Commonly Uncommon: Semantic Sparsity in Situation Recognition
2017 IEEE Conference on Computer Vision and Pattern Recognition (CVPR)(2016)
摘要
Semantic sparsity is a common challenge in structured visual classification problems; when the output space is complex, the vast majority of the possible predictions are rarely, if ever, seen in the training set. This paper studies semantic sparsity in situation recognition, the task of producing structured summaries of what is happening in images, including activities, objects and the roles objects play within the activity. For this problem, we find empirically that most object-role combinations are rare, and current state-of-the-art models significantly underperform in this sparse data regime. We avoid many such errors by (1) introducing a novel tensor composition function that learns to share examples across role-noun combinations and (2) semantically augmenting our training data with automatically gathered examples of rarely observed outputs using web data. When integrated within a complete CRF-based structured prediction model, the tensor-based approach outperforms existing state of the art by a relative improvement of 2.11% and 4.40% on top-5 verb and noun-role accuracy, respectively. Adding 5 million images with our semantic augmentation techniques gives further relative improvements of 6.23% and 9.57% on top-5 verb and noun-role accuracy.
更多查看译文
关键词
situation recognition,structured visual classification problems,output space,training set,structured summaries,novel tensor composition function,training data,prediction model,top-5 verb,noun-role accuracy,semantic augmentation techniques,semantic sparsity
AI 理解论文
溯源树
样例
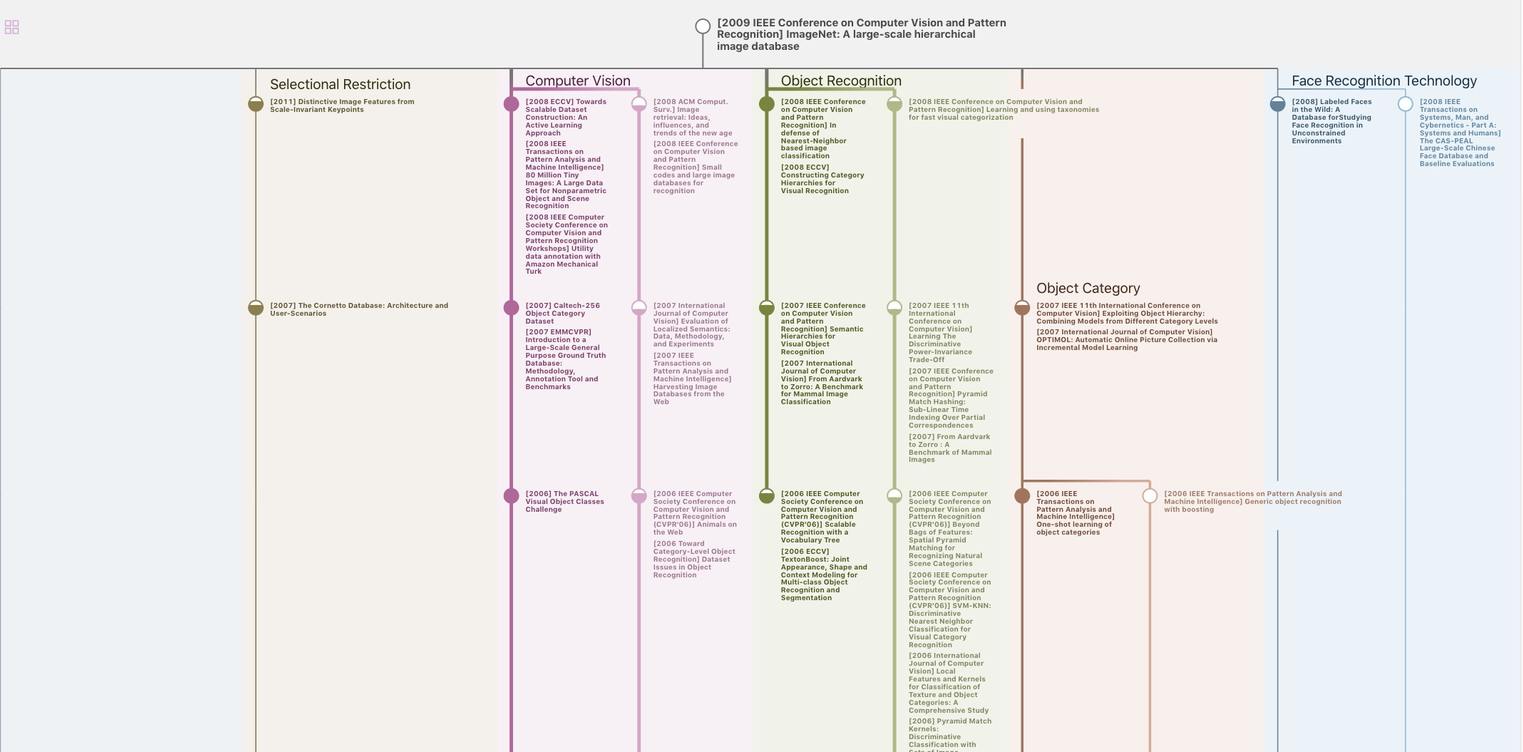
生成溯源树,研究论文发展脉络
Chat Paper
正在生成论文摘要