Predicting The Duration Of Non-Recurring Road Incidents By Cluster-Specific Models
2016 IEEE 19th International Conference on Intelligent Transportation Systems (ITSC)(2016)
摘要
In metropolitan areas, about 50% of traffic delays are caused by non-recurring traffic incidents. Hence, accurate prediction of the duration of such events is critical for traffic management authorities. In this paper, we study the predictability of the duration of traffic incidents by considering various external factors. As incident data is typically sparse, training a large number of models (for instance, model for each road) is not possible. On the other hand, training one model for the entire network may not be a suitable solution, as such a model will be too generalized and consequently unsuitable for many relatively rare scenarios. Therefore, we propose to solve this issue by first grouping incidents through common latent similarities among them and then training data-driven predictors for each group. In our numerical analysis we consider incident data from Singapore and the Netherlands. Our results show that by training cluster-specific models we can reduce the prediction error by 19.41% for incidents in Singapore and by 17.8% for incidents in the Netherlands.
更多查看译文
关键词
nonrecurring road incidents,cluster-specific models,metropolitan areas,nonrecurring traffic incidents,traffic management authorities,common latent similarities,data-driven predictors,Singapore,Netherlands
AI 理解论文
溯源树
样例
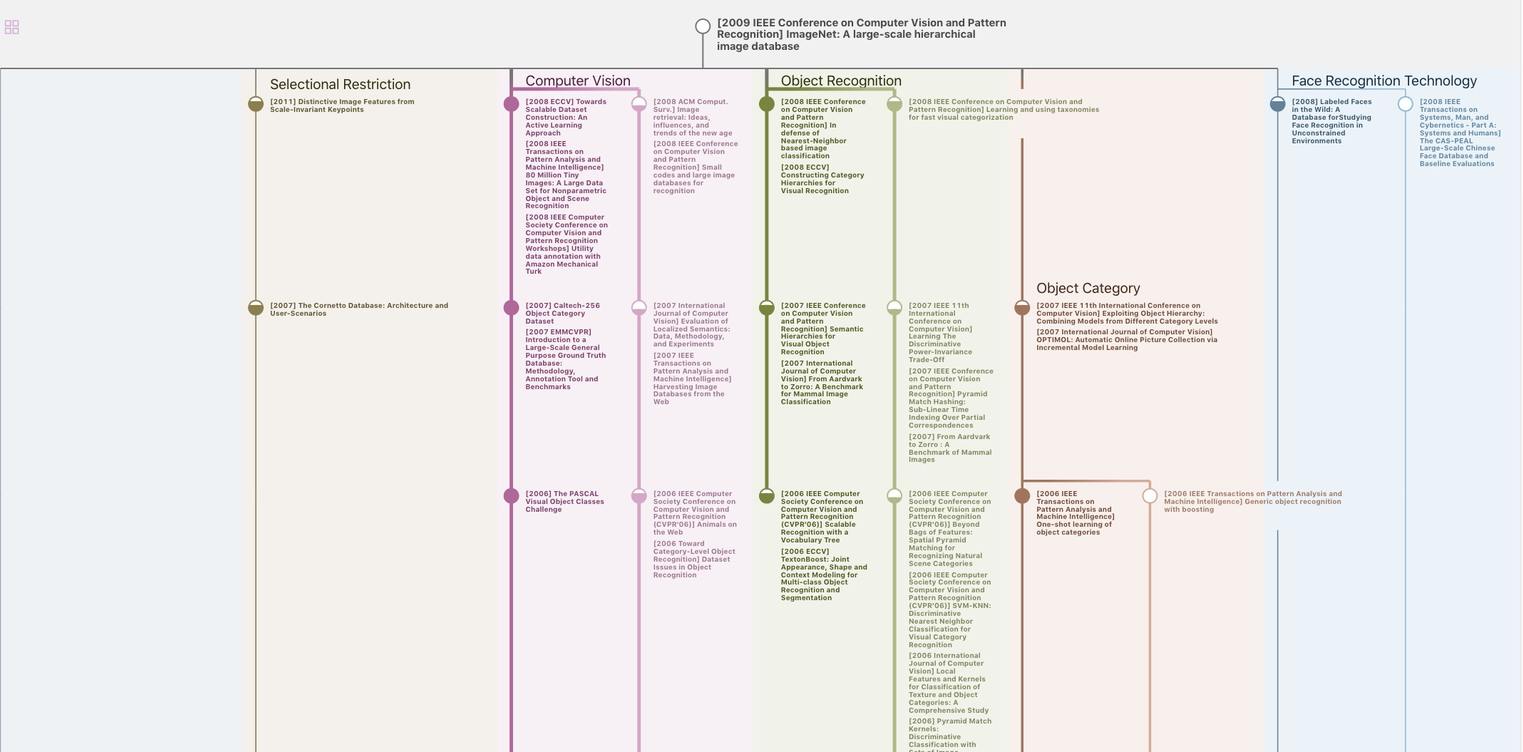
生成溯源树,研究论文发展脉络
Chat Paper
正在生成论文摘要