Efficient Training Over Long Short-Term Memory Networks for Wind Speed Forecasting
PROGRESS IN PATTERN RECOGNITION, IMAGE ANALYSIS, COMPUTER VISION, AND APPLICATIONS, CIARP 2016(2017)
摘要
Due to its variability, the development of wind power entails several difficulties, including wind speed forecasting. The Long Short-Term Memory (LSTM) is a particular type of recurrent network that can be used to work with sequential data, and previous works showed good empirical results. However, its training algorithm is expensive in terms of computation time. This paper proposes an efficient algorithm to train LSTM, decreasing computation time while maintaining good performance. The proposal is organized in two stages: (i) first to improve the weights output layer; (ii) next, update all weights using the original algorithm with one epoch. We used the proposed method to forecast wind speeds from 1 to 24 h ahead. Results demonstrated that our algorithm outperforms the original training algorithm, improving the efficiency and achieving better or comparable performance in terms of MAPE, MAE and RMSE.
更多查看译文
关键词
Wind speed forecasting, Recurrent neural networks, Long Short-Term Memory, Multivariate time series
AI 理解论文
溯源树
样例
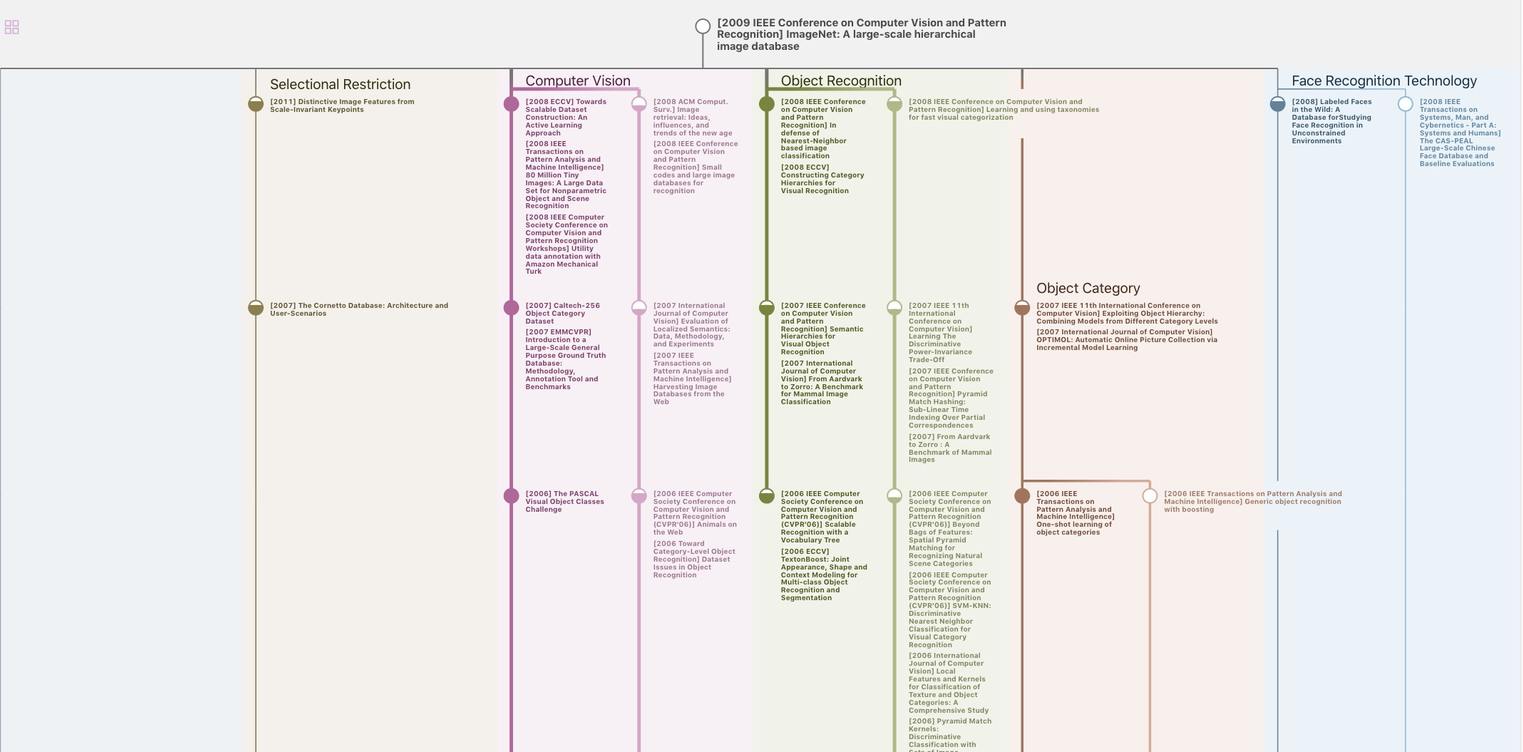
生成溯源树,研究论文发展脉络
Chat Paper
正在生成论文摘要