Discriminative Capacity and Phonetic Information of Bottleneck Features in Speech
PROGRESS IN PATTERN RECOGNITION, IMAGE ANALYSIS, COMPUTER VISION, AND APPLICATIONS, CIARP 2016(2017)
摘要
The impressive gain in performance obtained using deep neural network (DNN) for automatic speech recognition have motivated their application to other speech related tasks such as speaker recognition and language recognition, but there is still uncertainty about what is deep training strategy extracting, from the acoustic data, to make it such a powerful learning tool. This paper compares the discriminative capacity and the phonetic information conveyed by the feature-space maximum likelihood linear regression (fMLLR) before and after passing through a DNN trained to discriminate between tri-phone tied states. The proposed experimentation reflected the superiority of DNN bottleneck features regarding its information content.
更多查看译文
关键词
Entropy, Phonetic information, Bottleneck features, Deep neural network
AI 理解论文
溯源树
样例
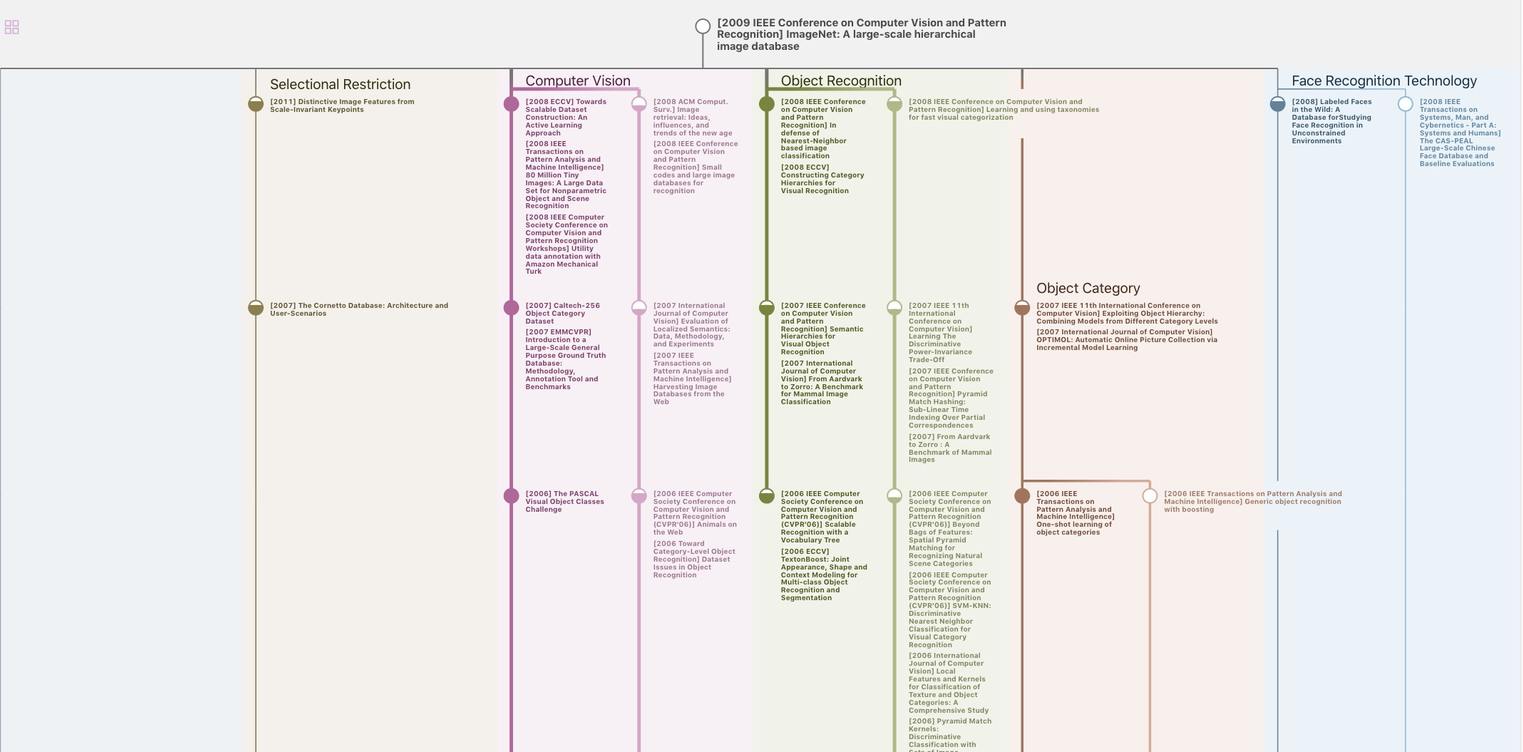
生成溯源树,研究论文发展脉络
Chat Paper
正在生成论文摘要