A study of vehicle detector generalization on U.S. highway
2016 IEEE 19th International Conference on Intelligent Transportation Systems (ITSC)(2016)
摘要
Vehicle detection is an essential task in an intelligent vehicle. Despite being a well-studied vision problem, it is unclear how well vehicle detectors generalize to new settings. Specifically, this paper studies the generalization capability of vehicle detectors on a U.S. highway dataset. Two types of models are employed in the experimental analysis, a subcategory aggregate channel features model and a region-based convolutional neural network model. The experiments demonstrate limited generalization capability of pre-trained models when evaluated on a dataset captured in new settings. This observation motivates technical modifications in order to improve generalization to the new dataset. By exploring novel training techniques, we significantly improve detection performance by up to 10%, demonstrating the importance of studying cross-dataset generalization.
更多查看译文
关键词
vehicle detector generalization,US highway,intelligent vehicle,vision problem,region-based convolutional neural network model,subcategory aggregate channel features model,training techniques,cross-dataset generalization,generalization capability
AI 理解论文
溯源树
样例
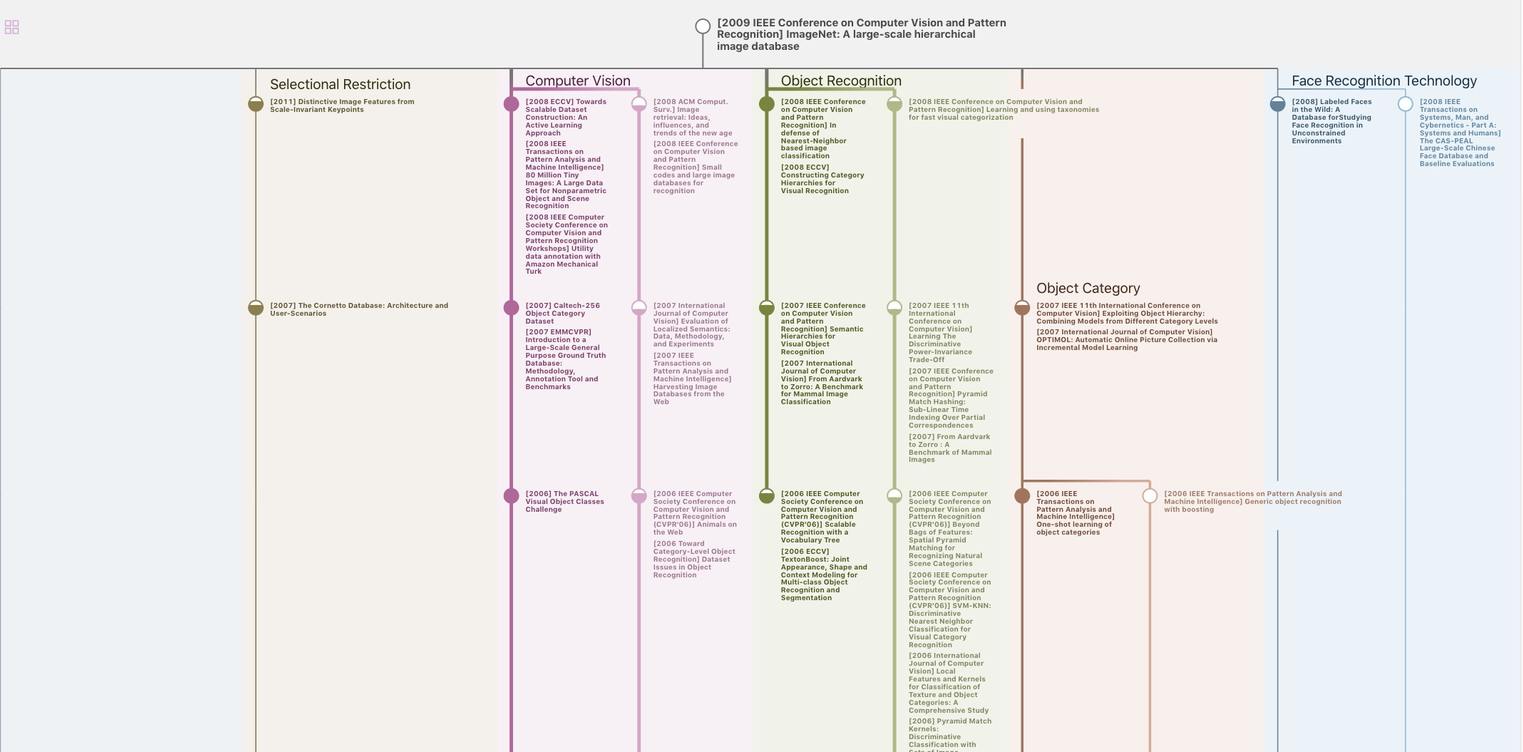
生成溯源树,研究论文发展脉络
Chat Paper
正在生成论文摘要