An Online-Updating Approach on Task Recommendation in Crowdsourcing Systems.
Lecture Notes in Computer Science(2016)
摘要
In crowdsourcing systems, task recommendation can help workers to find their right tasks faster as well as help requesters to receive good quality output quicker. A number of previous works adopted active learning for task recommendation in crowdsourcing systems to achieve certain accuracy with a very low cost. However, the model updating methods in previous works are not suitable for real-world applications. In our paper, we propose a generic online-updating method for learning a factor analysis model, ActivePMF on TaskRec (Probabilistic Matrix Factorization with Active Learning on Task Recommendation Framework), for crowdsourcing systems. The larger the profile of a worker (or task) is, the less important is retraining its profile on each new work done. In case of the worker (or task) having large profile, our algorithm only retrains the whole feature vector of the worker (or task) and keeps all other entries in the matrix fixed. Besides, our algorithm runs batch update to further improve the performance. Experiment results show that our online-updating approach is accurate in approximating to a full retrain while the average runtime of model update for each work done is reduced by more than 90% (from a few minutes to several seconds).
更多查看译文
关键词
Crowdsourcing System, Recommendation Task, Full Retraining, Probabilistic Matrix Factorization (PMF), Batch Update
AI 理解论文
溯源树
样例
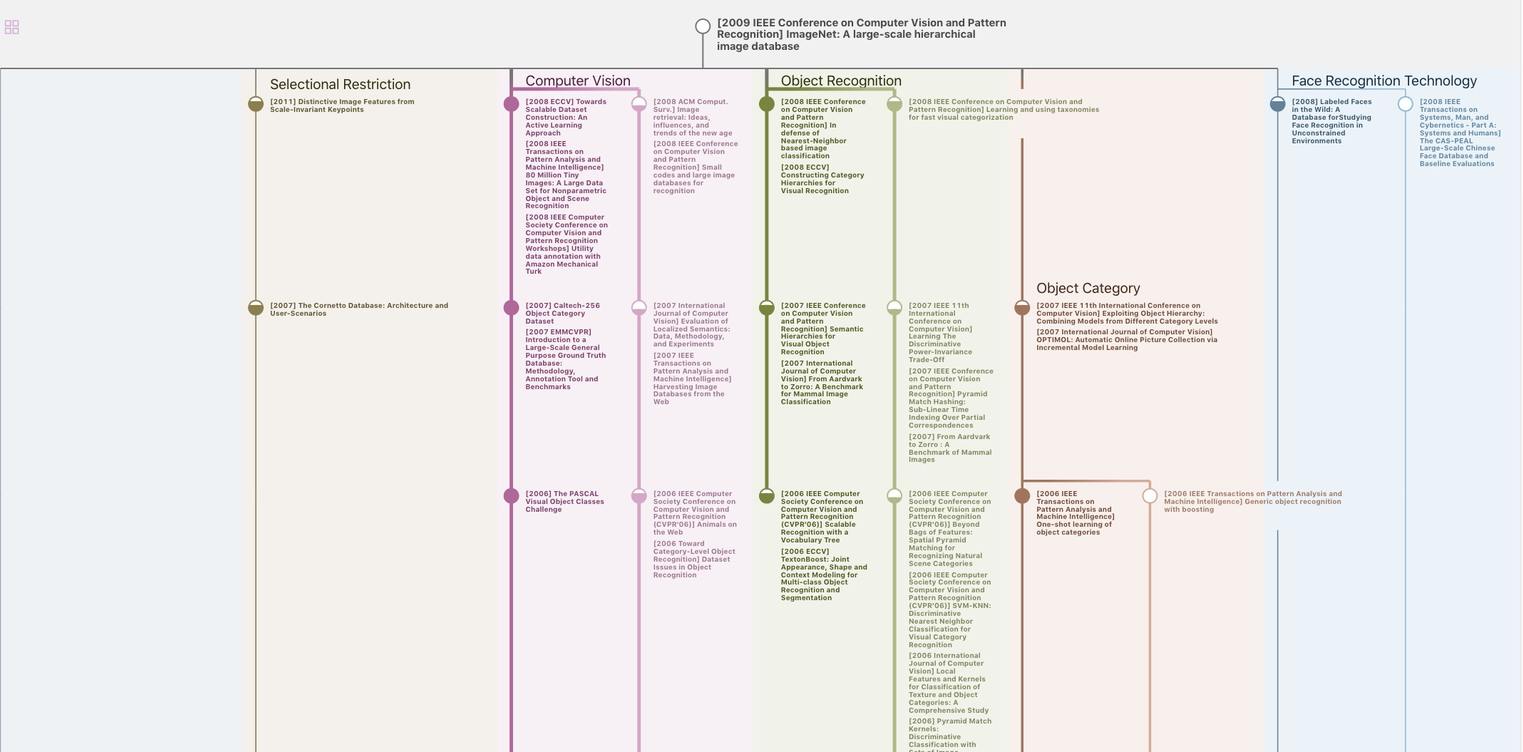
生成溯源树,研究论文发展脉络
Chat Paper
正在生成论文摘要