A light-weight yet accurate localization system for autonomous cars in large-scale and complex environments
2016 IEEE 19th International Conference on Intelligent Transportation Systems (ITSC)(2016)
摘要
We propose a light-weight yet accurate localization system for autonomous cars that operate in large-scale and complex urban environments. It provides appropriate localization accuracy and processing time at high frequency suitable for fast control actions, besides low power consumption desirable for limited energy availability in commercial cars. The localization system is based on the Particle Filter (PF) localization, which corrects particles' poses by applying map-matching between 2D global occupancy grid-maps computed offline and 2D local occupancy grid-maps constructed online. The localization system converts the dense 3D point clouds of a 3D LiDAR into sparse local maps, which allowed the reduction of the localization processing time without deteriorating its accuracy. We propose two map-matching distance functions: one is an improvement on the traditional Likelihood Field distance between two grid-maps and the other is an adaptation of the standard Cosine distance between two high-dimensional vectors, commonly used in the Information Retrieval research area. To collect data for evaluating the localization system, we used an in-house autonomous car. Experimental results showed that the localization system was able to operate at about 300Hz and 100Hz for the map-matching Likelihood Field distance and the Cosine distance functions, respectively, using a population of 500 particles.
更多查看译文
关键词
lightweight localization system,autonomous cars,complex urban environments,particle filter localization,2D global occupancy grid-maps,2D local occupancy grid-maps,3D LiDAR dense 3D point clouds,sparse local maps,map-matching distance functions,high-dimensional vectors,map-matching likelihood field distance,cosine distance functions,frequency 300 Hz,frequency 100 Hz
AI 理解论文
溯源树
样例
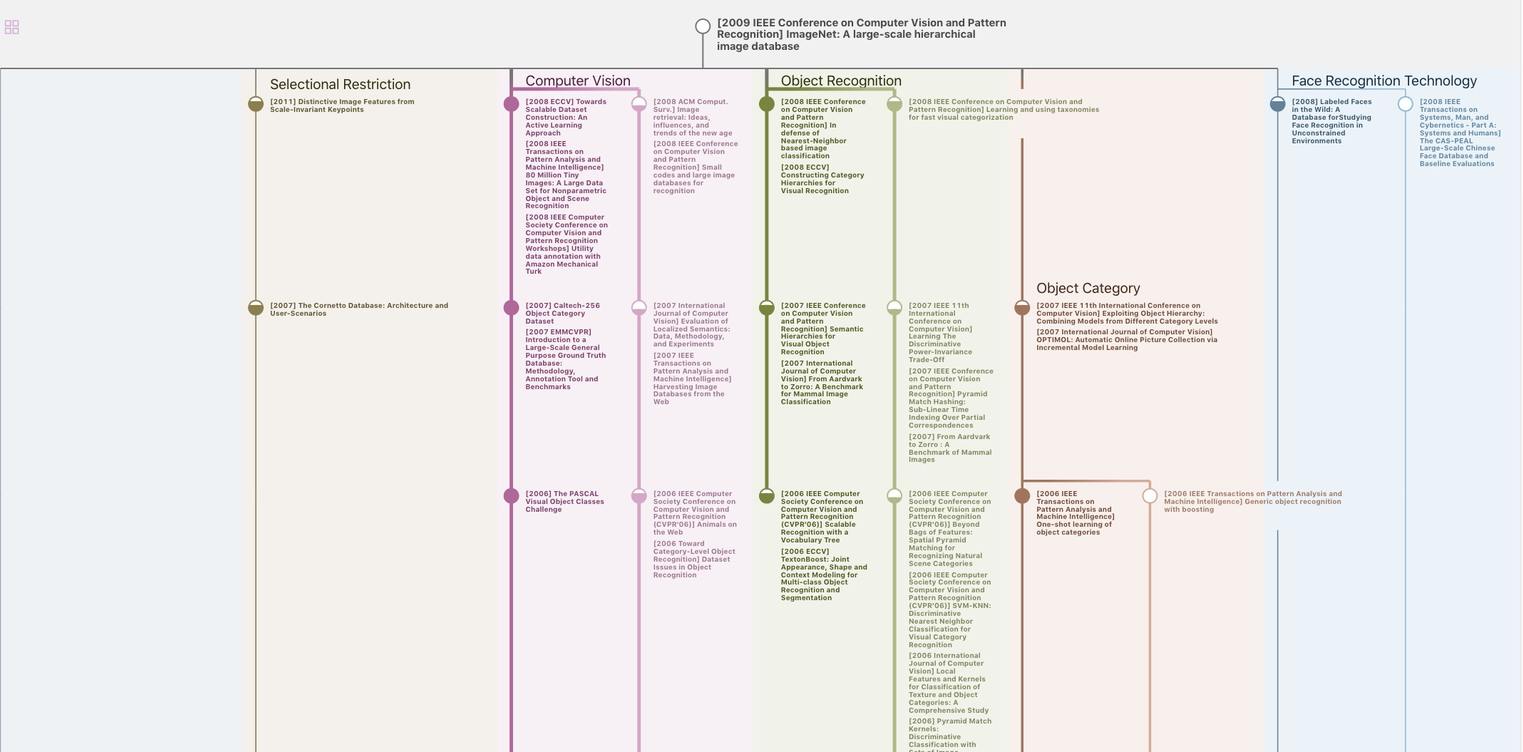
生成溯源树,研究论文发展脉络
Chat Paper
正在生成论文摘要