A Reverberation-Time-Aware Approach to Speech Dereverberation Based on Deep Neural Networks.
IEEE/ACM Trans. Audio, Speech & Language Processing(2017)
摘要
A reverberation-time-aware deep-neural-network DNN-based speech dereverberation framework is proposed to handle a wide range of reverberation times. There are three key steps in designing a robust system. First, in contrast to sigmoid activation and min-max normalization in state-of-the-art algorithms, a linear activation function at the output layer and global mean-variance normalization of target features are adopted to learn the complicated nonlinear mapping function from reverberant to anechoic speech and to improve the restoration of the low-frequency and intermediate-frequency contents. Next, two key design parameters, namely, frame shift size in speech framing and acoustic context window size at the DNN input, are investigated to show that RT60-dependent parameters are needed in the DNN training stage in order to optimize the system performance in diverse reverberant environments. Finally, the reverberation time is estimated to select the proper frame shift and context window sizes for feature extraction before feeding the log-power spectrum features to the trained DNNs for speech dereverberation. Our experimental results indicate that the proposed framework outperforms the conventional DNNs without taking the reverberation time into account, while achieving a performance only slightly worse than the oracle cases with known reverberation times even for extremely weak and severe reverberant conditions. It also generalizes well to unseen room sizes, loudspeaker and microphone positions, and recorded room impulse responses.
更多查看译文
关键词
Speech,Reverberation,Training,Context,Feature extraction,Speech processing
AI 理解论文
溯源树
样例
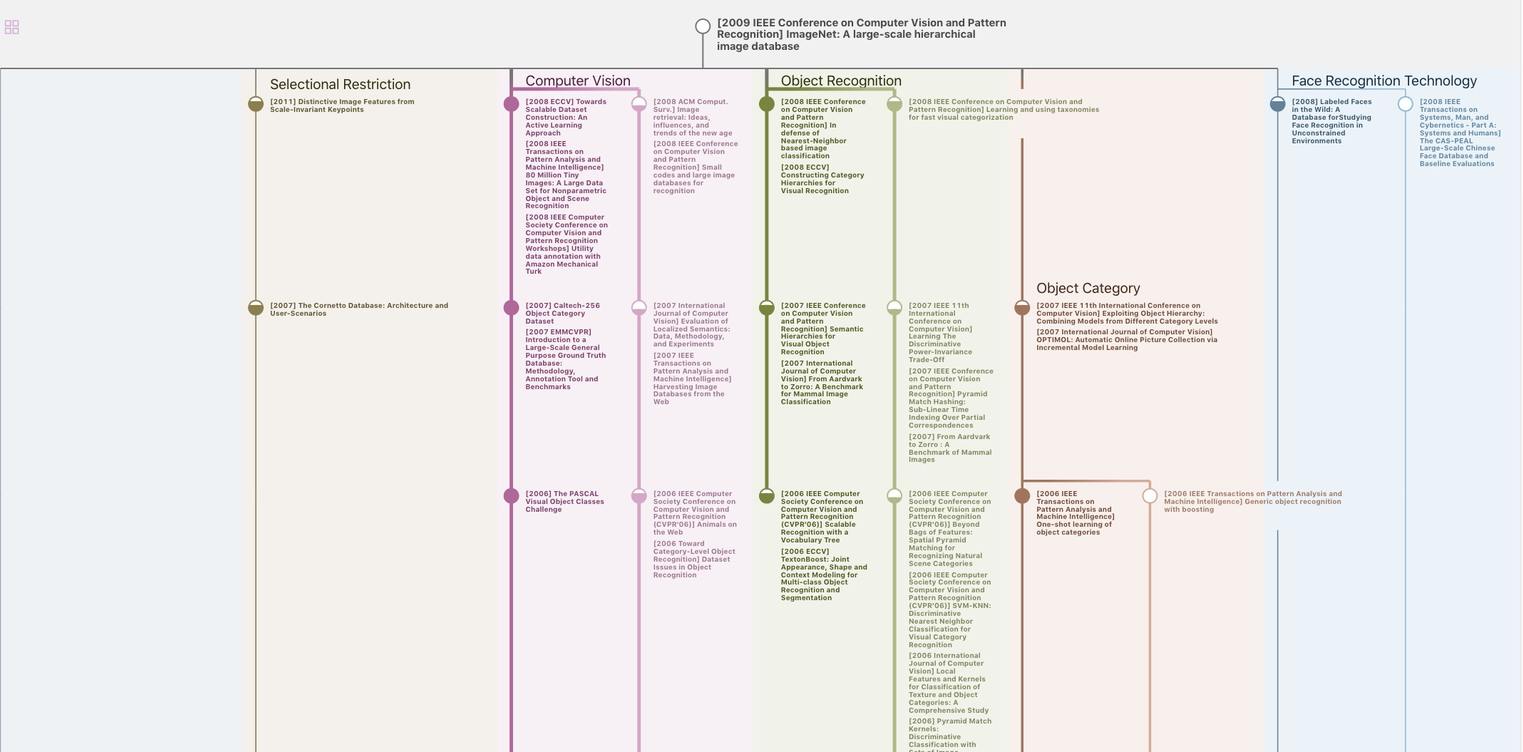
生成溯源树,研究论文发展脉络
Chat Paper
正在生成论文摘要