Semi-supervised community detection based on non-negative matrix factorization with node popularity.
Inf. Sci.(2017)
摘要
Based on the ideas of graph regularization, we present a semi-supervised and NMF-based model to utilize the prior information of networks.By introducing the parameters of node popularities, we propose a refined PSSNMF model, which is particularly suitable for networks with large degree heterogeneity and unbalanced community structure.The prior information with node popularity being introduced into the model is more effective than being directly encoded into the adjacent matrix. A plethora of exhaustive studies have proved that the community detection merely based on topological information often leads to relatively low accuracy. Several approaches aim to achieve performance improvement by utilizing the background information. But they ignore the effect of node degrees on the availability of prior information. In this paper, by combining the idea of graph regularization with the pairwise constraints, we present a semi-supervised non-negative matrix factorization (SSNMF) model for community detection. And then, to alleviate the influence of the heterogeneity of node degrees and community sizes, we propose an improved SSNMF model by introducing the node popularity, namely PSSNMF, which helps to utilize the prior information more effectively. At last, the extensive experiments on both artificial and real-world networks show that the proposed method improves, as expected, the accuracy of community detection, especially on networks with large degree heterogeneity and unbalanced community structure.
更多查看译文
关键词
Community detection,Non-negative matrix factorization,Semi-supervised learning,Node popularity
AI 理解论文
溯源树
样例
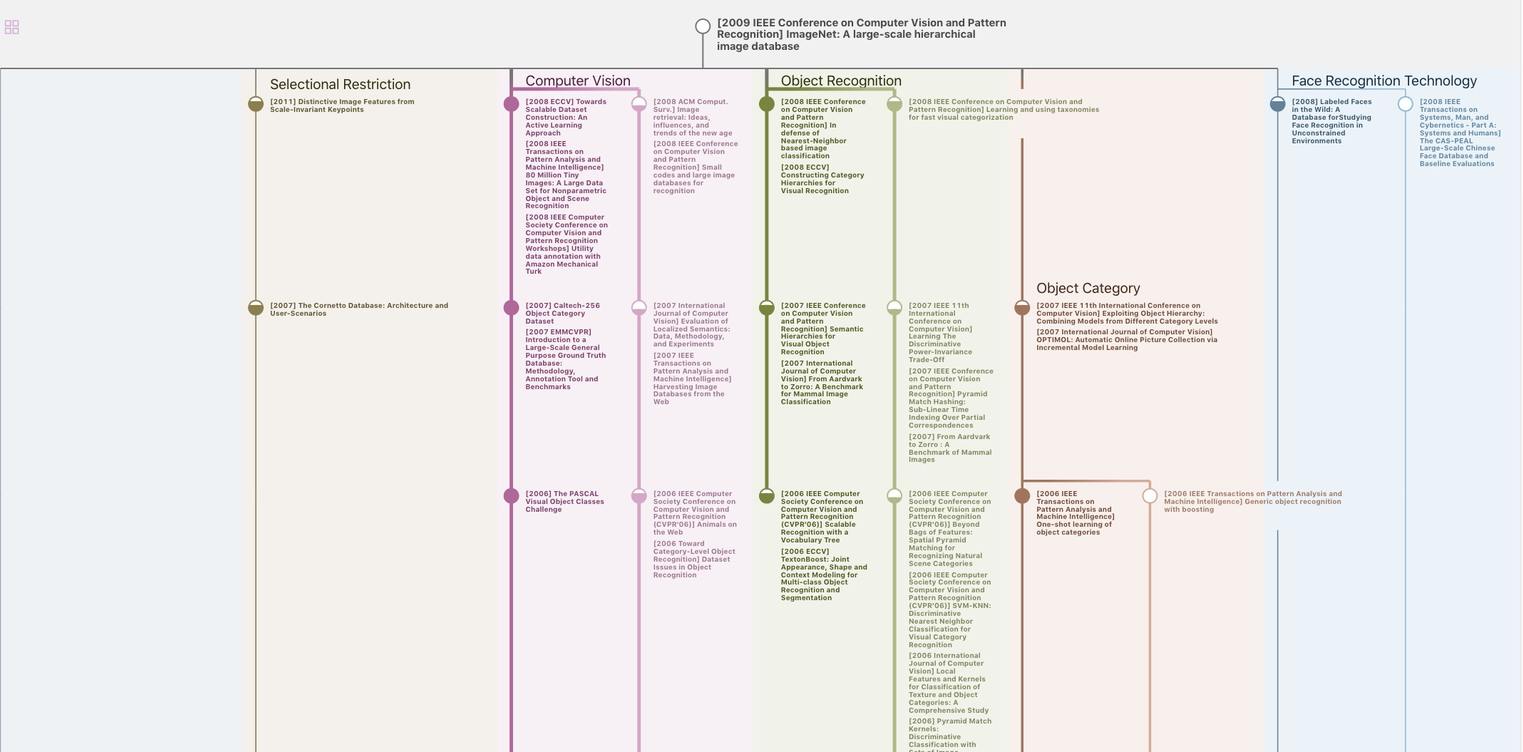
生成溯源树,研究论文发展脉络
Chat Paper
正在生成论文摘要