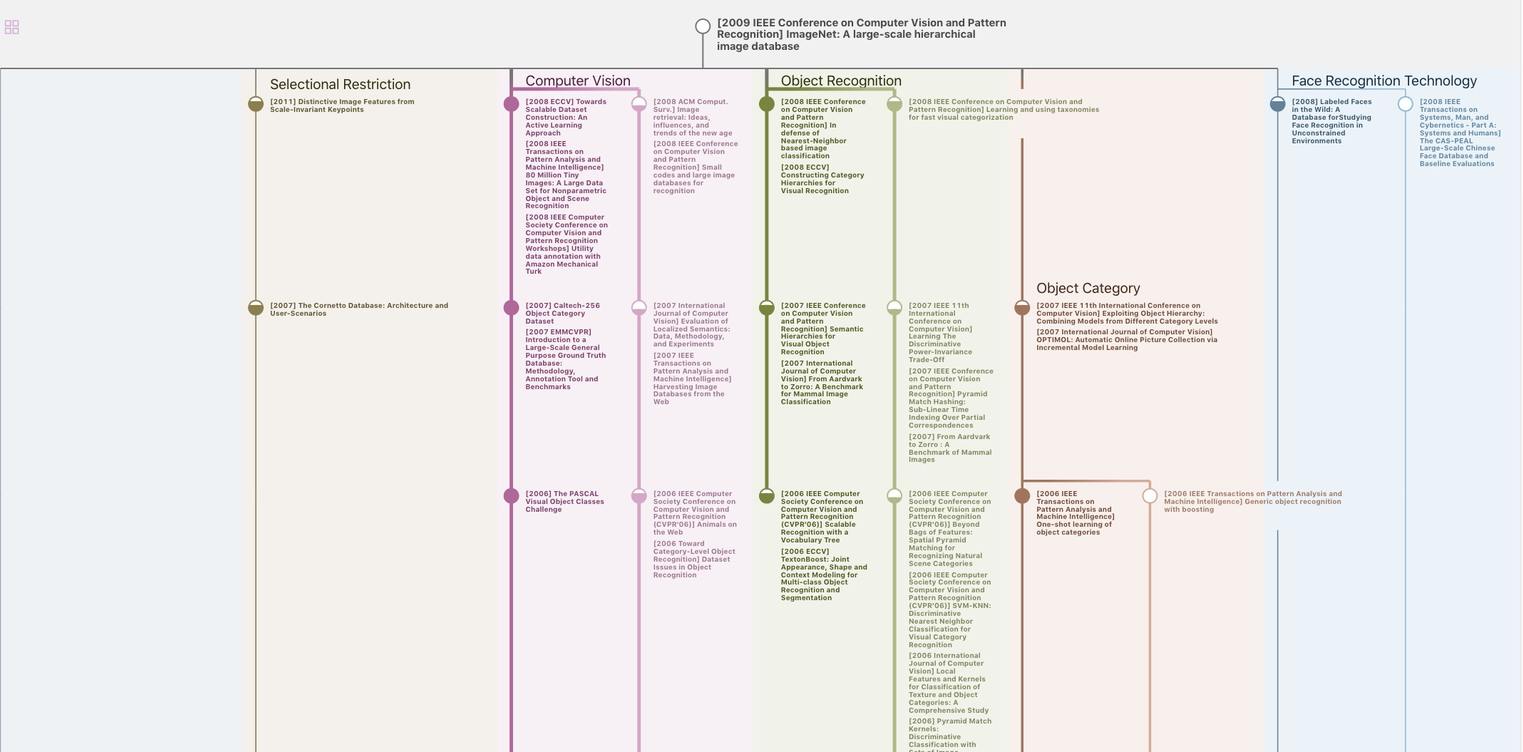
生成溯源树,研究论文发展脉络
Chat Paper
正在生成论文摘要