Predicting the Bursts of Data Access Streams by Filtering Correlated I/Os
2016 IEEE 18th International Conference on High Performance Computing and Communications; IEEE 14th International Conference on Smart City; IEEE 2nd International Conference on Data Science and Systems (HPCC/SmartCity/DSS)(2016)
摘要
Bursty behavior normally indicates that the workload generated by data accesses happens in short time, uneven spurts. In order to handle the bursts, the physical resources of IT devices have to be configured to offer capability which goes far beyond the average resource utilization, thus satisfying the performance. However, this kind of fat provisioning incurs wasting resources when the system does not experience peak workloads. If the bursts can be predicted in advance, thin provision will save a lot of resources in contrast to the fat provision. However, the bursty data access involves both correlated I/Os and non-correlated I/Os which are mixed together. Therefore, it has long been a challenge to effectively predict the bursts. By analyzing real traces, this paper observes that the non-correlated block I/Os dominate bursts across I/O workloads. Based on this observation, SAW-Apriori algorithm is proposed in this paper to mine the frequent and correlated I/Os by enhancing the temporal locality of traditionl Apriori algorithm. Furthermore, this paper proposes to predict the bursts by filtering those frequent and correlated I/Os. Experimental results demonstrate that the proposed approach significantly outperforms the traditional time series method when predicting the bursts.
更多查看译文
关键词
Storage I/O,bursty access,association mining,strengthened association window,prediction
AI 理解论文
溯源树
样例
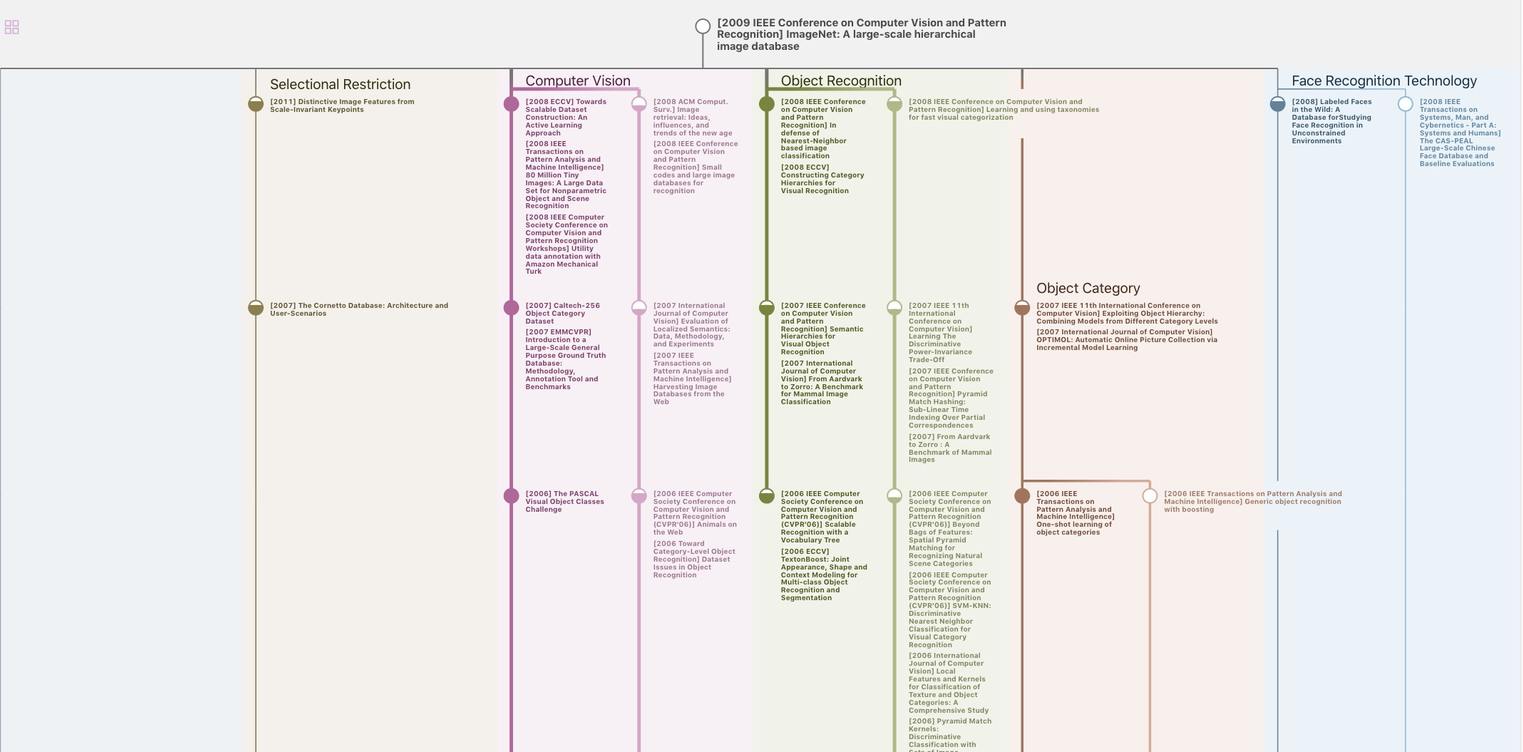
生成溯源树,研究论文发展脉络
Chat Paper
正在生成论文摘要