Low-Rank Matrix Recovery via Rank One Tight Frame Measurements
Journal of Fourier Analysis and Applications(2017)
摘要
The task of reconstructing a low rank matrix from incomplete linear measurements arises in areas such as machine learning, quantum state tomography and in the phase retrieval problem. In this note, we study the particular setup that the measurements are taken with respect to rank one matrices constructed from the elements of a random tight frame. We consider a convex optimization approach and show both robustness of the reconstruction with respect to noise on the measurements as well as stability with respect to passing to approximately low rank matrices. This is achieved by establishing a version of the null space property of the corresponding measurement map.
更多查看译文
关键词
Low rank matrix recovery,Quantum state tomography,Phase retrieval,Convex optimization,Nuclear norm minimization,Positive semidefinite least squares problem,Random measurements
AI 理解论文
溯源树
样例
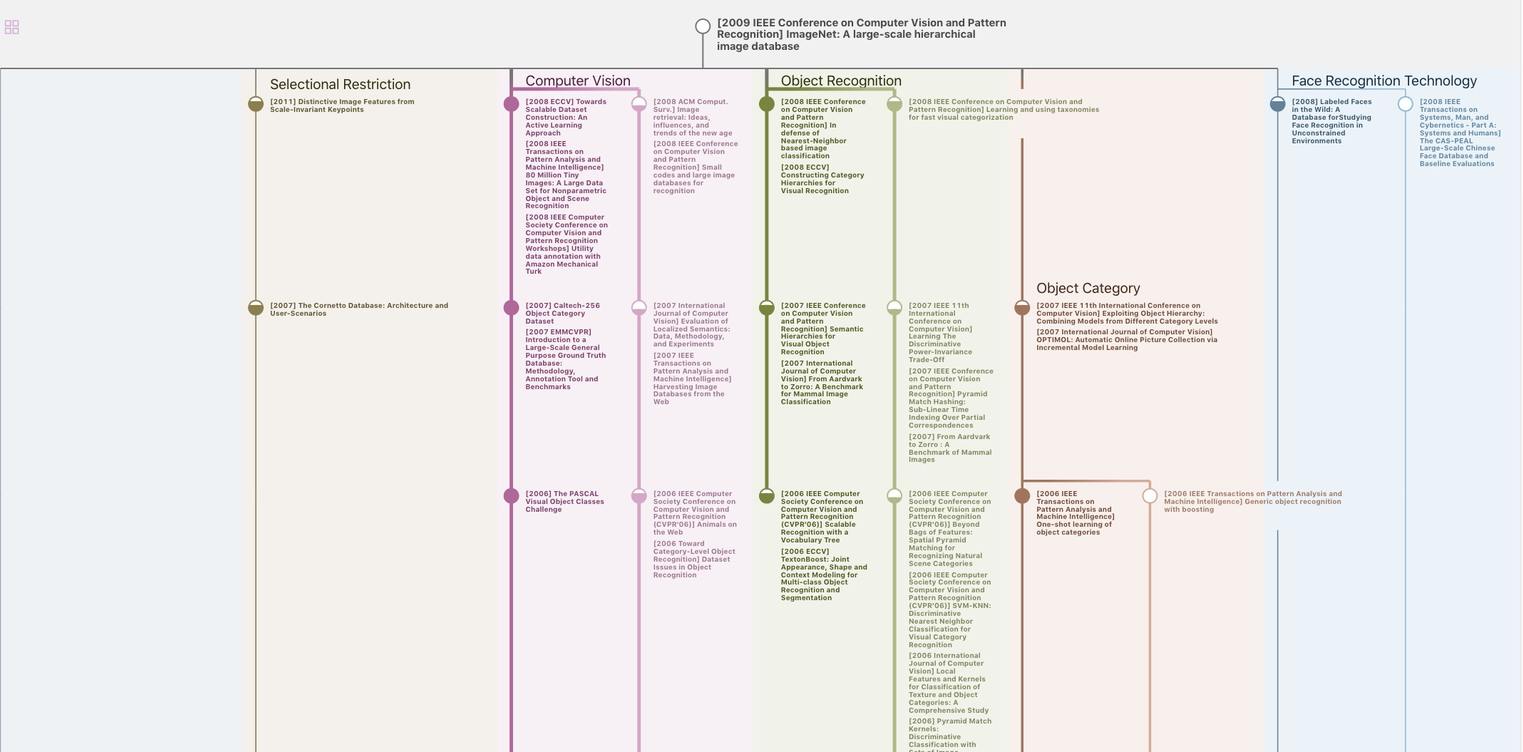
生成溯源树,研究论文发展脉络
Chat Paper
正在生成论文摘要