Holonet: Towards Robust Emotion Recognition In The Wild
ICMI-MLMI(2016)
摘要
In this paper, we present HoloNet, a well-designed Convolutional Neural Network (CNN) architecture regarding our submissions to the video based sub-challenge of the Emotion Recognition in the Wild (EmotiW) 2016 challenge. In contrast to previous related methods that usually adopt relatively simple and shallow neural network architectures to address emotion recognition task, our HoloNet has three critical considerations in network design. (1) To reduce redundant filters and enhance the non-saturated nonlinearity in the lower convolutional layers, we use a modified Concatenated Rectified Linear Unit (CReLU) instead of ReLU. (2) To enjoy the accuracy gain from considerably increased network depth and maintain efficiency, we combine residual structure and CReLU to construct the middle layers. (3) To broaden network width and introduce multi-scale feature extraction property, the topper layers are designed as a variant of inception-residual structure. The main benefit of grouping these modules into the HoloNet is that both negative and positive phase information implicitly contained in the input data can flow over it in multiple paths, thus deep multi-scale features explicitly capturing emotion variation can be well extracted from multi-path sibling layers, and then can be further concatenated for robust recognition. We obtain competitive results in this year's video based emotion recognition sub-challenge using an ensemble of two HoloNet models trained with given data only. Specifically, we obtain a mean recognition rate of 57.84%, outperforming the baseline accuracy with an absolute margin of 17.37%, and yielding 4.04% absolute accuracy gain compared to the result of last year's winner team. Meanwhile, our method runs with a speed of several thousands of frames per second on a GPU, thus it is well applicable to real-time scenarios.
更多查看译文
关键词
Emotion Recognition,EmotiW 2016 Challenge,Deep Learning,Convolutional Neural Networks
AI 理解论文
溯源树
样例
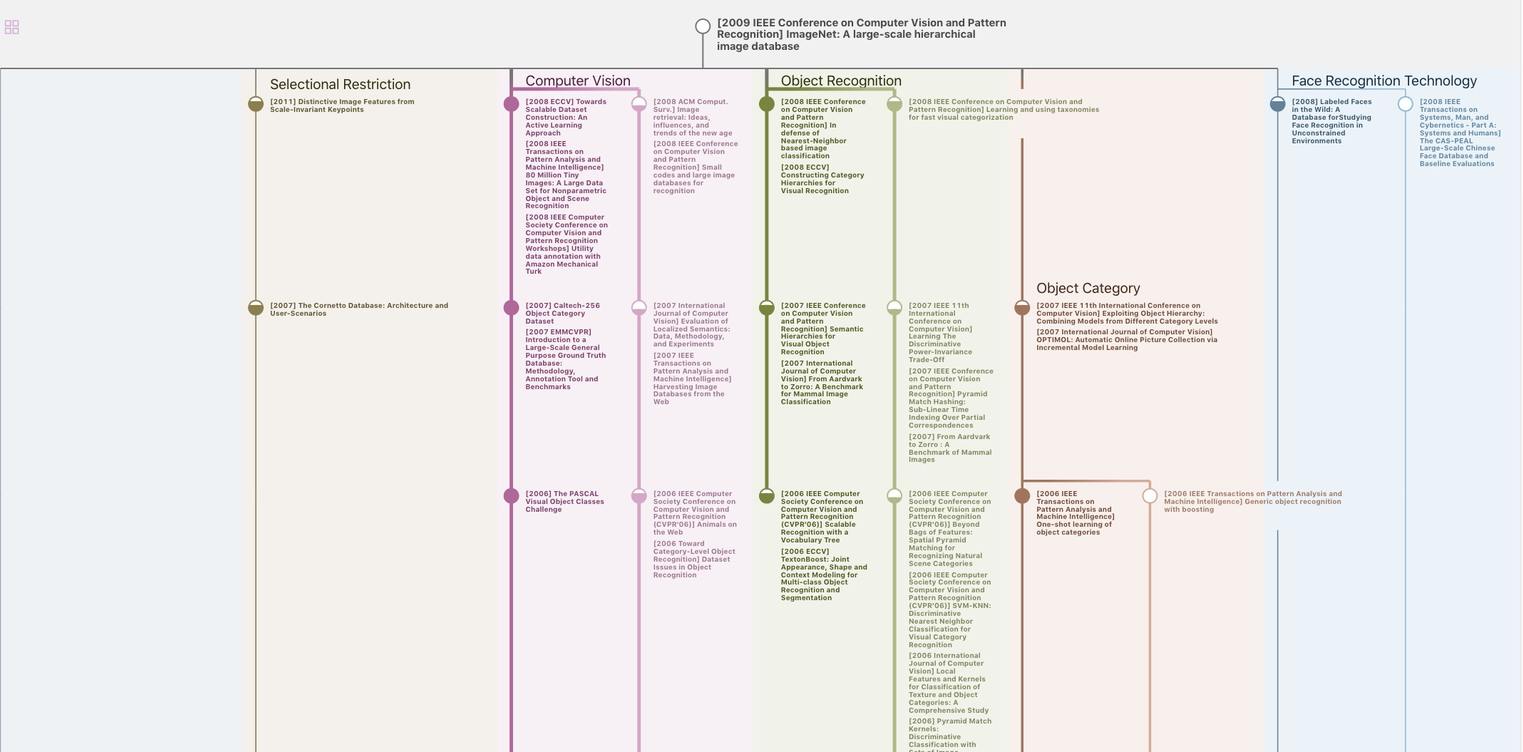
生成溯源树,研究论文发展脉络
Chat Paper
正在生成论文摘要