SSPP-DAN: Deep domain adaptation network for face recognition with single sample per person
2017 24TH IEEE INTERNATIONAL CONFERENCE ON IMAGE PROCESSING (ICIP)(2017)
摘要
Real-world face recognition using a single sample per person (SSPP) is a challenging task. The problem is exacerbated if the conditions under which the gallery image and the probe set are captured are completely different. To address these issues from the perspective of domain adaptation, we introduce an SSPP domain adaptation network (SSPP-DAN). In the proposed approach, domain adaptation, feature extraction, and classification are performed jointly using a deep architecture with domain-adversarial training. However, the SSPP characteristic of one training sample per class is insufficient to train the deep architecture. To overcome this shortage, we generate synthetic images with varying poses using a 3D face model. Experimental evaluations using a realistic SSPP dataset show that deep domain adaptation and image synthesis complement each other and dramatically improve accuracy. Experiments on a benchmark dataset using the proposed approach show state-of-the-art performance.
更多查看译文
关键词
SSPP face recognition, Domain adaptation, Image synthesis, SSPP-DAN, Surveillance camera
AI 理解论文
溯源树
样例
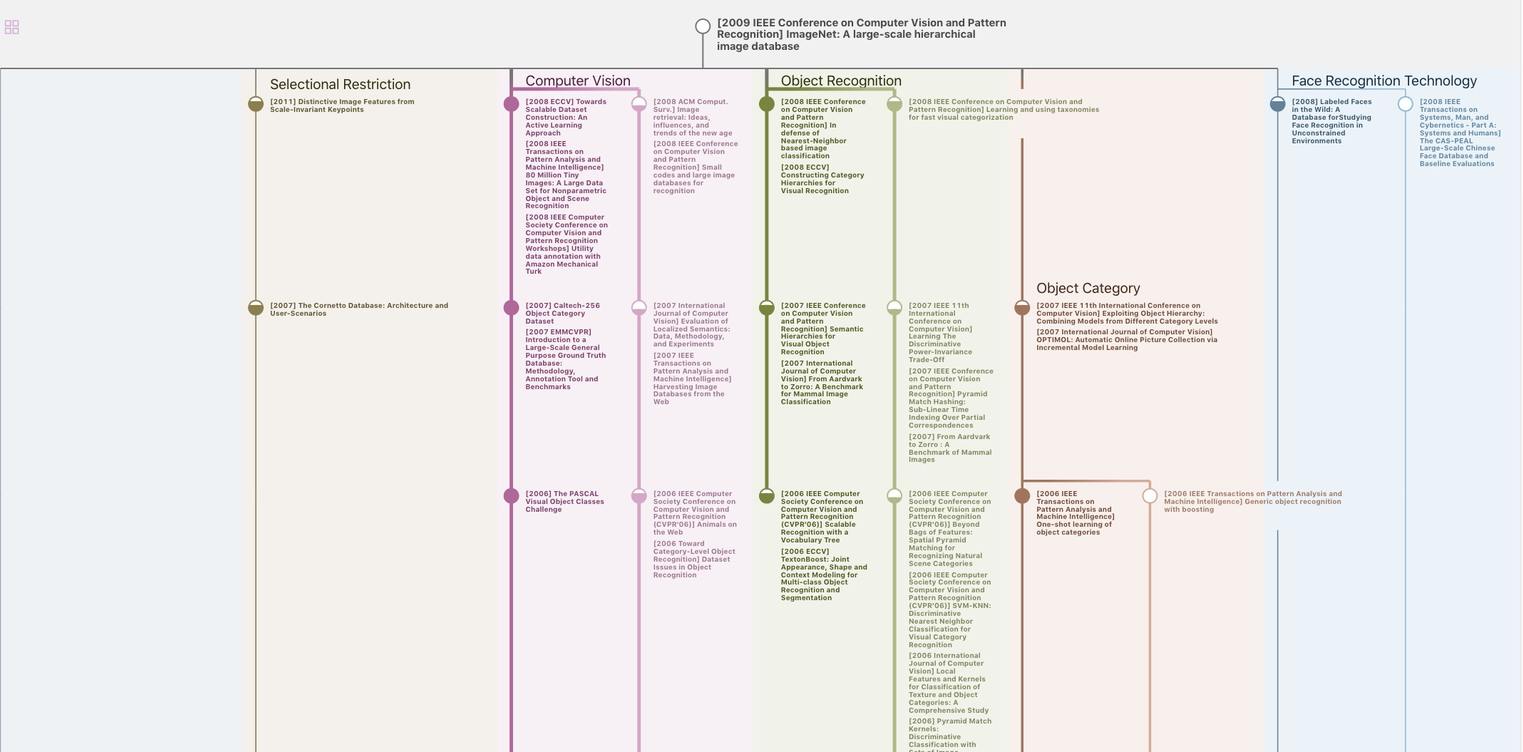
生成溯源树,研究论文发展脉络
Chat Paper
正在生成论文摘要