Optimizing Quantiles in Preference-based Markov Decision Processes
AAAI'17: Proceedings of the Thirty-First AAAI Conference on Artificial Intelligence(2016)
摘要
In the Markov decision process model, policies are usually evaluated by expected cumulative rewards. As this decision criterion is not always suitable, we propose in this paper an algorithm for computing a policy optimal for the quantile criterion. Both finite and infinite horizons are considered. Finally we experimentally evaluate our approach on random MDPs and on a data center control problem.
更多查看译文
AI 理解论文
溯源树
样例
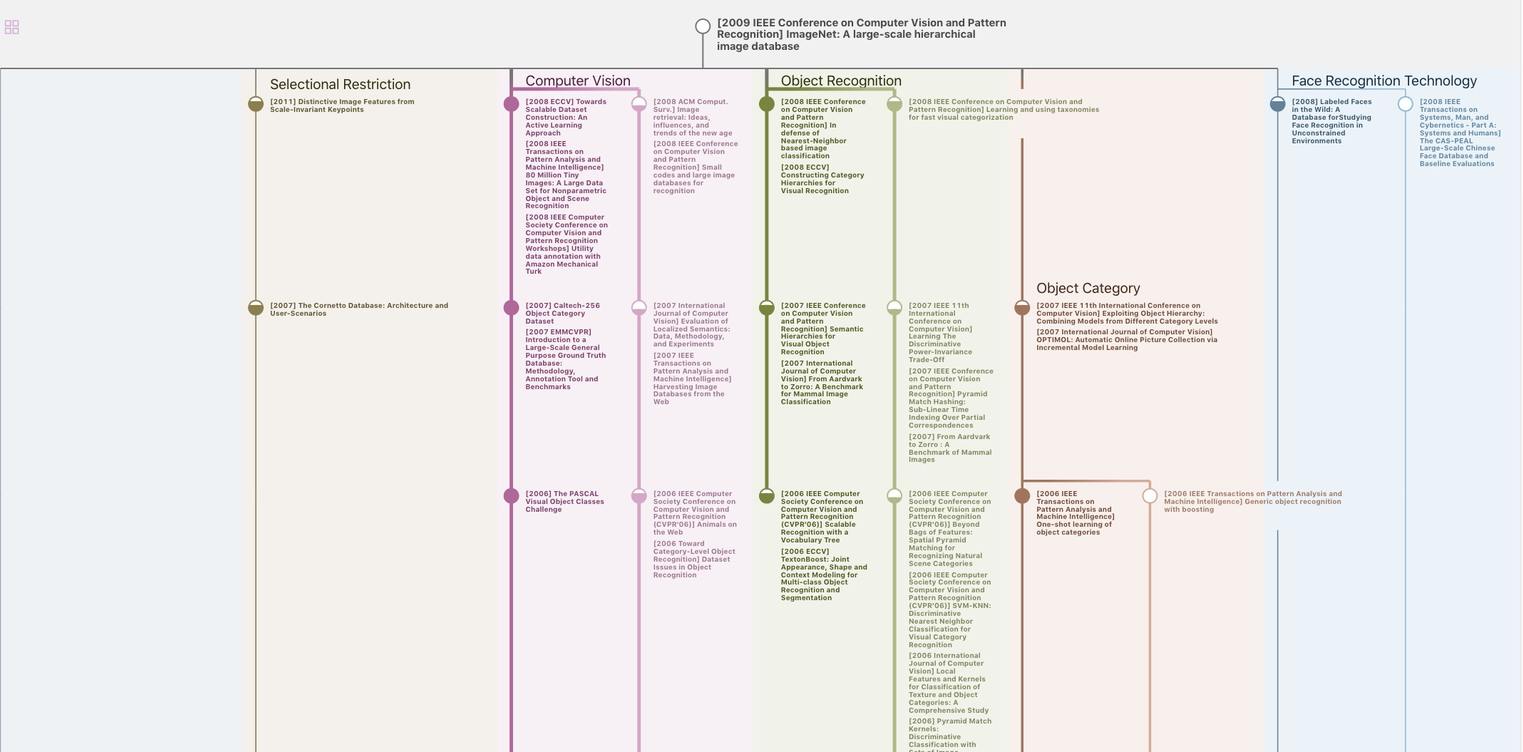
生成溯源树,研究论文发展脉络
Chat Paper
正在生成论文摘要