Structure Feature Learning Method For Incomplete Data
INTERNATIONAL JOURNAL OF PATTERN RECOGNITION AND ARTIFICIAL INTELLIGENCE(2016)
摘要
Learning with incomplete data remains challenging in many real-world applications especially when the data is high-dimensional and dynamic. Many imputation-based algorithms have been proposed to handle with incomplete data, where these algorithms use statistics of the historical information to remedy the missing parts. However, these methods merely use the structural information existing in the data, which are very helpful for sharing between the complete entries and the missing ones. For example, in traffic system, some group information and temporal smoothness exist in the data structure. In this paper, we propose to incorporate these structural information and develop structural feature leaning method for learning with incomplete data (SFLIC). The SFLIC model adopt a fused Lasso based regularizer and a group Lasso style regularizer to enlarge the data sharing along both the temporal smoothness level and the feature group level to fill the gap where the data entries are missing. The proposed SFLIC model is a nonsmooth function according to the model parameters, and we adopt the smoothing proximal gradient (SPG) method to seek for an efficient solution. We evaluate our model on both synthetic and real-world highway traffic datasets. Experimental results show that our method outperforms the state-of-the-art methods.
更多查看译文
关键词
Intelligent transportation system, incomplete data, structure feature learning
AI 理解论文
溯源树
样例
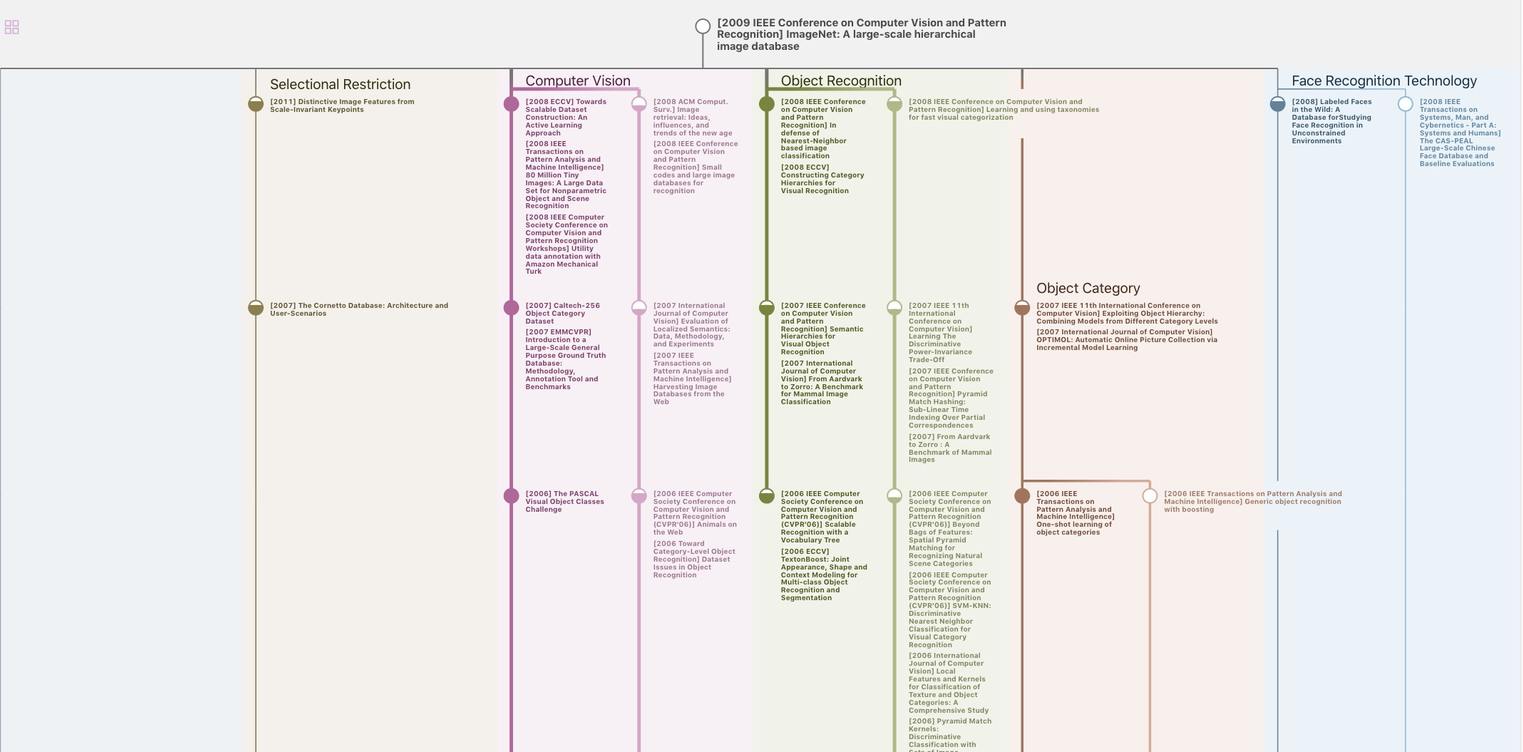
生成溯源树,研究论文发展脉络
Chat Paper
正在生成论文摘要