On Local Expert Discovery Via Geo-Located Crowds, Queries, And Candidates
ACM Transactions on Spatial Algorithms and Systems(2016)
摘要
Local experts are critical for many location-sensitive information needs, and yet there is a research gap in our understanding of the factors impacting who is recognized as a local expert and in methods for discovering local experts. Hence, in this article, we explore a geo-spatial learning-to-rank framework for identifying local experts. Three of the key features of the proposed approach are: (i) a learning-based framework for integratingmultiple user-based, content-based, list-based, and crowd-based factors impacting local expertise that leverages the fine-grained GPS coordinates of millions of social media users; (ii) a location-sensitive randomwalk that propagates crowd knowledge of a candidate's expertise; and (iii) a comprehensive controlled study over AMT-labeled local experts on eight topics and in four cities. We find significant improvements of local expert finding versus two state-of-the-art alternatives, as well as evidence for the generalizability of local expert ranking models to new topics and new locations.
更多查看译文
关键词
Local expert discovery,Twitter list,geo-spatial
AI 理解论文
溯源树
样例
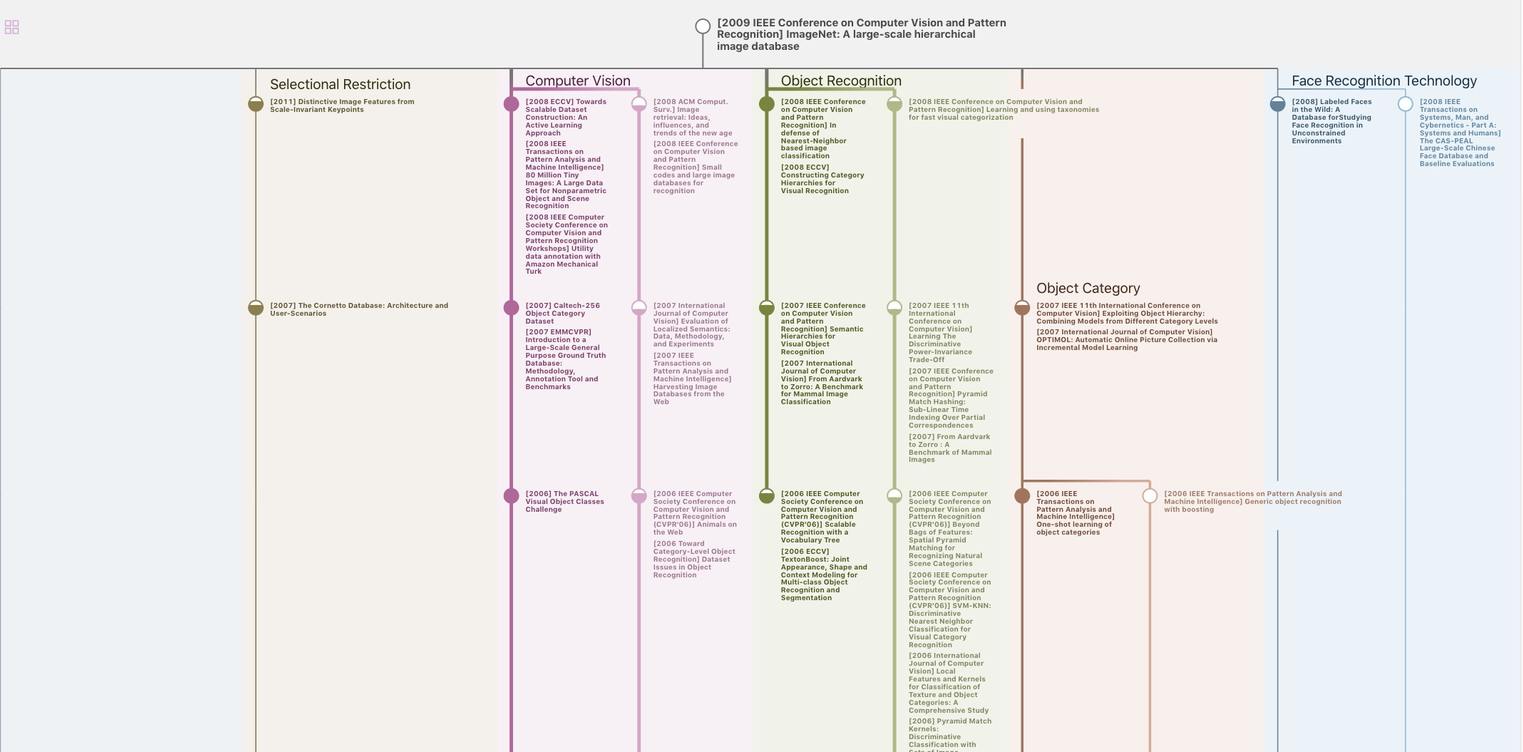
生成溯源树,研究论文发展脉络
Chat Paper
正在生成论文摘要