KEPLER: Keypoint and Pose Estimation of Unconstrained Faces by Learning Efficient H-CNN Regressors
2017 12th IEEE International Conference on Automatic Face & Gesture Recognition (FG 2017)(2017)
摘要
Keypoint detection is one of the most important pre-processing steps in tasks such as face modeling, recognition and verification. In this paper, we present an iterative method for Keypoint Estimation and Pose prediction of unconstrained faces by Learning Efficient H-CNN Regressors (KEPLER) for addressing the face alignment problem. Recent state of the art methods have shown improvements in face keypoint detection by employing Convolution Neural Networks (CNNs). Although a simple feed forward neural network can learn the mapping between input and output spaces, it cannot learn the inherent structural dependencies. We present a novel architecture called H-CNN (Heatmap-CNN) which captures structured global and local features and thus favors accurate keypoint detection. H-CNN is jointly trained on the visibility, fiducials and 3D-pose of the face. As the iterations proceed, the error decreases making the gradients small and thus requiring efficient training of DCNNs to mitigate this. KEPLER performs global corrections in pose and fiducials for the first four iterations followed by local corrections in a subsequent stage. As a by-product, KEPLER also provides 3D pose (pitch, yaw and roll) of the face accurately. In this paper, we show that without using any 3D information, KEPLER outperforms state of the art methods for alignment on challenging datasets such as AFW [38] and AFLW [17].
更多查看译文
关键词
3D information,3D pose,fiducials,DCNN,3D-pose,Heatmap-CNN,feed forward neural network,Convolution Neural Networks,face keypoint detection,face alignment problem,pose prediction,keypoint estimation,iterative method,recognition,face modeling,H-CNN regressors,learning,unconstrained faces,pose estimation,KEPLER
AI 理解论文
溯源树
样例
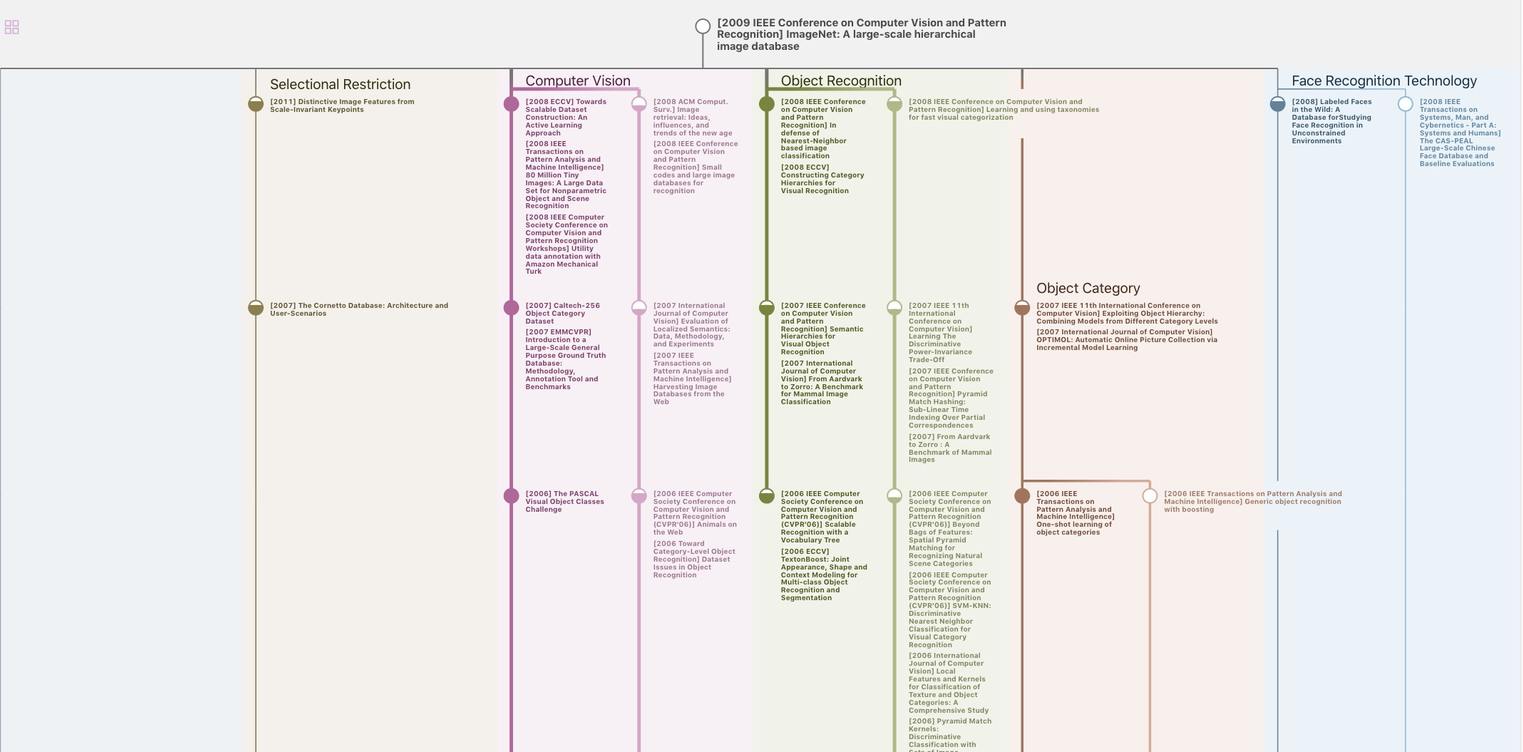
生成溯源树,研究论文发展脉络
Chat Paper
正在生成论文摘要