Object-Centric Representation Learning From Unlabeled Videos
COMPUTER VISION - ACCV 2016, PT V(2016)
摘要
Supervised (pre-)training currently yields state-of-the-art performance for representation learning for visual recognition, yet it comes at the cost of (1) intensive manual annotations and (2) an inherent restriction in the scope of data relevant for learning. In this work, we explore unsupervised feature learning from unlabeled video. We introduce a novel object-centric approach to temporal coherence that encourages similar representations to be learned for object-like regions segmented from nearby frames. Our framework relies on a Siamese-triplet network to train a deep convolutional neural network (CNN) representation. Compared to existing temporal coherence methods, our idea has the advantage of lightweight preprocessing of the unlabeled video (no tracking required) while still being able to extract object-level regions from which to learn invariances. Furthermore, as we show in results on several standard datasets, our method typically achieves substantial accuracy gains over competing unsupervised methods for image classification and retrieval tasks.
更多查看译文
关键词
Video Frame, Convolutional Neural Network, Temporal Coherence, Deep Neural Network, Region Proposal
AI 理解论文
溯源树
样例
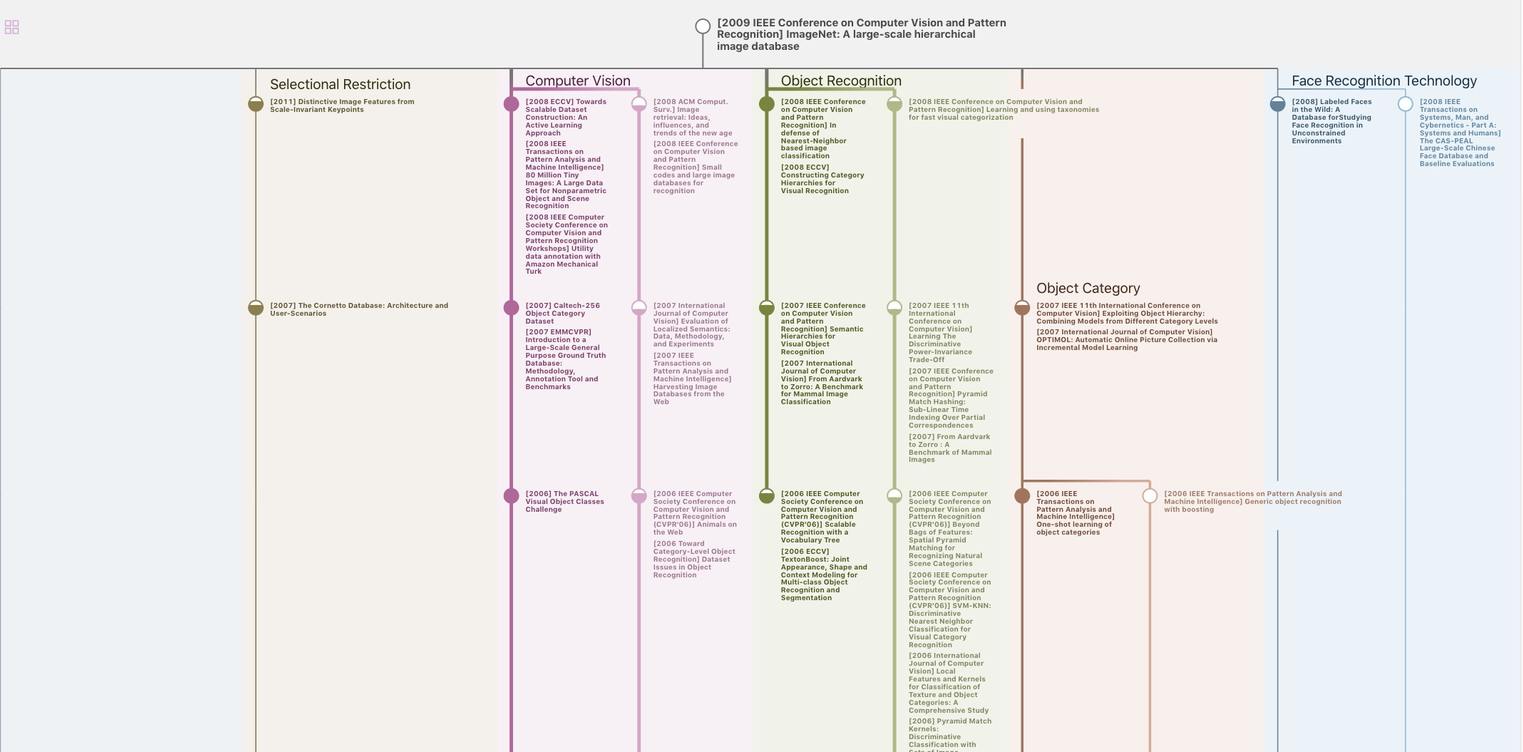
生成溯源树,研究论文发展脉络
Chat Paper
正在生成论文摘要