Model-Free Control of Thermostatically Controlled Loads Connected to a District Heating Network.
Energy and Buildings(2018)
摘要
Optimal control of thermostatically controlled loads connected to a district heating network is considered a sequential decision-making problem under uncertainty. The practicality of a direct model-based approach is compromised by two challenges, namely scalability due to the large dimensionality of the problem and the system identification required to identify an accurate model. To help in mitigating these problems, this paper leverages on recent developments in reinforcement learning in combination with a market-based multi-agent system to obtain a scalable solution that obtains a significant performance improvement in a practical learning time. In a first step, all relevant (and practically available) state information is collected from which a limited set of features is extracted, resulting in a low-dimensional representation of the system state. In a second step, a control action for the entire cluster is extracted from a policy determined offline. In a third and final step, this control action is dispatched over the different thermostatically controlled loads using a market-based multi-agent system. This process is repeated following a receding horizon approach. The control approach is applied to a scenario comprising 100 thermostatically controlled loads connected to a radial district heating network supplied by a central combined heat and power plant. Both for an energy arbitrage and a peak shaving objective, the control approach requires 60 days to obtain a performance within 65% of a theoretical lower bound on the cost.
更多查看译文
关键词
District heating,Combined heat and power,Reinforcement learning,Thermostatically controlled loads
AI 理解论文
溯源树
样例
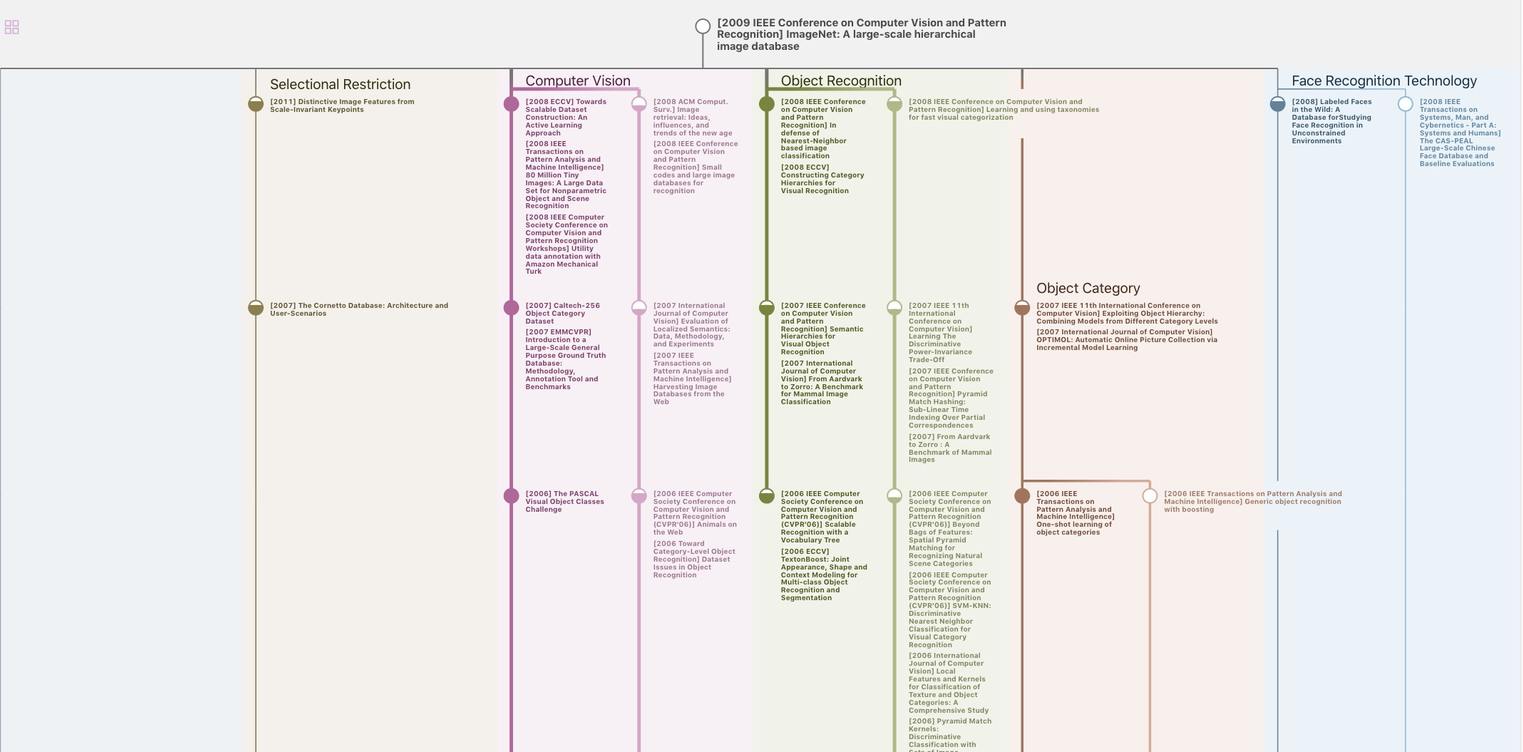
生成溯源树,研究论文发展脉络
Chat Paper
正在生成论文摘要