Online growing neural gas for anomaly detection in changing surveillance scenes
Pattern Recognition(2017)
摘要
Anomaly detection is still a challenging task for video surveillance due to complex environments and unpredictable human behaviors. Most existing approaches train offline detectors using manually labeled data and predefined parameters, and are hard to model changing scenes. This paper introduces a neural network based model called online Growing Neural Gas (online GNG) to perform an unsupervised learning. Unlike a parameter-fixed GNG, our model updates learning parameters continuously, for which we propose several online neighbor-related strategies. Specific operations, namely neuron insertion, deletion, learning rate adaptation and stopping criteria selection, get upgraded to online modes. In the anomaly detection stage, the behavior patterns far away from our model are labeled as anomalous, for which far away is measured by a time-varying threshold. Experiments are implemented on three surveillance datasets, namely UMN, UCSD Ped1/Ped2 and Avenue dataset. All datasets have changing scenes due to mutable crowd density and behavior types. Anomaly detection results show that our model can adapt to the current scene rapidly and reduce false alarms while still detecting most anomalies. Quantitative comparisons with 12 recent approaches further confirm our superiority. © 2016 Elsevier Ltd
更多查看译文
关键词
Anomaly detection,Unsupervised learning,Video surveillance
AI 理解论文
溯源树
样例
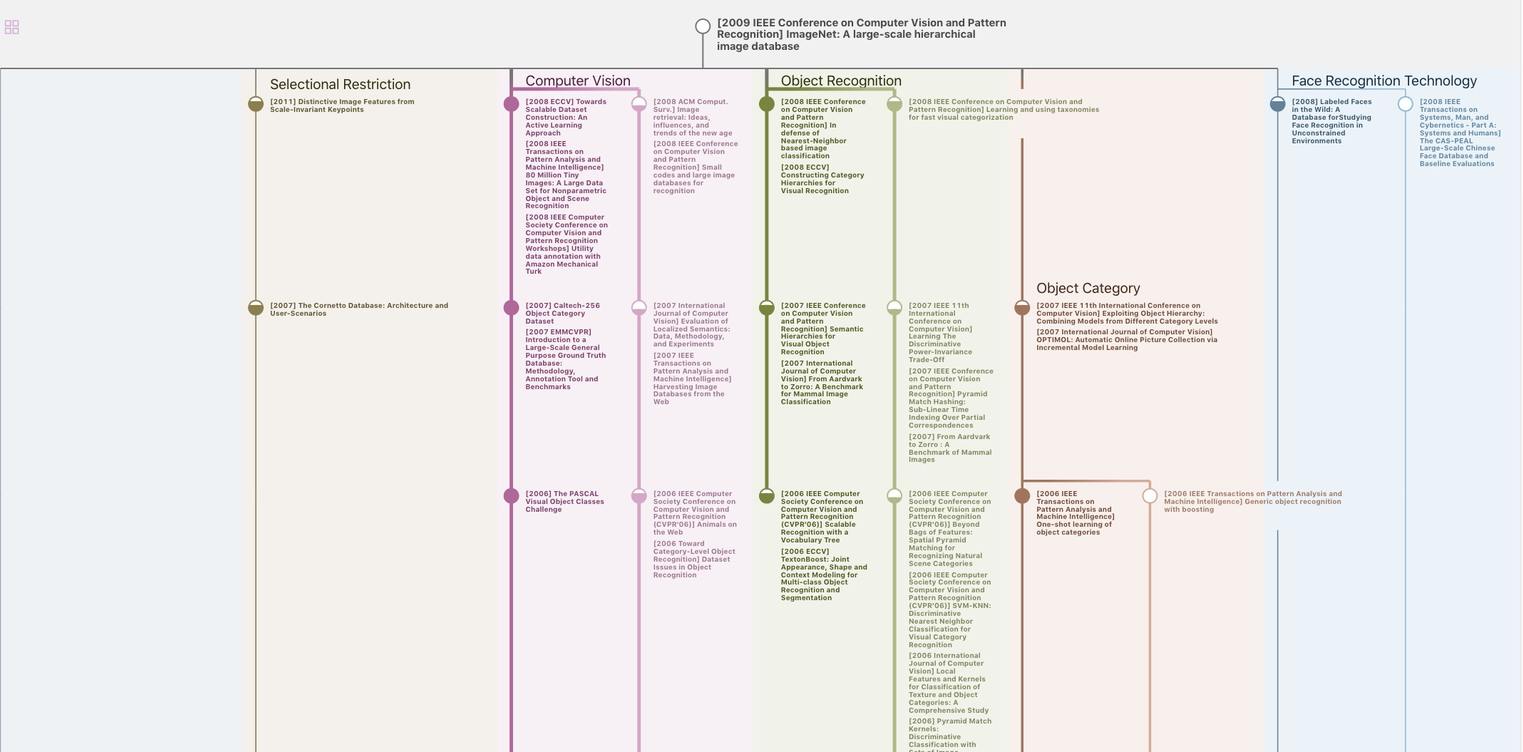
生成溯源树,研究论文发展脉络
Chat Paper
正在生成论文摘要