A Novel Manifold Regularized Online Semi-supervised Learning Algorithm.
Lecture Notes in Computer Science(2016)
摘要
In this paper, we propose a novel manifold regularized online semi-supervised learning ((OSL)-L-2) model in an Reproducing Kernel Hilbert Space (RK-HS). The proposed algorithm, named Model-Based Online Manifold Regularization (MOMR), is derived by solving a constrained optimization problem, which is different from the stochastic gradient algorithm used for solving the online version of the primal problem of Laplacian support vector machine (LapSVM). Taking advantage of the convex property of the proposed model, an exact solution can be obtained iteratively by solving its Lagrange dual problem. Furthermore, a buffering strategy is introduced to improve the computational efficiency of the algorithm. Finally, the proposed algorithm is experimentally shown to have a comparable performance to the standard batch manifold regularization algorithm.
更多查看译文
关键词
Manifold regularization,Online semi-supervised learning,Lagrange dual problem
AI 理解论文
溯源树
样例
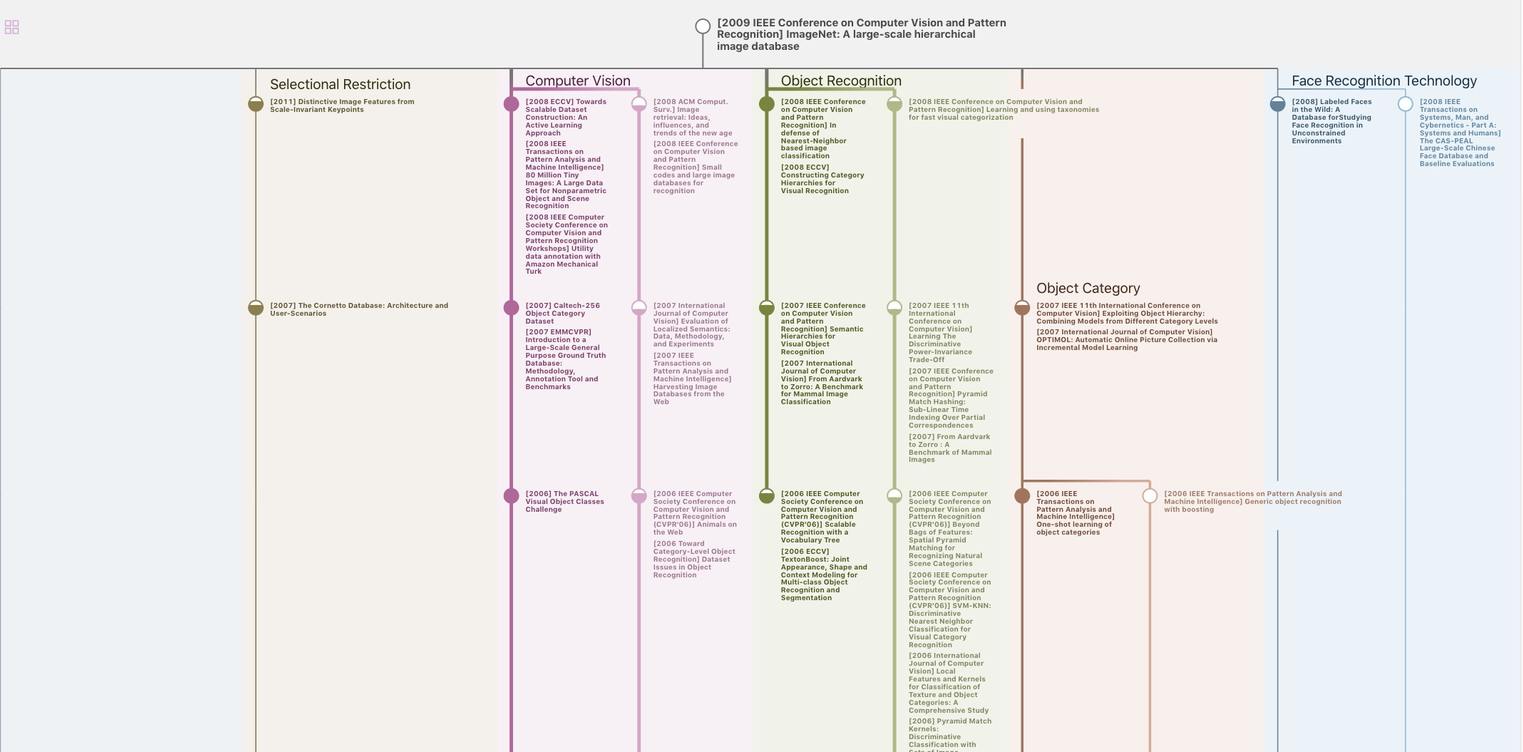
生成溯源树,研究论文发展脉络
Chat Paper
正在生成论文摘要