Question Retrieval for Community-based Question Answering via Heterogeneous Network Integration Learning
arXiv: Information Retrieval(2016)
摘要
Community based question answering platforms have attracted substantial users to share knowledge and learn from each other. As the rapid enlargement of CQA platforms, quantities of overlapped questions emerge, which makes users confounded to select a proper reference. It is urgent for us to take effective automated algorithms to reuse historical questions with corresponding answers. In this paper we focus on the problem with question retrieval, which aims to match historical questions that are relevant or semantically equivalent to resolve one s query directly. The challenges in this task are the lexical gaps between questions for the word ambiguity and word mismatch problem. Furthermore, limited words in queried sentences cause sparsity of word features. To alleviate these challenges, we propose a novel framework named HNIL which encodes not only the question contents but also the askers social interactions to enhance the question embedding performance. More specifically, we apply random walk based learning method with recurrent neural network to match the similarities between askers question and historical questions proposed by other users. Extensive experiments on a large scale dataset from a real world CQA site show that employing the heterogeneous social network information outperforms the other state of the art solutions in this task.
更多查看译文
AI 理解论文
溯源树
样例
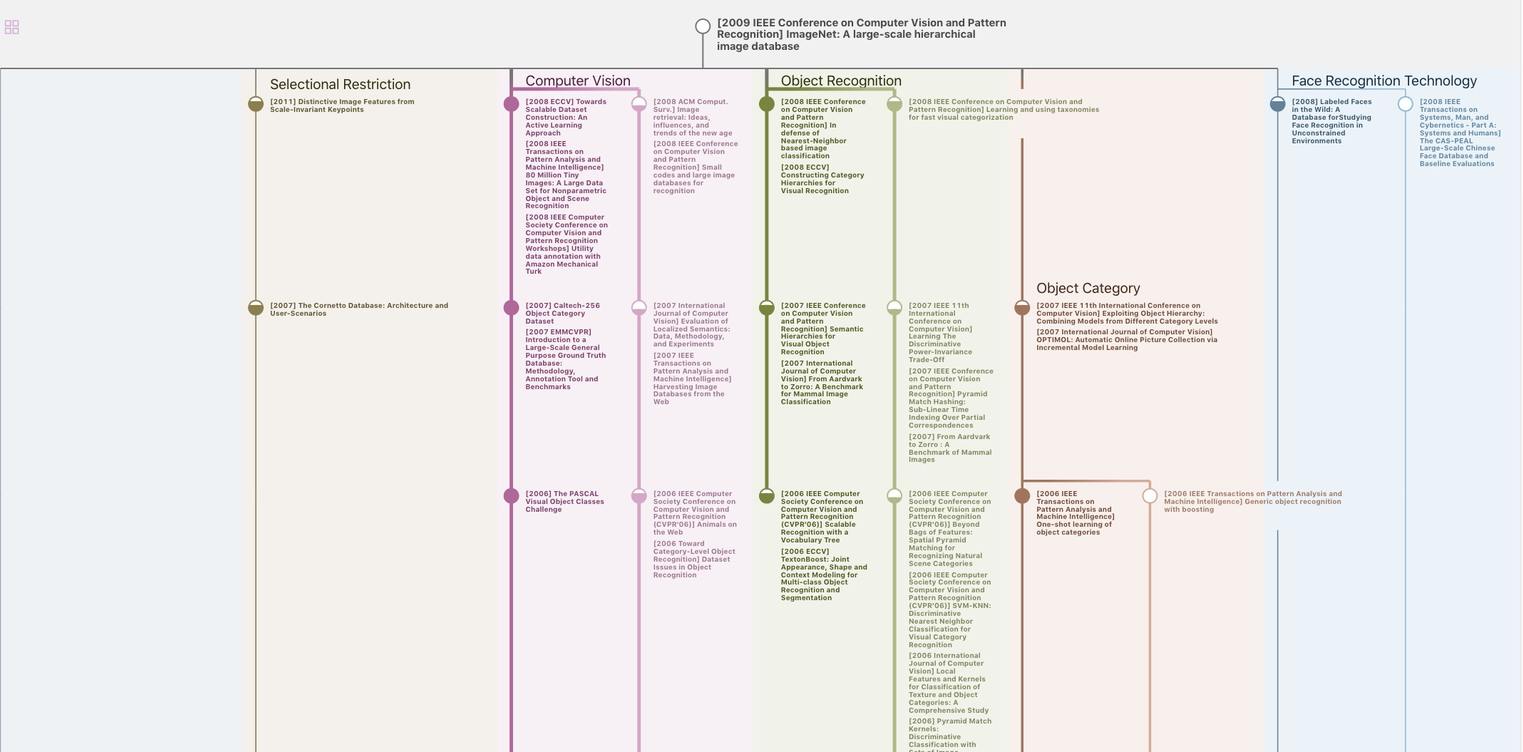
生成溯源树,研究论文发展脉络
Chat Paper
正在生成论文摘要