Non-Optimal Semi-Autonomous Agent Behavior Policy Recognition
PROCEEDINGS OF THE 8TH INTERNATIONAL JOINT CONFERENCE ON COMPUTATIONAL INTELLIGENCE, VOL 1: ECTA(2016)
摘要
The coordination between cooperative autonomous agents is mainly based on knowing or estimating the behavior policy of each others. Most approaches assume that agents estimate the policies of the others by considering the optimal ones. Unfortunately, this assumption is not valid when an external entity changes the behavior of a semi-autonomous agent in a non-optimal way. We face such problems when an operator is guiding or tele-operating a system where many factors can affect his behavior such as stress, hesitations, preferences, etc. In such situations the recognition of the other agent policies becomes harder than usual since considering all situations of hesitations or stress is not feasible.In this paper, we propose an approach able to recognize and predict future actions and behavior of such agents when they can follow any policy including non-optimal ones and different hesitations and preferences cases by using online learning techniques. The main idea of our approach is based on estimating, initially, the policy by the optimal one, then we update it according to the observed behavior to derive a new estimated policy. In this paper, we present three learning methods of updating policies, show their stability and efficiency and compare them with existing approaches.
更多查看译文
关键词
Behavior, Recognition, MDP, Reinforcement Learning
AI 理解论文
溯源树
样例
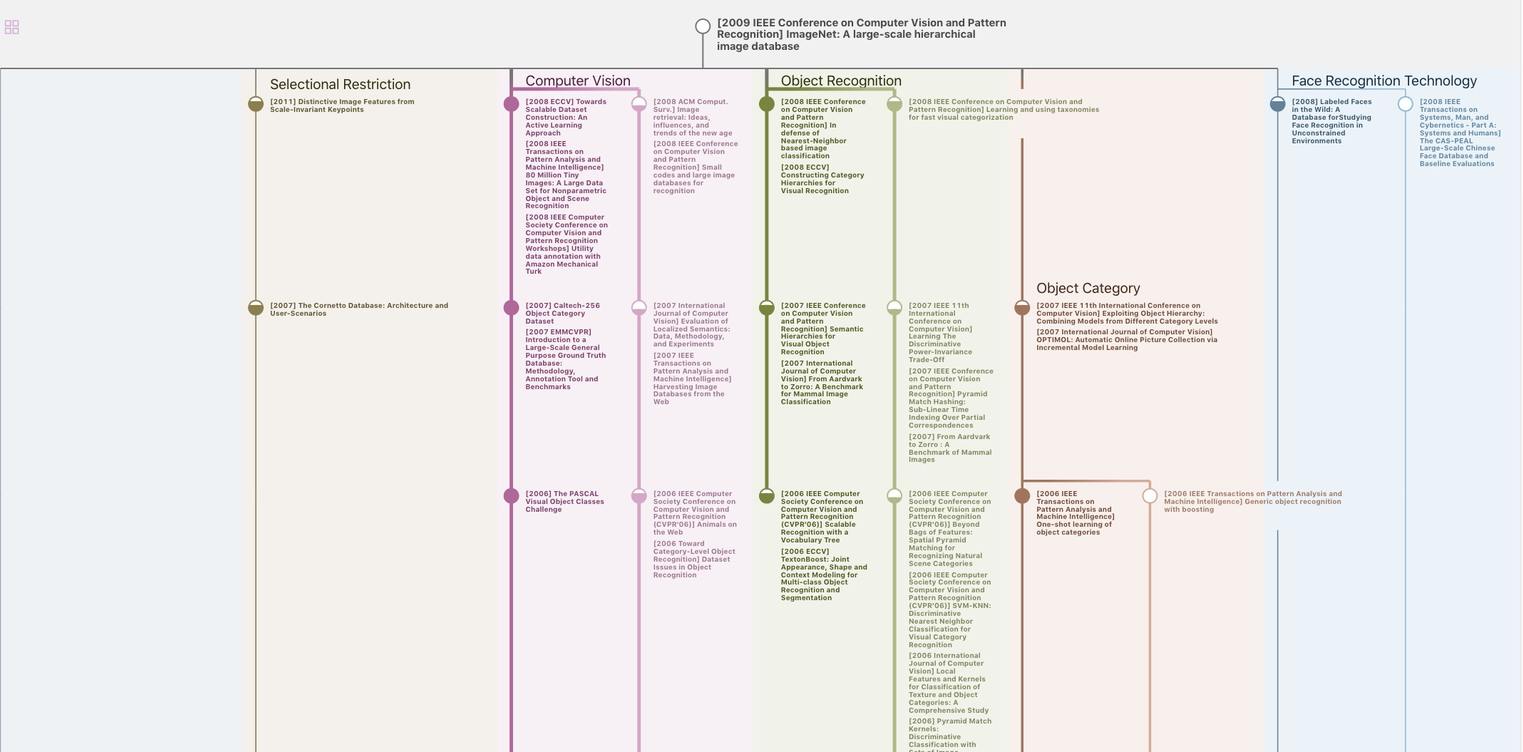
生成溯源树,研究论文发展脉络
Chat Paper
正在生成论文摘要