User-Centric Similarity Search.
IEEE Trans. Knowl. Data Eng.(2017)
摘要
User preferences play a significant role in market analysis. In the database literature, there has been extensive work on query primitives, such as the well known top-$k$ query that can be used for the ranking of products based on the preferences customers have expressed. Still, the fundamental operation that evaluates the similarity between products is typically done ignoring these preferences. Instead products are depicted in a feature space based on their attributes and similarity is computed via traditional distance metrics on that space. In this work, we utilize the rankings of the products based on the opinions of their customers in order to map the products in a user-centric space where similarity calculations are performed. We identify important properties of this mapping that result in upper and lower similarity bounds, which in turn permit us to utilize conventional multidimensional indexes on the original product space in order to perform these user-centric similarity computations. We show how interesting similarity calculations that are motivated by the commonly used range and nearest neighbor queries can be performed efficiently, while pruning significant parts of the data set based on the bounds we derive on the user-centric similarity of products.
更多查看译文
关键词
Business,Indexes,Electronic mail,Extraterrestrial measurements,Nearest neighbor searches,Euclidean distance
AI 理解论文
溯源树
样例
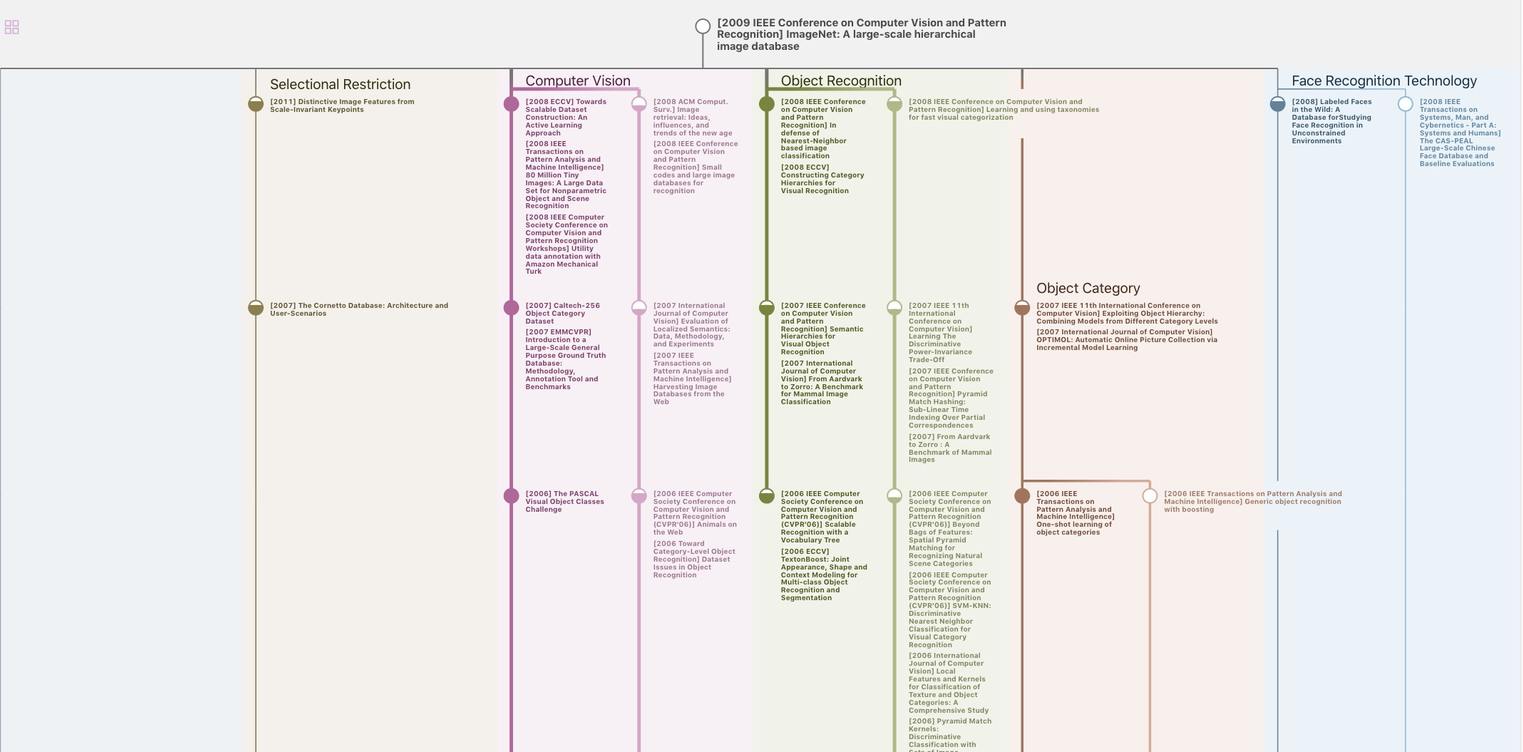
生成溯源树,研究论文发展脉络
Chat Paper
正在生成论文摘要