Strongly Adaptive Regret Implies Optimally Dynamic Regret.
arXiv: Learning(2017)
摘要
To cope with changing environments, recent developments in online learning have introduced the concepts of adaptive regret and dynamic regret independently. In this paper, we illustrate an intrinsic connection between these two concepts by showing that the dynamic regret can be expressed in terms of the adaptive regret and the functional variation. This observation implies that strongly adaptive algorithms can be directly leveraged to minimize the dynamic regret. As a result, we present a series of strongly adaptive algorithms whose dynamic regrets are minimax optimal for convex functions, exponentially concave functions, and strongly convex functions, respectively. To the best of our knowledge, this is the first time that such kind of dynamic regret bound is established for exponentially concave functions. Moreover, all of those adaptive algorithms do not need any prior knowledge of the functional variation, which is a significant advantage over previous specialized methods for minimizing dynamic regret.
更多查看译文
关键词
adaptive regret,dynamic regret,optimally
AI 理解论文
溯源树
样例
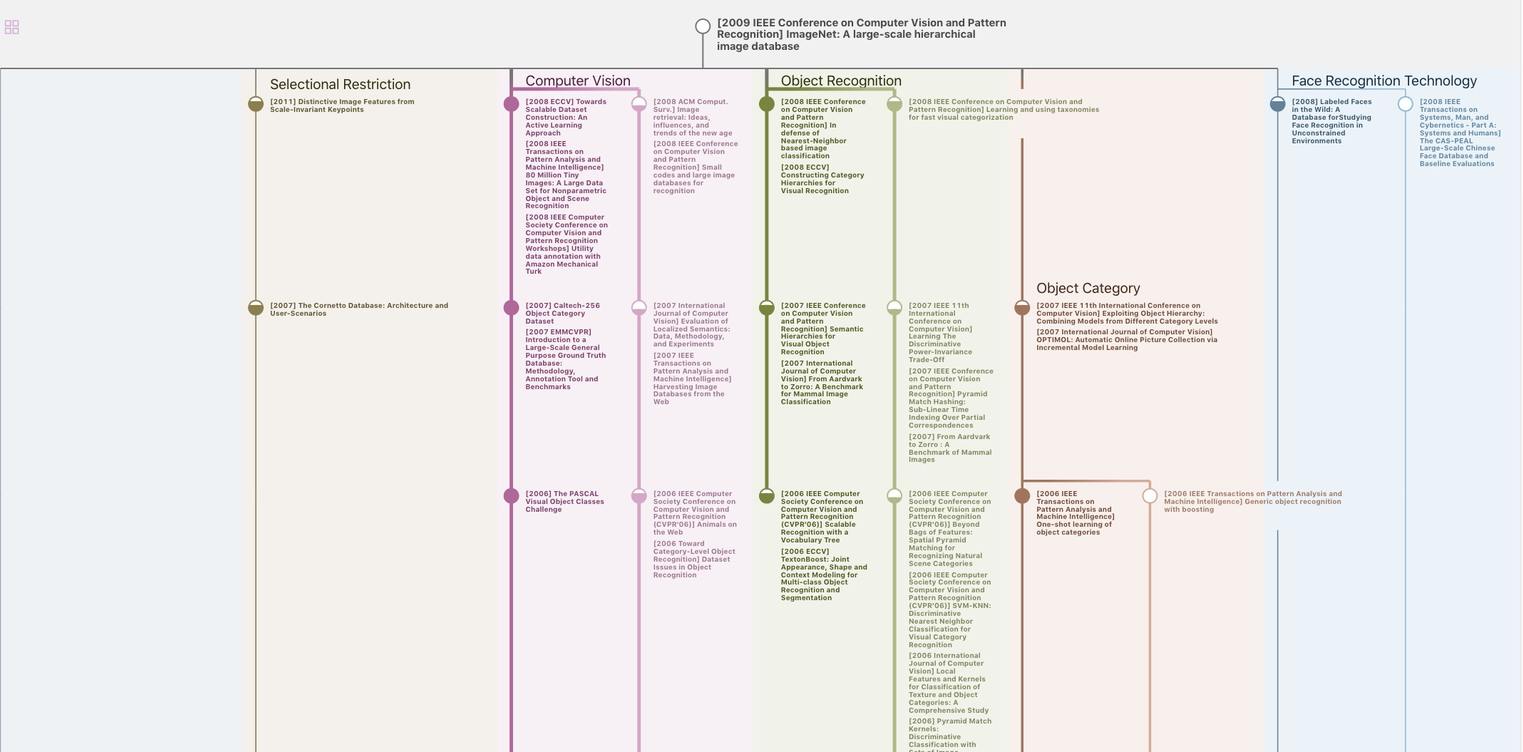
生成溯源树,研究论文发展脉络
Chat Paper
正在生成论文摘要